CGAN-based Synthetic Medical Image Augmentation between Retinal Fundus Images and Vessel Segmented Images
2020 5th International Conference on Control and Robotics Engineering (ICCRE)(2020)
摘要
Computer-aided medical diagnosis is widely used to assist in the interpretation of medical images. Early screening of diseases has a great value for the clinical diagnosis. However, medical images are scarce and fraught with legal concerns about patient privacy. Inspired by the prevailing learning capability of Generative Adversarial Network (GAN), We propose simulated learning: it first learns a mapping from retinal fundus images to vessel segmented images and then trains a learning model to generate synthetic fundus images. In the results, we evaluated our proposed method from both quantitative and qualitative aspects. For generated segmented images, our method achieves a dice coefficient of 0.838 and an AUC of 0.985 on the DRIVE datasets. You can also see more information about our medical image algorithm results at https://github.com/qiqi7788/Bidirectional-Mapping-formedical-image. These results also verify the effectiveness of our algorithm.
更多查看译文
关键词
Generative Adversarial Network (GAN),Dice coefficient,AUC
AI 理解论文
溯源树
样例
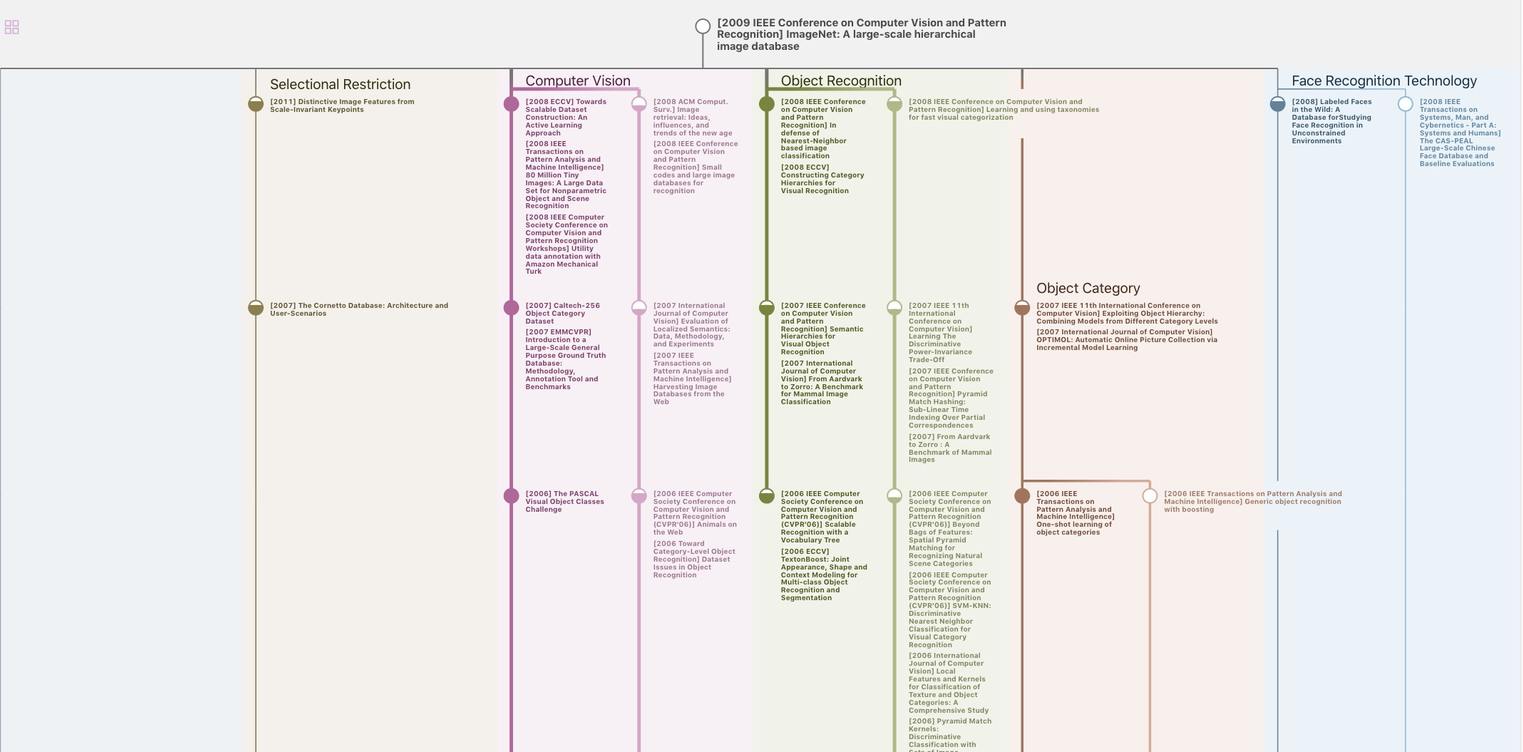
生成溯源树,研究论文发展脉络
Chat Paper
正在生成论文摘要