Causal deep learning reveals the comparative effectiveness of antihyperglycemic treatments in poorly controlled diabetes
NATURE COMMUNICATIONS(2022)
摘要
Type-2 diabetes is associated with severe health outcomes, the effects of which are responsible for approximately 1/4 th of the total healthcare spending in the United States (US). Current treatment guidelines endorse a massive number of potential anti-hyperglycemic treatment options in various combinations. Strategies for optimizing treatment selection are lacking. Real-world data from a nationwide population of over one million high-risk diabetic patients (HbA1c ≥ 9%) in the US is analyzed to evaluate the comparative effectiveness for HbA1c reduction in this population of more than 80 different treatment strategies ranging from monotherapy up to combinations of five concomitant classes of drugs across each of 10 clinical cohorts defined by age, insulin dependence, and a number of other chronic conditions. A causal deep learning approach developed on such data allows for more personalized evaluation of treatment selection. An average confounder-adjusted reduction in HbA1c of 0.69% [−0.75, −0.65] is observed between patients receiving high vs low ranked treatments across cohorts for which the difference was significant. This method can be extended to explore treatment optimization for other chronic conditions.
更多查看译文
关键词
causal deep learning,diabetes,antihyperglycemic treatments,deep learning
AI 理解论文
溯源树
样例
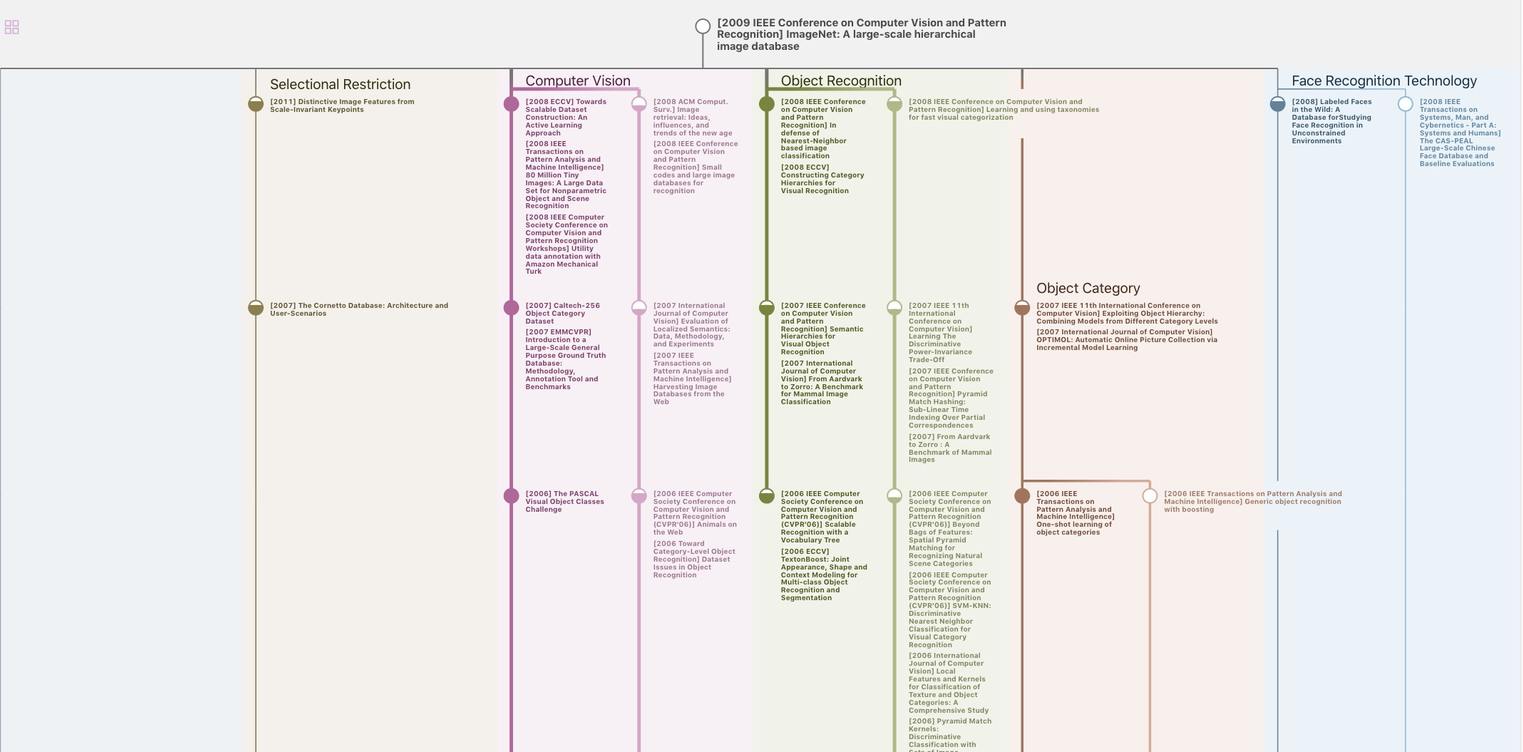
生成溯源树,研究论文发展脉络
Chat Paper
正在生成论文摘要