Residual Autoencoder Deep Neural Network for Electrical Capacitance Tomography
CMC-COMPUTERS MATERIALS & CONTINUA(2022)
摘要
Great achievements have been made during the last decades in the field of Electrical Capacitance Tomography (ECT) image reconstruction. However, there is still a need to make these image reconstruction results faster and of better quality. Recently, Deep Learning (DL) is flourishing and is adopted in many fields. The DL is very good at dealing with complex nonlinear functions and it is built using several series of Artificial Neural Networks (ANNs). An ECT image reconstruction model using DNN is proposed in this paper. The proposed model mainly uses Residual Autoencoder called (ECT_ResAE). Alarge-scale dataset of 320 k instances have been generated to train and test the proposed ECT_ResAE model. Each instance contains two vectors; a distinct permittivity distribution and its corresponding capacitance measurements. The capacitance vector has been modulated to generate a 66x66 image, and represented to the ECT_ResAE as an input. The scalability and practicability of the ECT_ResAE network are tested using noisy data, new samples, and experimental data. The experimental results show that the proposed ECT_ResAE image reconstruction model provides accurate reconstructed images. It achieved an average image Correlation Coefficient (CC) of more than 99% and an averageRelative ImageError (IE) around 8.5%.
更多查看译文
关键词
ECT, image reconstruction, deep learning, residual, autoencoder, IE, CC
AI 理解论文
溯源树
样例
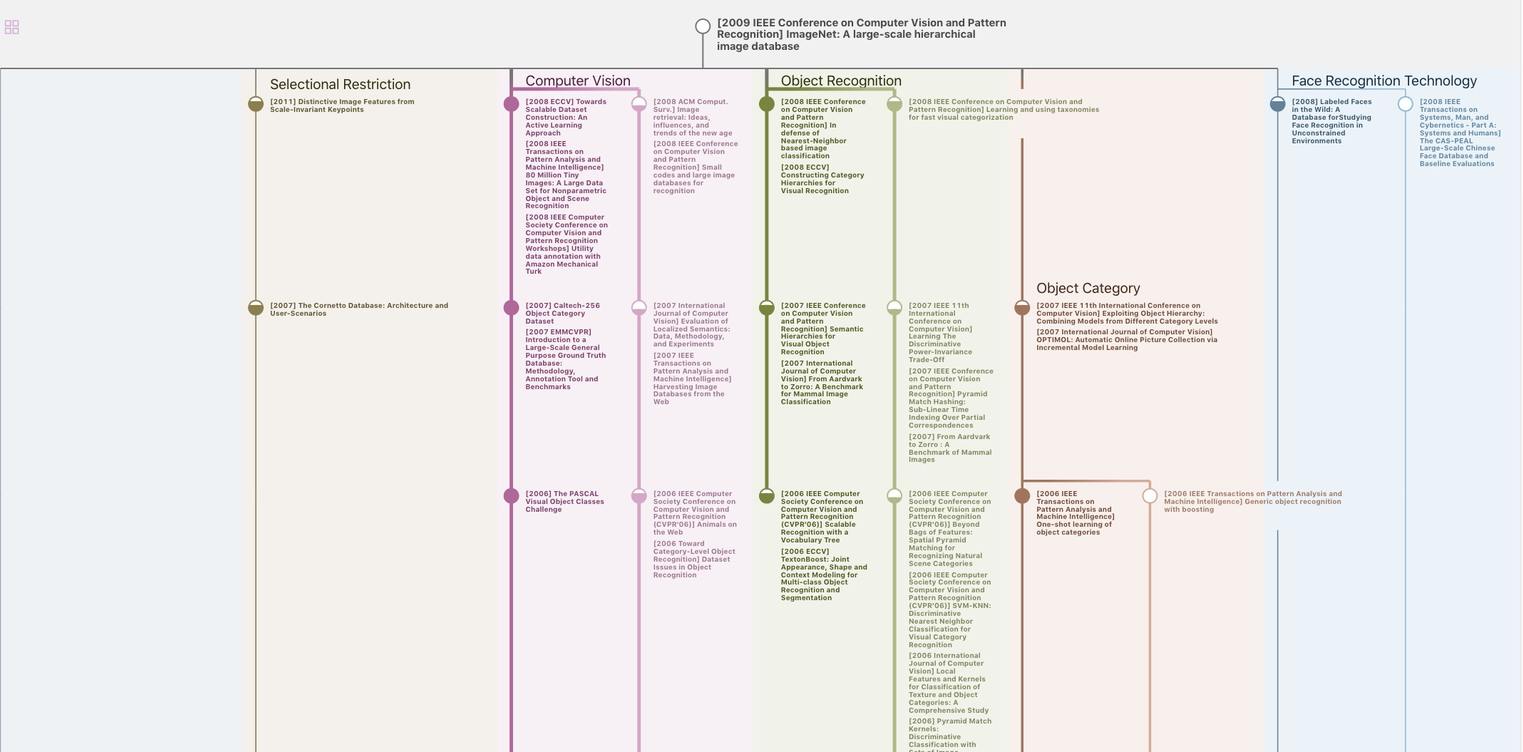
生成溯源树,研究论文发展脉络
Chat Paper
正在生成论文摘要