Robust Colorectal Polyp Characterization Using a Hybrid Bayesian Neural Network
CANCER PREVENTION THROUGH EARLY DETECTION, CAPTION 2022(2022)
摘要
Computer-Aided Diagnosis (CADx) systems can play a crucial role as a second opinion for endoscopists to improve the overall optical diagnostic performance of colonoscopies. While such supportive systems hold great potential, optimal clinical implementation is currently impeded, since deep neural network-based systems often tend to overestimate the confidence about their decisions. In other words, these systems are poorly calibrated, and, hence, may assign high prediction scores to samples associated with incorrect model predictions. For the optimal clinical workflow integration and physician-AI collaboration, a reliable CADx system should provide accurate and well-calibrated classification confidence. An important application of these models is characterization of Colorectal polyps (CRPs), that are potential precursor lesions of Colorectal cancer (CRC). An improved optical diagnosis of CRPs during the colonoscopy procedure is essential for an appropriate treatment strategy. In this paper, we incorporate Bayesian variational inference and investigate the performance of a hybrid Bayesian neural network-based CADx system for the characterization of CRPs. Results of conducted experiments demonstrate that this Bayesian variational inference-based approach is capable of quantifying model uncertainty along with calibration confidence. This framework is able to obtain classification accuracy comparable to the deterministic version of the network, while achieving a 24.65% and 9.14% lower Expected Calibration Error (ECE) compared to the uncalibrated and calibrated deterministic network using a postprocessing calibration technique, respectively.
更多查看译文
关键词
Colorectal polyp characterization, Bayesian inference, Model calibration, Classification uncertainty
AI 理解论文
溯源树
样例
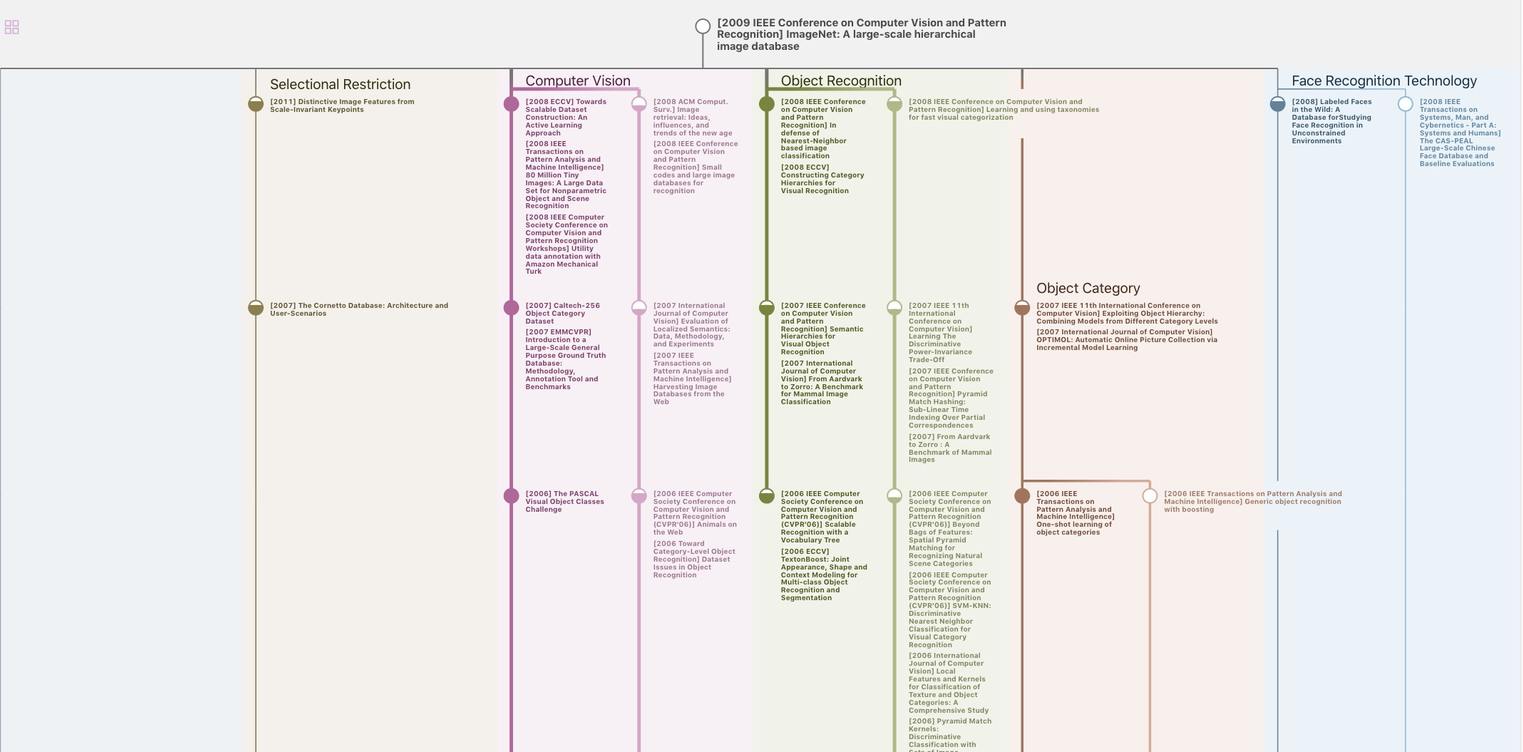
生成溯源树,研究论文发展脉络
Chat Paper
正在生成论文摘要