Fast Nonlinear Model Predictive Control Using LSTM Networks: A Model Linearisation Approach
2022 30th Mediterranean Conference on Control and Automation (MED)(2022)
摘要
This work describes a fast Model Predictive Control (MPC) algorithm in which Long Short-Term Memory (LSTM) networks are used to model dynamical processes. To obtain a computationally simple quadratic optimisation MPC task, a linear approximation of the model is repeatedly determined on-line using an original linearisation method that is specially tailored for the LSTM model. For a benchmark polymerisation process, it is shown that the described approach results in more precise prediction and better control quality than the classical model linearisation method. It is also shown that the described algorithm gives very similar control quality to that observed in MPC with nonlinear optimisation.
更多查看译文
关键词
fast nonlinear Model Predictive Control,LSTM networks,Model linearisation approach,fast Model Predictive Control algorithm,Long Short-Term Memory networks,model dynamical processes,computationally simple quadratic optimisation MPC task,original linearisation method,LSTM model,benchmark polymerisation process,described approach results,classical model linearisation method,described algorithm,similar control quality,nonlinear optimisation
AI 理解论文
溯源树
样例
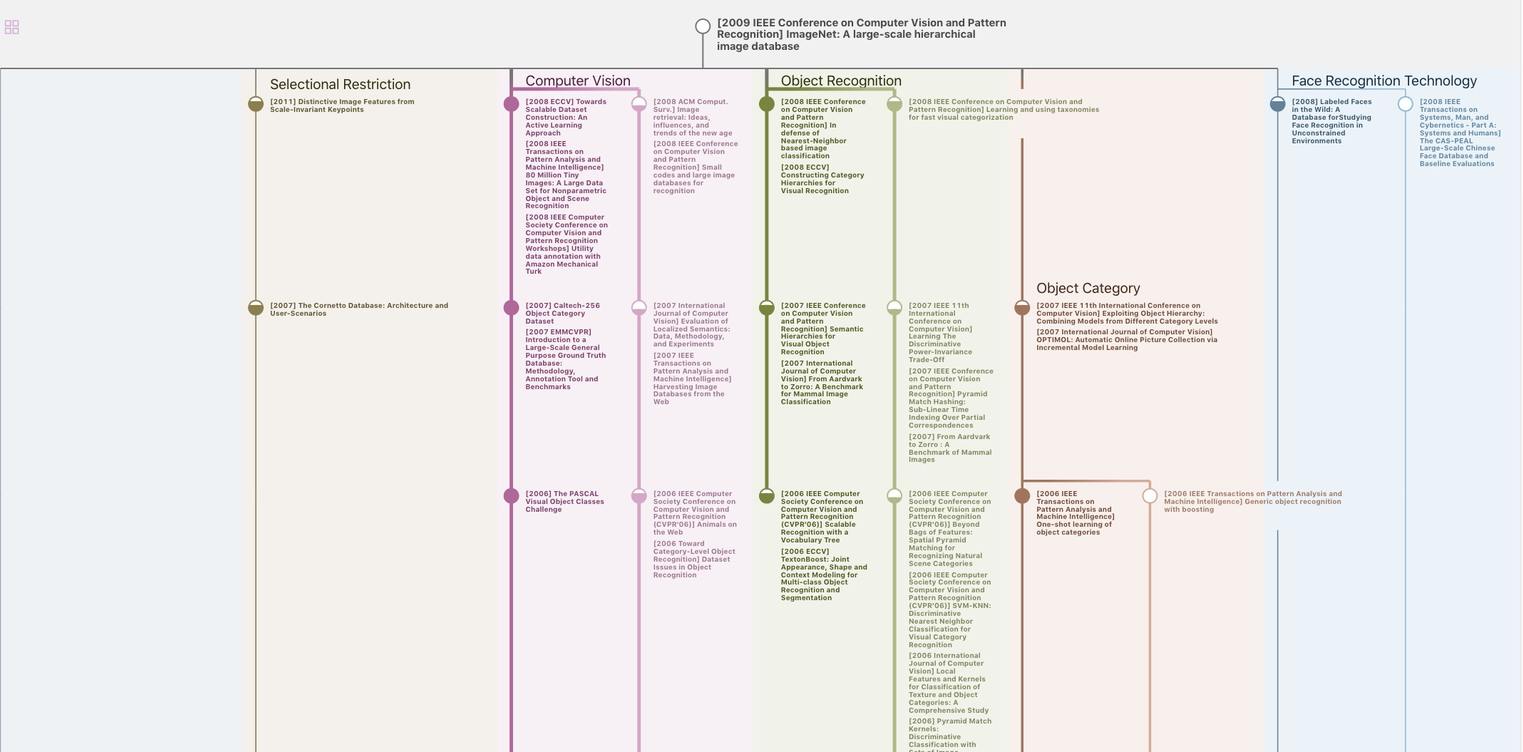
生成溯源树,研究论文发展脉络
Chat Paper
正在生成论文摘要