On Asymptotic Stability of Nonlinear Systems with Deep Reinforcement Learning Controllers
2022 30th Mediterranean Conference on Control and Automation (MED)(2022)
摘要
Controlling systems with learning-based control strategies is attracting the interest of the research community due to the advantages that machine learning offers, such as the possibility of controlling nonlinear systems that would be hard to control with conventional techniques, the possibility of controlling systems whose model is not available and so on. Reinforcement Learning (RL) and Deep Neural Networks (DNN) can be merged to obtain Deep Reinforcement Learning (DRL) control strategies. Yet, such new approaches are implemented only in few real-world applications since classical DRL control policies cannot guarantee asymptotic stability, which is a key requirement to guarantee safety. In this work, we propose a framework that, after extracting the DRL control policy, tries to synthesise a Lyapunov function that certifies the asymptotic stability of the system controlled with such a policy. We also show that our framework paves the way for safety guarantees that are often necessary when deriving a control policy. Results show that Lyapunov functions can be synthesised for the considered benchmark systems, thus ensuring asymptotic stability. Furthermore, the corresponding regions of attraction prove the quality of DRL control policies wrt other state-of-the-art learning-based controls.
更多查看译文
关键词
Lyapunov stability,Regions of attraction,Deep Reinforcement Learning,Actor-Critic
AI 理解论文
溯源树
样例
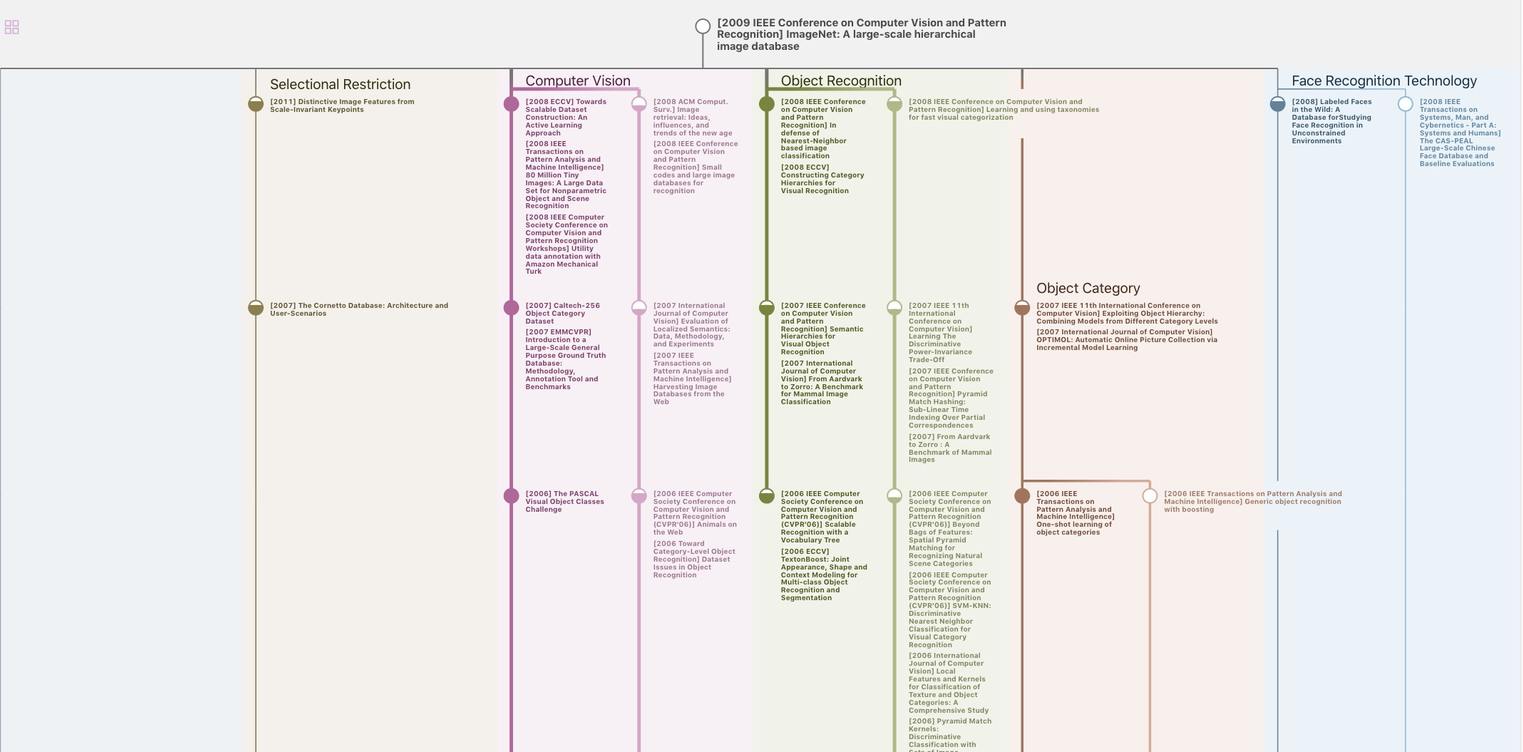
生成溯源树,研究论文发展脉络
Chat Paper
正在生成论文摘要