Explanatory artificial intelligence (YAI): human-centered explanations of explainable AI and complex data
DATA MINING AND KNOWLEDGE DISCOVERY(2022)
摘要
In this paper we introduce a new class of software tools engaged in delivering successful explanations of complex processes on top of basic Explainable AI (XAI) software systems. These tools, that we call cumulatively Explanatory AI (YAI) systems, enhance the quality of the basic output of a XAI by adopting a user-centred approach to explanation that can cater to the individual needs of the explainees with measurable improvements in usability. Our approach is based on Achinstein’s theory of explanations, where explaining is an illocutionary (i.e., broad yet pertinent and deliberate) act of pragmatically answering a question. Accordingly, user-centrality enters in the equation by considering that the overall amount of information generated by answering all questions can rapidly become overwhelming and that individual users may perceive the need to explore just a few of them. In this paper, we give the theoretical foundations of YAI, formally defining a user-centred explanatory tool and the space of all possible explanations, or explanatory space , generated by it. To this end, we frame the explanatory space as an hypergraph of knowledge and we identify a set of heuristics and properties that can help approximating a decomposition of it into a tree-like representation for efficient and user-centred explanation retrieval. Finally, we provide some old and new empirical results to support our theory, showing that explanations are more than textual or visual presentations of the sole information provided by a XAI.
更多查看译文
关键词
Explanatory AI,Explainable AI,Theory of explanations,Human–computer interaction,Human-centred explanations
AI 理解论文
溯源树
样例
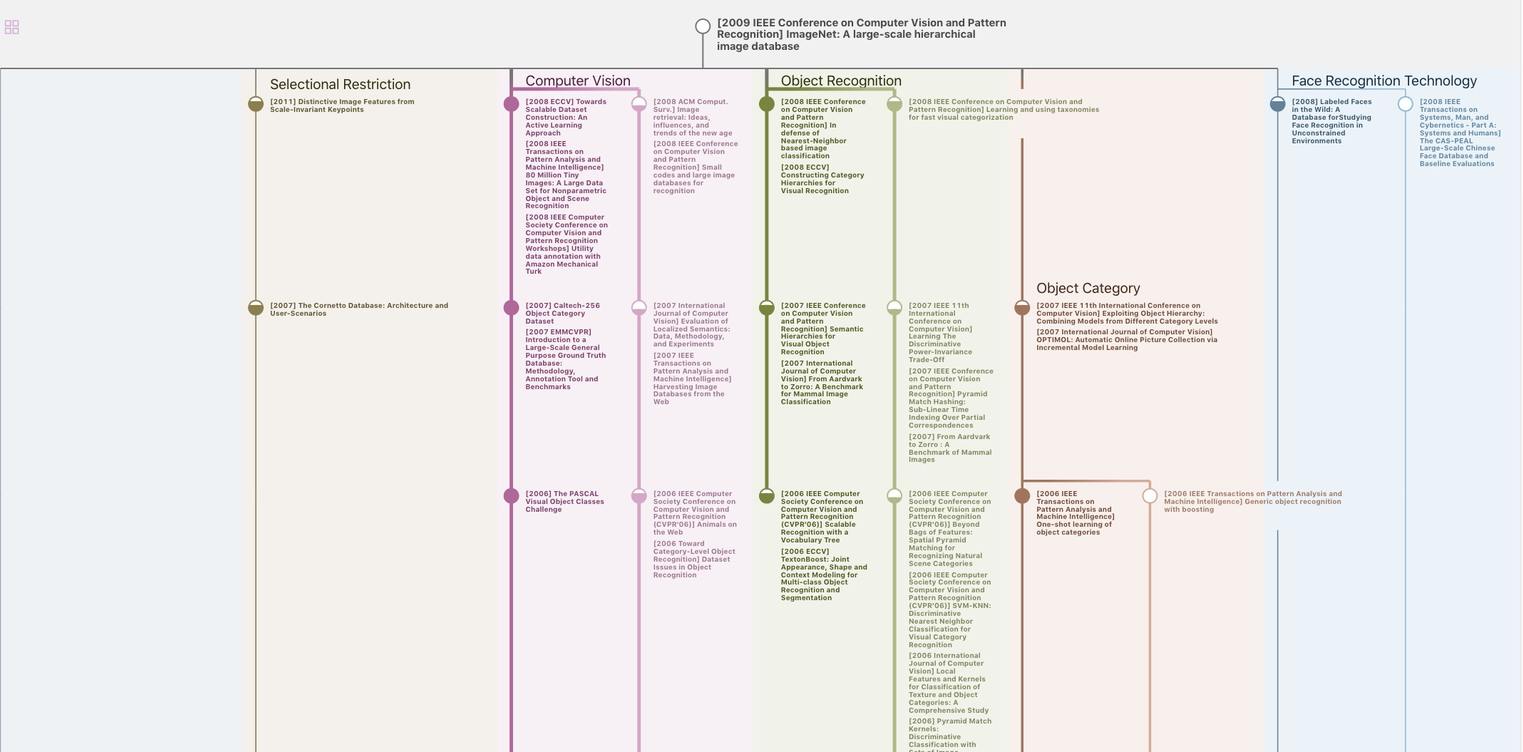
生成溯源树,研究论文发展脉络
Chat Paper
正在生成论文摘要