ASCUE: An Adversarial Network-Based Semantical Conformance Checking Method for Unsupervised Event Extraction in Social Internet of Things
COMPUTER JOURNAL(2022)
摘要
Event extraction (EE) methods are widely used in the Social Internet of Things (SIoT) to help objects obtain the key information from messages shared by other objects. Existing supervised EE methods can only extract predefined events and can hardly extract events with unseen event types. To address this issue, we propose an unsupervised EE method based on the idea of semantic role labeling. However, after extracting all possible events from a given message text, some of these events face the semantically inconsistent issue that will destroy the information credibility in SIoT. To solve this issue, we present ASCUE, an adversarial network-based semantic conformance checking method for unsupervised EE in SIoT. Briefly, ASCUE first mines all the event candidates from a given text unsupervisedly. Next, ASCUE introduces two independent Bidirectional Encoder Representations from Transformers models to capture the semantics of the event candidate and text, respectively. Moreover, to enable the model to achieve competitive performance as label embedding for semantic conformance checking, an adversarial network is added into ASCUE's training stage. Finally, ASCUE extracts all the events that are semantically consistent with the original text by classifying them into two categories: semantically consistent and semantically inconsistent. In addition, since the existing datasets do not label whether the events are semantically consistent with the original text, we re-annotate an existing dataset to fit our task. Experimental results on the re-annotated dataset show that our model outperforms the state-of-the-art baselines in terms of accuracy and F1 scores.
更多查看译文
关键词
adversarial network, semantical conformance checking, unsupervised event Extraction, Social Internet of Things
AI 理解论文
溯源树
样例
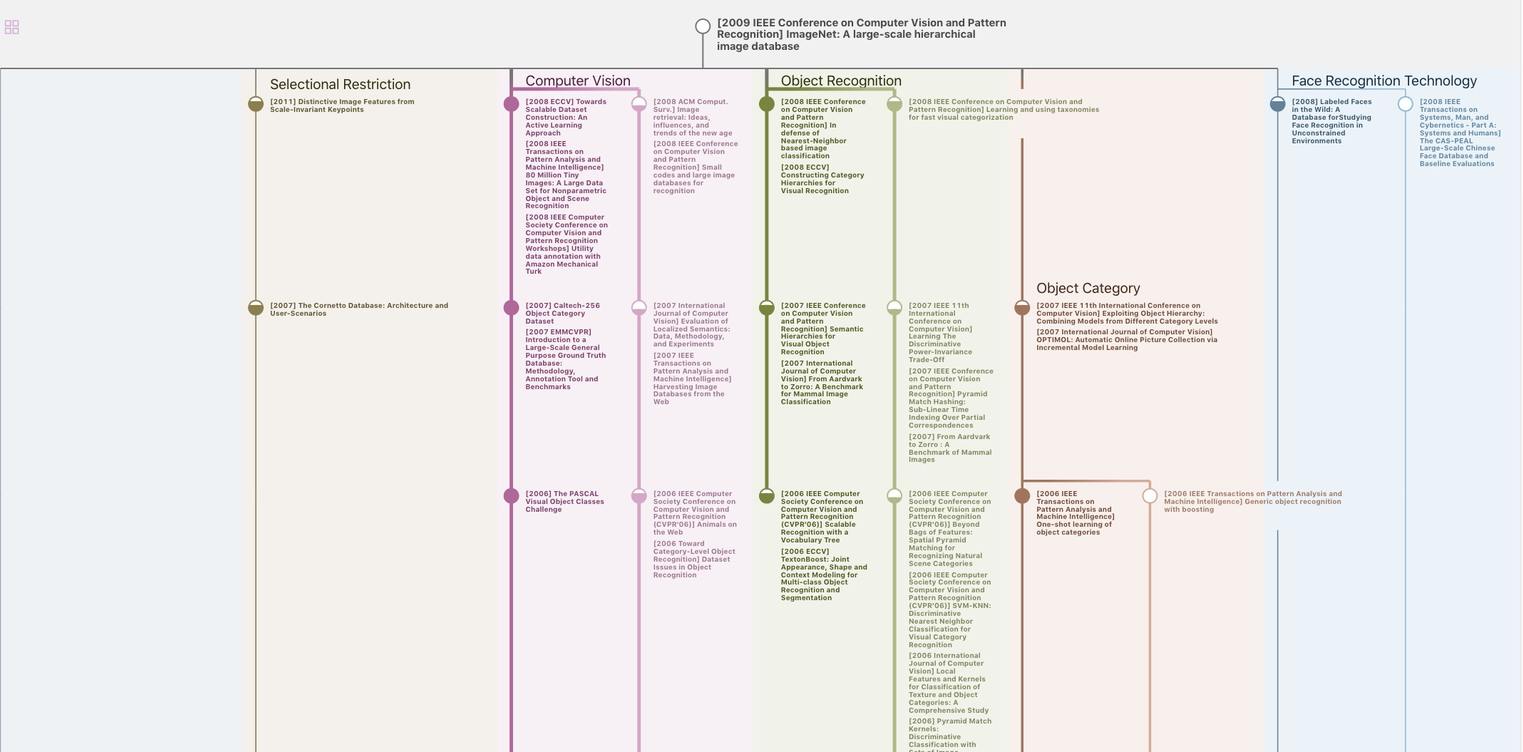
生成溯源树,研究论文发展脉络
Chat Paper
正在生成论文摘要