Novel channel selection model based on graph convolutional network for motor imagery
COGNITIVE NEURODYNAMICS(2022)
摘要
Multi-channel electroencephalography (EEG) is used to capture features associated with motor imagery (MI) based brain-computer interface (BCI) with a wide spatial coverage across the scalp. However, redundant EEG channels are not conducive to improving BCI performance. Therefore, removing irrelevant channels can help improve the classification performance of BCI systems. We present a new method for identifying relevant EEG channels. Our method is based on the assumption that useful channels share related information and that this can be measured by inter-channel connectivity. Specifically, we treat all candidate EEG channels as a graph and define channel selection as the problem of node classification on a graph. Then we design a graph convolutional neural network (GCN) model for channels classification. Channels are selected based on the outputs of our GCN model. We evaluate our proposed GCN-based channel selection (GCN-CS) method on three MI datasets. On three datasets, GCN-CS achieves performance improvements by reducing the number of channels. Specifically, we achieve classification accuracies of 79.76% on Dataset 1, 89.14% on Dataset 2 and 87.96% on Dataset 3, which outperform competing methods significantly.
更多查看译文
关键词
Brain-computer interface(BCI), Motor imagery(MI), Graph convolutional neural network (GCN), Channel selection
AI 理解论文
溯源树
样例
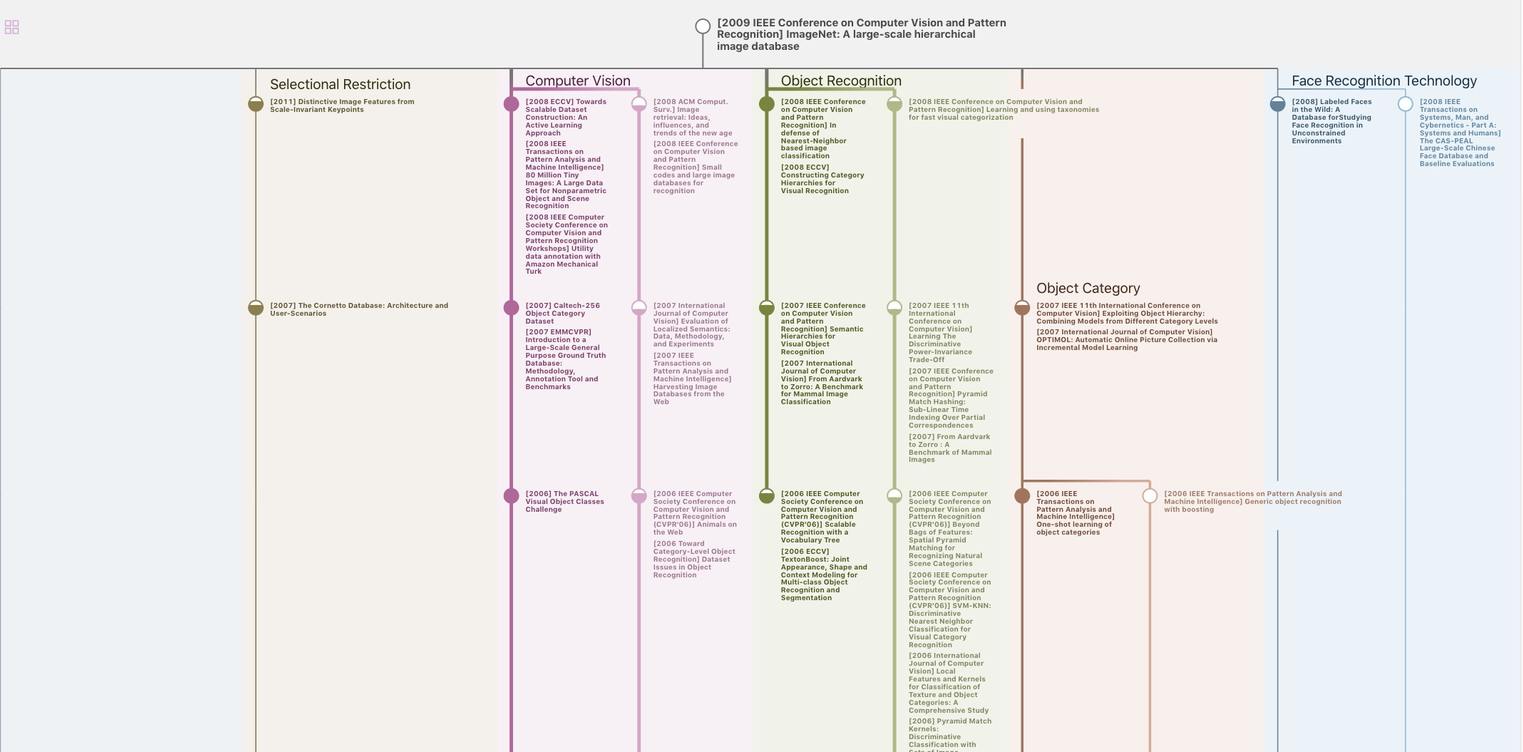
生成溯源树,研究论文发展脉络
Chat Paper
正在生成论文摘要