Hyperspectral Endoscopy Using Deep Learning for Laryngeal Cancer Segmentation
ARTIFICIAL NEURAL NETWORKS AND MACHINE LEARNING - ICANN 2022, PT IV(2022)
摘要
We propose an efficient hyperspectral imaging (HSI) Deep Learning system for laryngeal cancer prediction. An in-vivo data set with 13 hyperspectral (HS) cubes of malignant laryngeal tumors has been collected directly from 13 different patients at Klinikum Braunschweig using a HS camera. In such medical applications, the data set is usually very sparse, limited, complex and noisy. Therefore, an efficient HSI Deep Learning system is highly needed. First, a relevant wavelength analysis has been done in order to detect the most informative channels in the HS cubes to speed up the prediction and reduce the noise. Based on the results, a new UNet, called Efficient Exception UNet (EFX-UNet), is devised and the two channels No. 13 and No. 14 from each cube are used for training and prediction. The EFX-UNet predicts the probability of the patient having a malignant tumor and yields an initial segmentation. After that a deeper analysis is carried on for the detected patients using the 8 channels No. 9 to No. 16 with our modified Deep UNet to precisely segment the tumor. The system is tested on real patient data. With EFXUNet we could reduce the prediction time from 60 s with Deep UNet to only 5 s. Although the data set is very limited and very complex, we could achieve a dice score test of 68.6% with EFX-UNet for initial segmentation and 88% with the Deep UNet in our system.
更多查看译文
关键词
Deep Learning, Hyperspectral imaging, Cancer detection
AI 理解论文
溯源树
样例
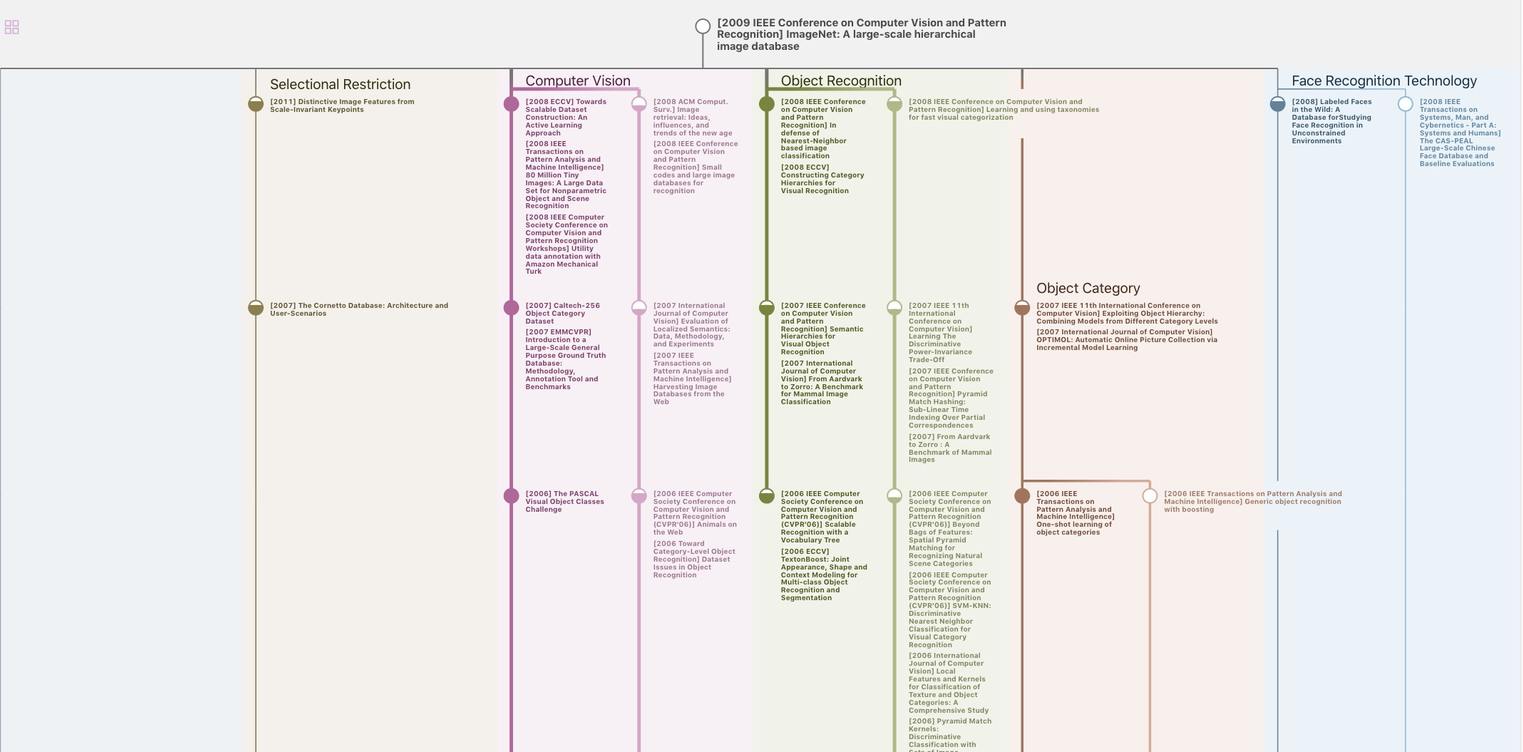
生成溯源树,研究论文发展脉络
Chat Paper
正在生成论文摘要