Image-Based Detection of Structural Defects Using Hierarchical Multi-scale Attention
PATTERN RECOGNITION, DAGM GCPR 2022(2022)
摘要
With improving acquisition technologies, the inspection and monitoring of structures has become a field of application for deep learning. While other research focuses on the design of neural network architectures, this work points out the applicability of transfer learning for detecting cracks and other structural defects. Being a high-performer on the Cityscapes benchmark, hierarchical multi-scale attention [43] also renders suitable for transfer learning in the domain of structural defects. Using the joint scales of 0.25, 0.5, and 1.0, the approach achieves 92% mean intersection-over-union on the test set. The effectiveness of multi-scale attention is demonstrated for class demarcation on large scales and class determination on lower scales. Furthermore, a line-based tolerant intersection-over-union metric is introduced for more robust benchmarking in the field of crack detection. The dataset of 743 images covering crack, spalling, corrosion, efflorescence, vegetation, and control point is unprecedented in terms of quantity and realism.
更多查看译文
关键词
Deep learning, Structural defects, Crack detection, Hierarchical multi-scale attention
AI 理解论文
溯源树
样例
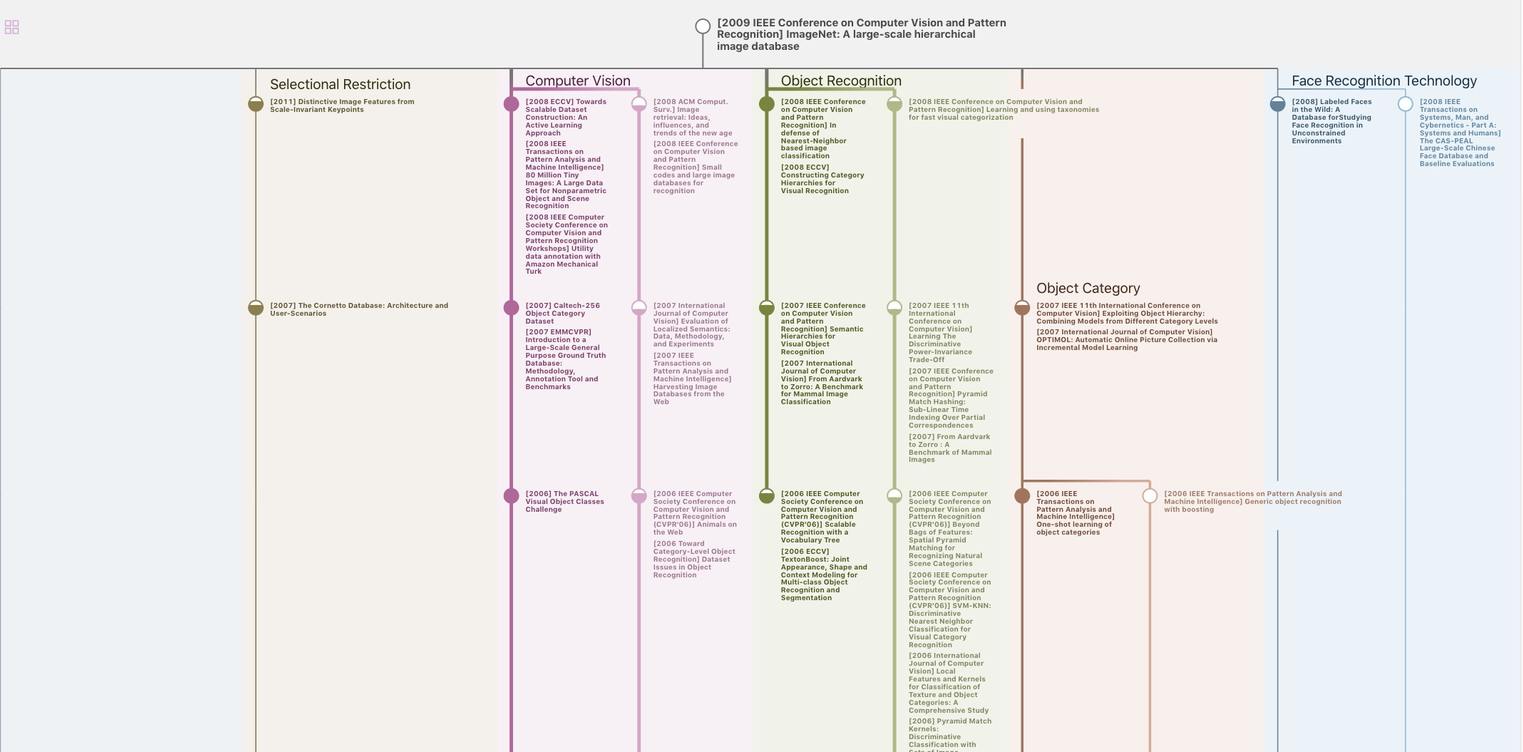
生成溯源树,研究论文发展脉络
Chat Paper
正在生成论文摘要