GCNMFCDA: A Method Based on Graph Convolutional Network and Matrix Factorization for Predicting circRNA-Disease Associations
INTELLIGENT COMPUTING THEORIES AND APPLICATION, ICIC 2022, PT II(2022)
摘要
Numerous studies reveal that Circular RNAs (circRNAs) are critical for human physiological and pathological processes. Research on the disease-related circRNAs can provide insight into the mechanisms of extraordinary disease and benefit the prevention and therapy of numerous untreated human diseases. As biological experiments require a great deal of labor and time, the calculation-based methods have become more and more salient in the field. Here we present a brand-new model named GCNMFCDA, which contains Graph Convolutional Network (GCN) and Matrix Factorization (MF) to predict disease-related circRNAs. Theoretically, we first utilize circRNA and disease similarities to construct initial node features. Then we apply GCN to learn potential embeddings of circRNA and disease based on initial node features. Finally, the inner product between circRNA embedding and disease embedding is introduced to construct the new score matrix based on matrix factorization. We adopt fivefold and tenfold cross validation to evaluate our model. GCNMFCDA obtains an average AUC of 0.9330 and 0.9290, respectively, and performs better than the other eleven existing methods. Furthermore, case studies on breast cancer and glioma illustrate that 19 and 18 of the top 20 candidate circRNAs were respectively confirmed in the validation datasets or published literature. These experimental results reveal that GCNMFCDA can discover circRNA-disease associations effectively and reliably.
更多查看译文
关键词
Graph Convolutional Network, Matrix factorization, circRNA-disease association
AI 理解论文
溯源树
样例
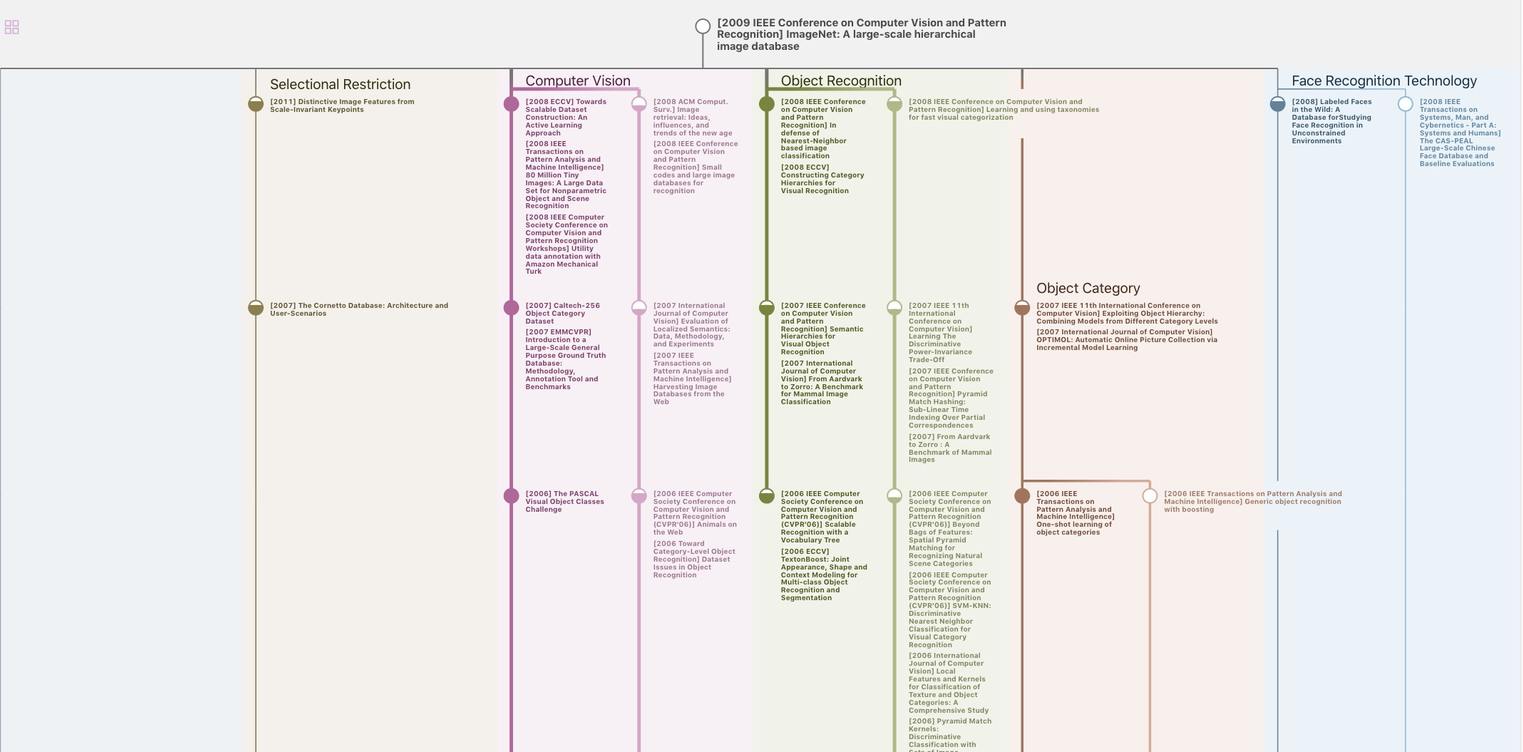
生成溯源树,研究论文发展脉络
Chat Paper
正在生成论文摘要