Self-supervised Prototype Conditional Few-Shot Object Detection
IMAGE ANALYSIS AND PROCESSING, ICIAP 2022, PT II(2022)
摘要
Traditional deep learning-based object detection methods require a large amount of annotation for training, and creating such a dataset is expensive. Few-shot object detection which detects a new category of objects with a small amount of data is a difficult task. Many previous methods have been applied to detect new categories with few data by fine-tuning. However, fine-tuning methods cannot be sufficiently adapted quickly to new environments because of time-consuming training. In this study, we develop methods that can be adapted to new categories without fine-tuning. A prototype conditional detection module built on prototypes for each category is intended to be conditioned and detected by prototypes. Moreover, a self-supervised conditional detection module improves the representational capability for detecting new categories by a self-supervised task that uses embeddings outside the annotation region to detect the same regions before and after image transformation. The results of extensive experiments on the PASCAL VOC and MS COCO show the effectiveness of the proposed method.
更多查看译文
关键词
Few-shot object detection, Few-shot learning, Object detection
AI 理解论文
溯源树
样例
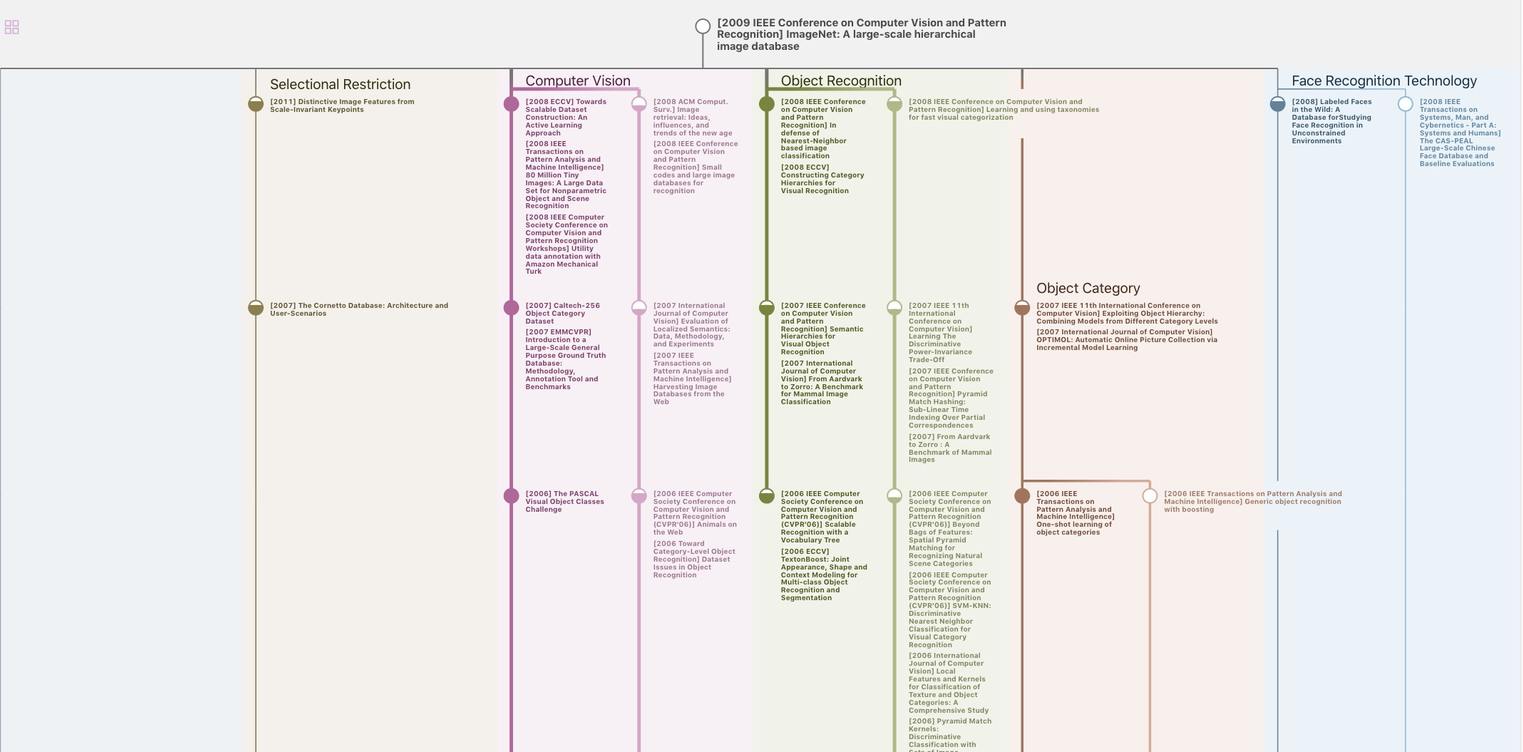
生成溯源树,研究论文发展脉络
Chat Paper
正在生成论文摘要