Image Novelty Detection Based on Mean-Shift and Typical Set Size
IMAGE ANALYSIS AND PROCESSING, ICIAP 2022, PT II(2022)
摘要
The detection of anomalous or novel images given a training dataset of only clean reference data (inliers) is an important task in computer vision. We propose a new shallow approach that represents both inlier and outlier images as ensembles of patches, which allows us to effectively detect novelties as mean shifts between reference data and outliers with the Hotelling T-2 test. Since mean-shift can only be detected when the outlier ensemble is sufficiently separate from the typical set of the inlier distribution, this typical set acts as a blind spot for novelty detection. We therefore minimize its estimated size as our selection rule for critical hyperparameters, such as, e.g., the size of the patches is crucial. To showcase the capabilities of our approach, we compare results with classical and deep learning methods on the popular datasets MNIST and CIFAR-10, and demonstrate its real-world applicability in a large-scale industrial inspection scenario.
更多查看译文
关键词
Image novelty detection, Independent component analysis, Mean-shift
AI 理解论文
溯源树
样例
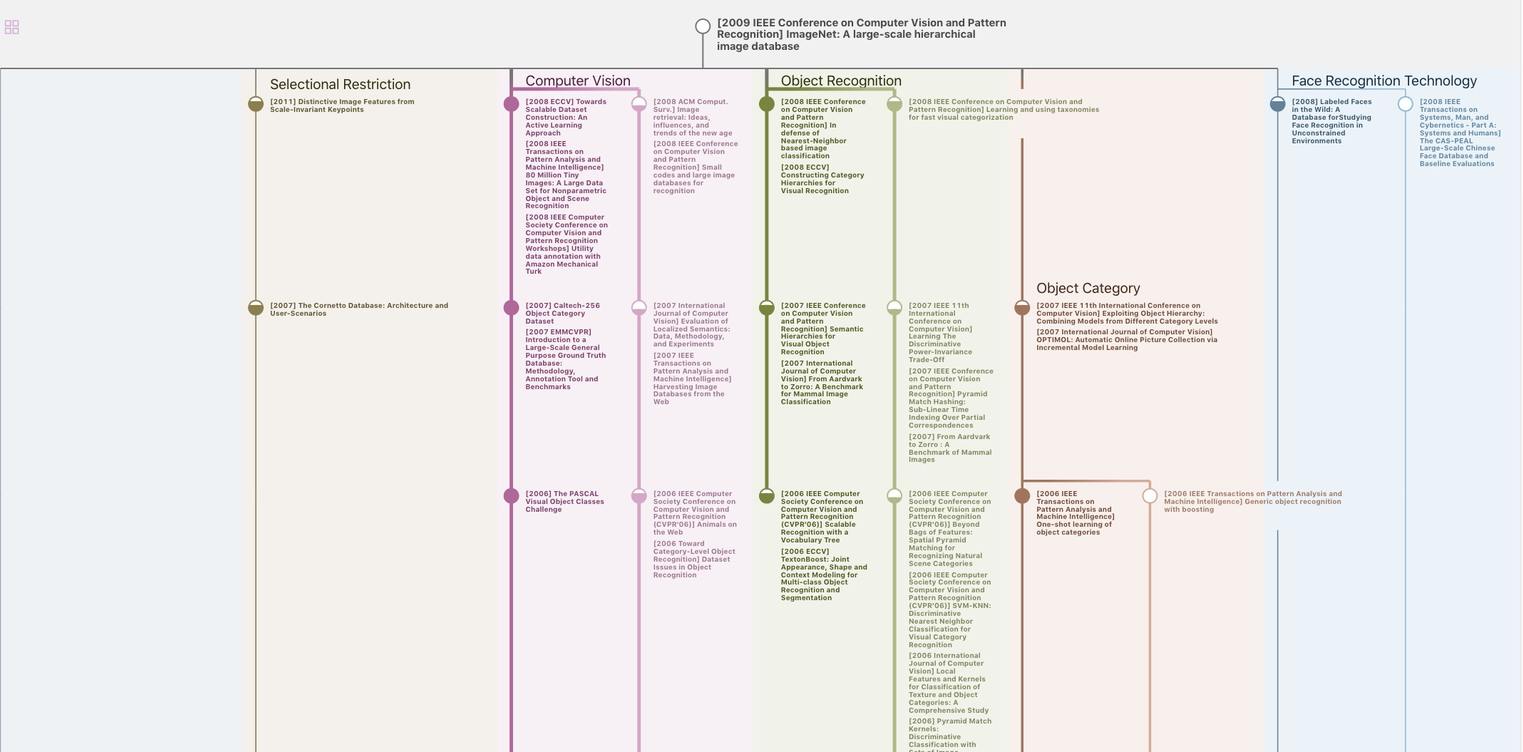
生成溯源树,研究论文发展脉络
Chat Paper
正在生成论文摘要