Case Study on the Use of the SafeML Approach in Training Autonomous Driving Vehicles
IMAGE ANALYSIS AND PROCESSING, ICIAP 2022, PT III(2022)
摘要
The development quality for the control software for autonomous vehicles is rapidly progressing, so that the control units in the field generally perform very reliably. Nevertheless, fatal misjudgments occasionally occur putting people at risk: such as the recent accident in which a Tesla vehicle in Autopilot mode rammed a police vehicle. Since the object recognition software which is a part of the control software is based on machine learning (ML) algorithms at its core, one can distinguish a training phase from a deployment phase of the software. In this paper we investigate to what extent the deployment phase has an impact on the robustness and reliability of the software; because just as traditional, software based on ML degrades with time. A widely known effect is the so-called concept drift: in this case, one finds that the deployment conditions in the field have changed and the software, based on the outdated training data, no longer responds adequately to the current field situation. In a previous research paper, we developed the SafeML approach with colleagues from the University of Hull, where datasets are compared for their statistical distance measures. In doing so, we detected that for simple, benchmark data, the statistical distance correlates with the classification accuracy in the field. The contribution of this paper is to analyze the applicability of the SafeML approach to complex, multidimensional data used in autonomous driving. In our analysis, we found that the SafeML approach can be used for this data as well. In practice, this would mean that a vehicle could constantly check itself and detect concept drift situation early.
更多查看译文
关键词
Automotive, Safety, SafeML, Machine learning, Autonomous driving
AI 理解论文
溯源树
样例
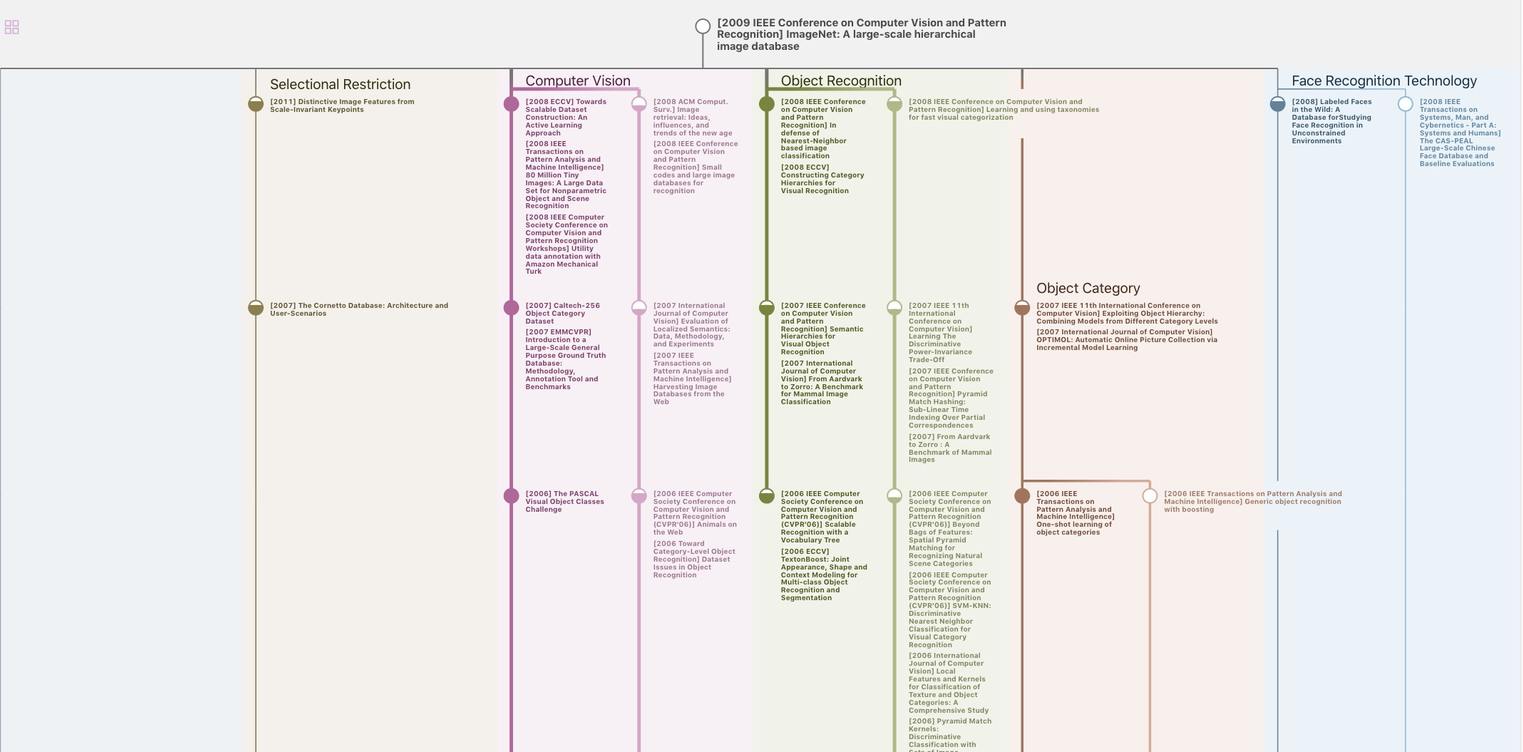
生成溯源树,研究论文发展脉络
Chat Paper
正在生成论文摘要