SCAF: Skip-Connections in Auto-encoder for Face Alignment with Few Annotated Data
IMAGE ANALYSIS AND PROCESSING, ICIAP 2022, PT I(2022)
摘要
Supervised face alignment methods need large amounts of training data to achieve good performance in terms of accuracy and generalization. However face alignment datasets rarely exceed a few thousand samples making these methods prone to overfitting on the specific training dataset. Semi-supervised methods like TS3 or 3FabRec have emerged to alleviate this issue by using labeled and unlabeled data during the training. In this paper we propose Skip-Connections in Auto-encoder for Face alignment (SCAF), we build on 3FabRec by adding skip-connections between the encoder and the decoder. These skip-connections lead to better landmark predictions, especially on challenging examples. We also apply for the first time active learning to the face alignment task and introduce a new acquisition function, the Negative Neighborhood Magnitude, specially designed to assess the quality of heatmaps. These two proposals show their effectiveness on several face alignment datasets when training with limited data.
更多查看译文
关键词
Face alignment, Semi-supervised training, Active learning
AI 理解论文
溯源树
样例
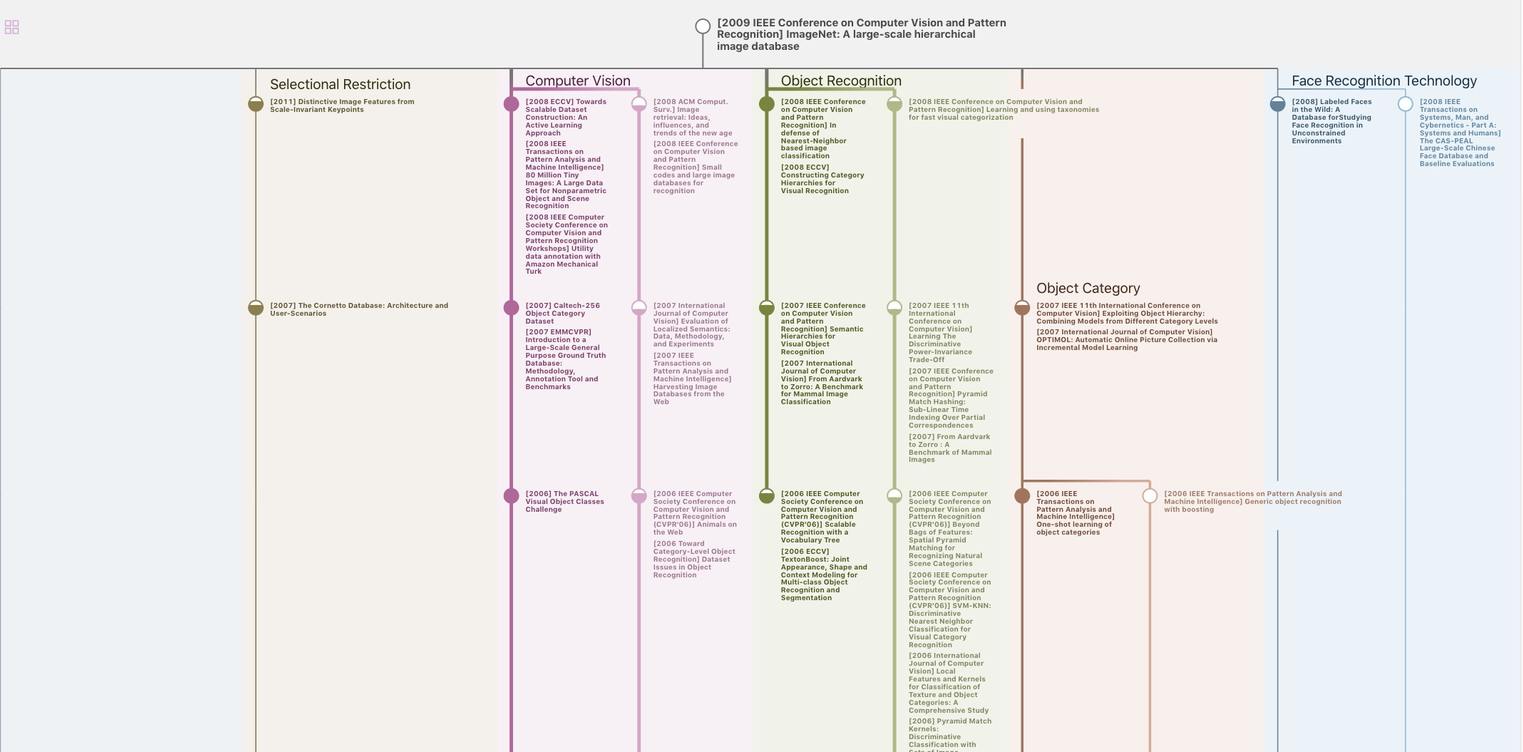
生成溯源树,研究论文发展脉络
Chat Paper
正在生成论文摘要