Unsupervised Deformable Image Registration in a Landmark Scarcity Scenario: Choroid OCTA
IMAGE ANALYSIS AND PROCESSING, ICIAP 2022, PT I(2022)
摘要
Recent advances in OCTA allow the imaging of blood flow deeper than the retinal layers at the level of the choriocapillaris (CC), where a pattern of small dark areas represents the absence of flow, called flow voids. The distribution of flow voids can be used as a biomarker to diagnose and monitor the progression of relevant pathologies or the efficacy of applied treatments. A pixel-to-pixel comparison can help to carry out this monitoring effectively, although in order to carry out this comparison, the used images must be perfectly aligned. CC images are characterized by their granularity, presenting numerous and complex local deformations, so a deformable registration is necessary to carry out a reliable comparison. However, CC OCTA images also present a characteristic absence of visually significant anatomical structures. This landmark scarcity hardens drastically the identification of points of interest to achieve an accurate registration. Based on this context, we designed a methodology to accurately perform this deformable registration in this challenging scenario. Hence, we propose a convolutional neural network model trained by unsupervised learning to register images in a real clinical scenario, being obtained at different time instants from patients with central serous chorioretinopathy (CSC) treated with photodynamic therapy. Our methodology produces superior alignment to those achieved with other proven methods, helping to improve the monitoring of the efficacy of photodynamic therapy applied to patients with CSC. Our robust and adaptable methodology can also be exploited in other similar scenarios of complex registrations with anatomical landmark scarcity.
更多查看译文
关键词
Ophthalmology, OCTA imaging, Choriocapillaris, Deformable image registration, Flow voids, Convolutional neural networks
AI 理解论文
溯源树
样例
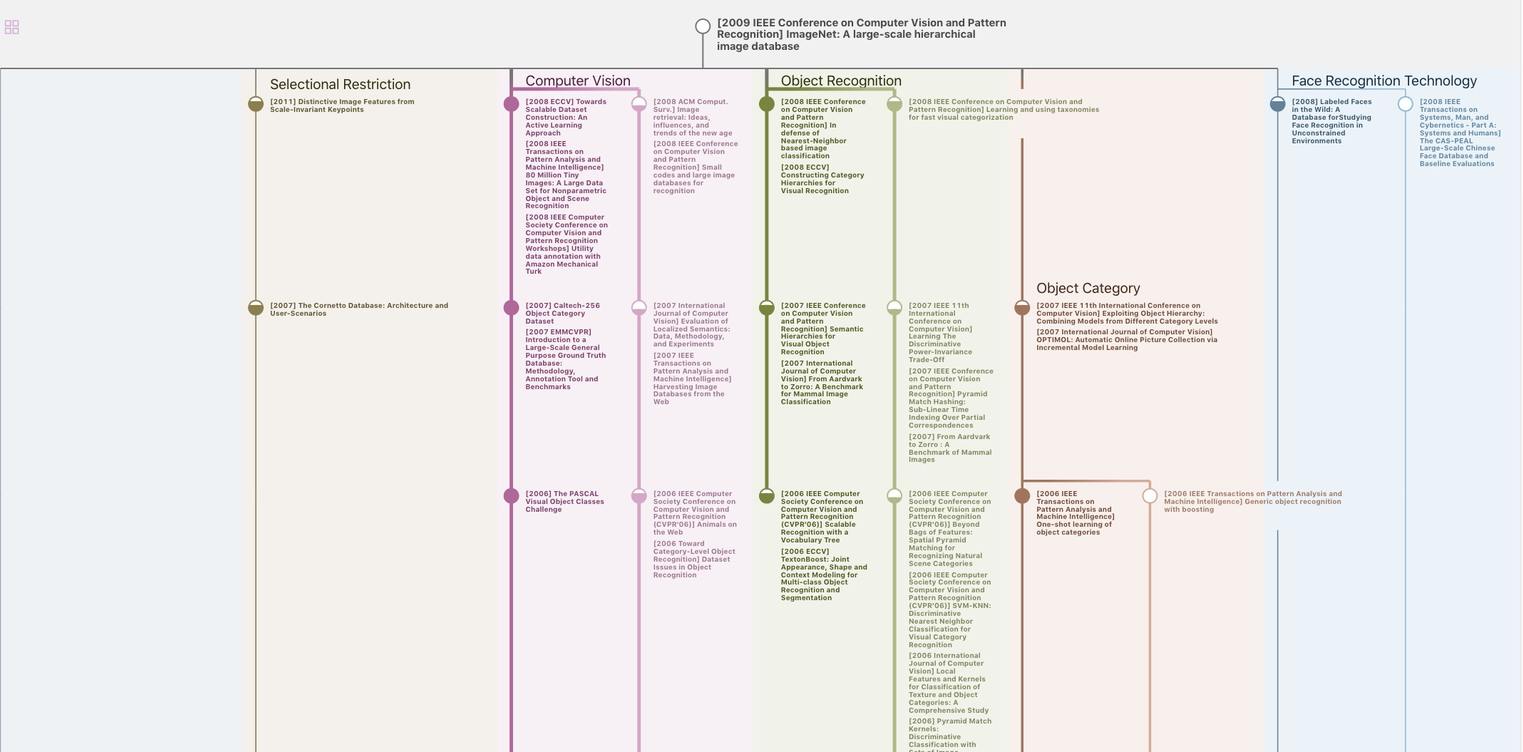
生成溯源树,研究论文发展脉络
Chat Paper
正在生成论文摘要