SVP-Classifier: Single-View Point Cloud Data Classifier with Multi-view Hallucination
IMAGE ANALYSIS AND PROCESSING, ICIAP 2022, PT II(2022)
摘要
We address single-view 3D shape classification with partial Point Cloud Data (PCD) inputs. Conventional PCD classifiers achieve the best performance when trained and evaluated with complete 3D object scans. However, they all experience a performance drop when trained and evaluated on partial single-view PCD. We propose a Single-View PCD Classifier (SVP-Classifier), which first hallucinates the features of other viewpoints covering the unseen part of the object with a Conditional Variational Auto-Encoder (CVAE). It then aggregates the hallucinated multi-view features with a multi-level Graph Convolutional Network (GCN) to form a global shape representation that helps to improve the single-view PCD classification performance. With experiments on the single-view PCDs generated from ModelNet40 and ScanObjectNN, we prove that the proposed SVP-Classifier outperforms the best single-view PCD-based methods, after they have been retrained on single-view PCDs, thus reducing the gap between single-view methods and methods that employ complete PCDs. Code and datasets are available: https://github.com/IIT-PAVIS/SVP-Classifier.
更多查看译文
关键词
Multi-view feature hallucination, 3D object classification, Partial point cloud
AI 理解论文
溯源树
样例
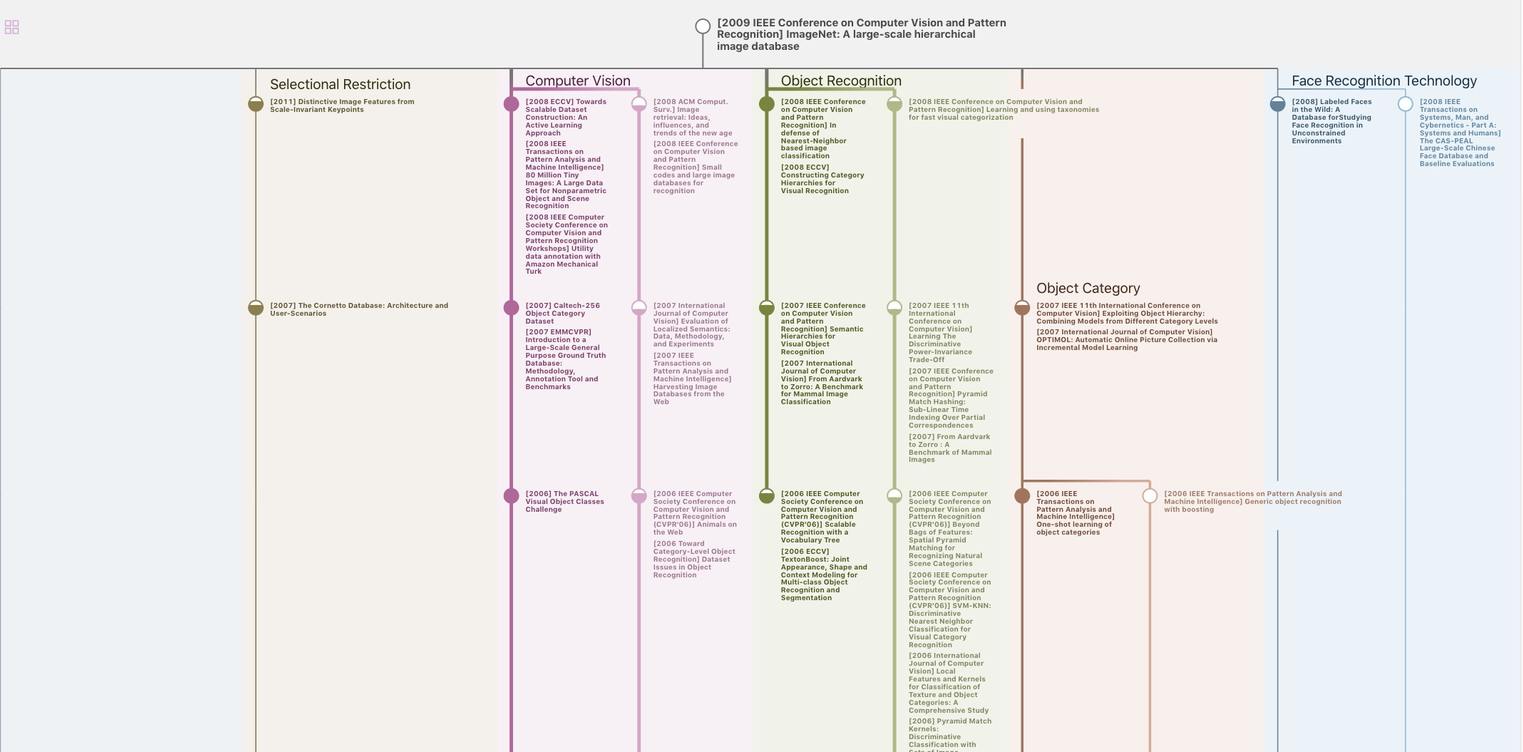
生成溯源树,研究论文发展脉络
Chat Paper
正在生成论文摘要