Self-supervised Learning Based on a Pre-trained Method for the Subtype Classification of Spinal Tumors
COMPUTATIONAL MATHEMATICS MODELING IN CANCER ANALYSIS, CMMCA 2022(2022)
摘要
Spinal tumors contain multiple pathological subtypes, and different subtypes may correspond to different treatments and prognoses. Diagnosis of spinal tumor subtypes from medical images in the early stage is of great clinical significance. Due to the complex morphology and high heterogeneity of spinal tumors, it can be challenging to diagnose subtypes from medical images accurately. In recent years, a number of researchers have applied deep learning technology to medical image analysis. However, such research usually requires a large number of labeled samples for training, which can be difficult to obtain. Therefore, the use of unlabeled medical images to improve the identification performance of models is a hot research topic. This study proposed a self-supervised learning based pre-trained method Res-MAE using a convolutional neural network and masked autoencoder. First, this method trains an efficient feature encoder using a large amount of unlabeled spinal medical data with an image reconstruction task. Then this encoder is transferred to the downstream subtype classification in a multi-modal fusion model for fine-tuning. This multi-modal fusion model adopts a bipartite graph and multi-branch for spinal tumor subtype classification. The experimental results show that the accuracy of the proposed method can be increased by up to 10.3%, and the F1 can be increased by up to 13.8% compared with the baseline method.
更多查看译文
关键词
Self-supervised learning, Pre-training model, Subtype classification
AI 理解论文
溯源树
样例
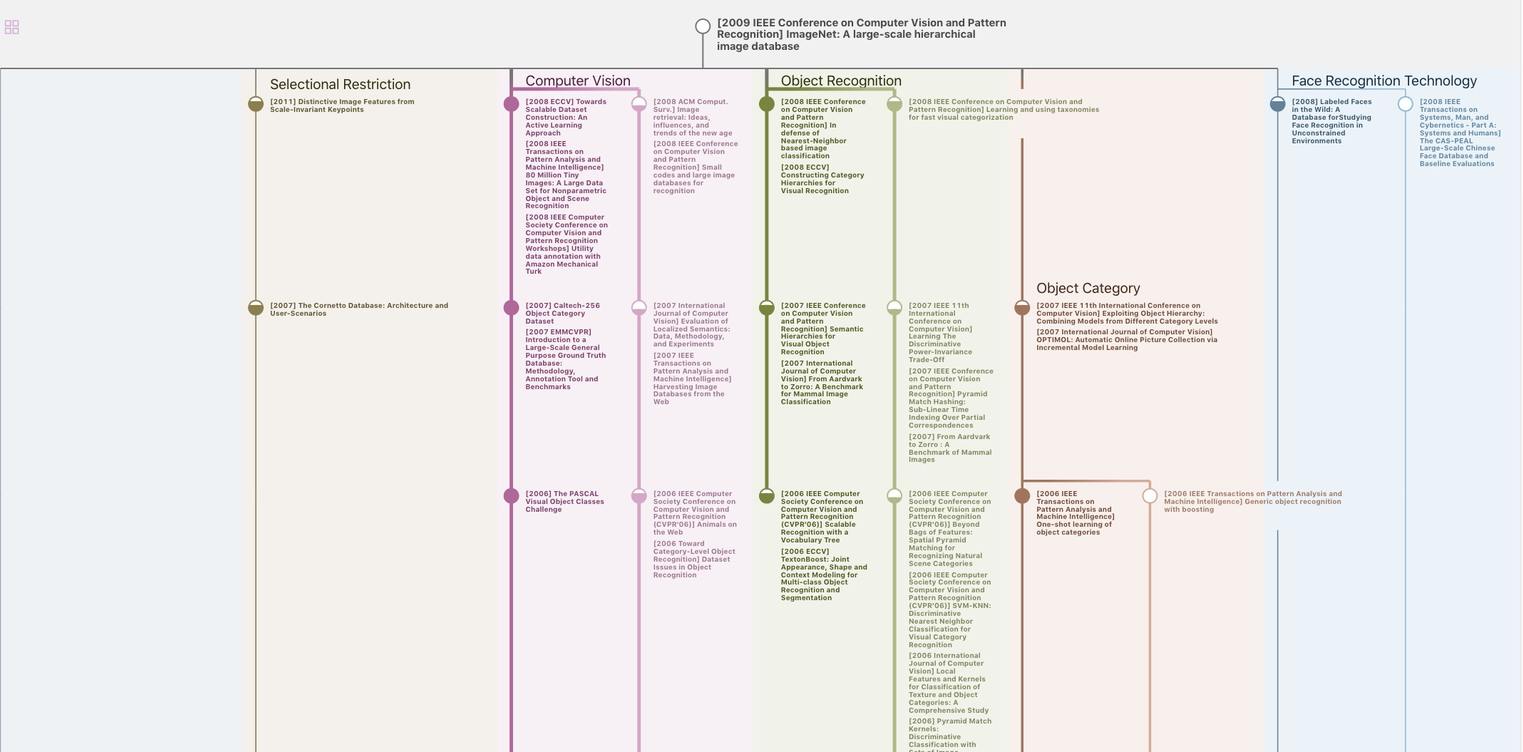
生成溯源树,研究论文发展脉络
Chat Paper
正在生成论文摘要