Early prediction of learners at risk in self-paced education: A neural network approach
Expert Systems with Applications(2023)
摘要
To address the demands of modern education and increase flexibility, many higher education institutions are considering self-paced education programs. However, student retention is yet a widely recognized challenge faced in self-paced education. While many studies have examined the potential of the use of data about student interaction with learning technologies to predict student success, studies that focus on self-paced education are scarce. To address this gap in the literature, this paper reports on the findings of a study that has investigated the performance of a well-known deep learning technique i.e., Long Short-term Memory (LSTM), in the prediction of students at risk of failing a course offered in a self-paced mode of online education. The study has utilized a freely accessible Open University Learning Analytics Dataset comprising 22,437 students with 69 % pass, and 31 % failed instances. The deep LSTM shows the highest predictive power to classify between pass and fail students, compared to all other alternatives by achieving an accuracy of 84.57 %, precision of 82.24 % and recall of 79.43 %. Interestingly, with only first five weeks of course activity log data used for training, the receiver operating characteristic based diagnostic accuracy of the LSTM algorithm is achieved up to 71 %, that outperforms almost all other conventional algorithms - despite trained on the complete dataset collected for the entire duration of the course i.e. up to 38 weeks. Furthermore, this study has also employed a shapely additive explanation model to identify the most important predictors of student retention, e.g., assessment submission and attempted quizzes. This approach is essential in order to increase the interpretability of deep learning techniques and, thus, increase their potential to generate actionable insights.
更多查看译文
关键词
Early prediction,Machine learning,Deep learning,Long short-term memory (LSTM),Students at risk,Virtual learning environment (VLE)
AI 理解论文
溯源树
样例
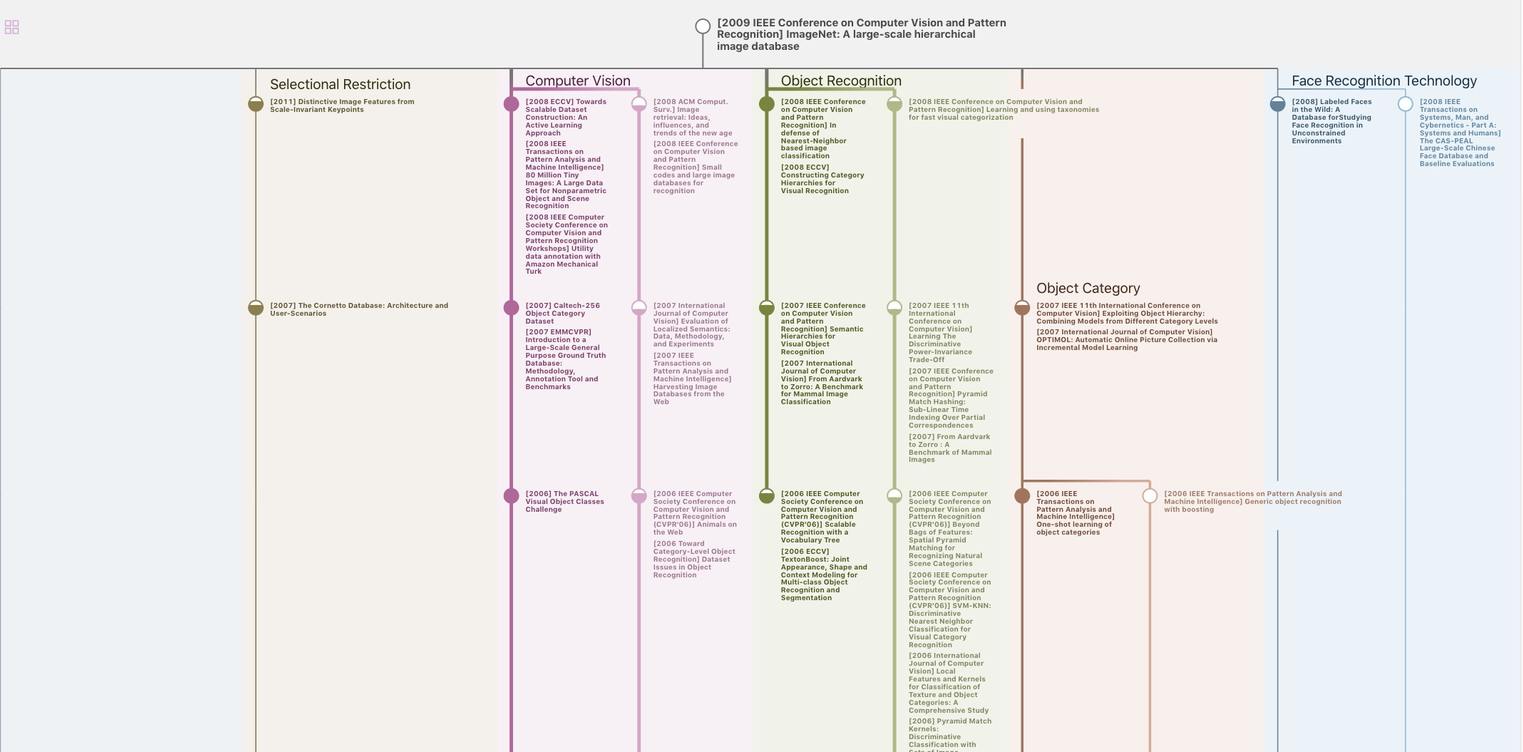
生成溯源树,研究论文发展脉络
Chat Paper
正在生成论文摘要