A BERT-Based Approach for Multilingual Discourse Connective Detection
NATURAL LANGUAGE PROCESSING AND INFORMATION SYSTEMS (NLDB 2022)(2022)
摘要
In this paper, we report on our experiments towards multilingual discourse connective (or DC) identification and show how language specific BERT models seem to be sufficient even with little task-specific training data. While some languages have large corpora with human annotated DCs, most languages are low in such resources. Hence, relying solely on discourse annotated corpora to train a DC identification system for low resourced languages is insufficient. To address this issue, we developed a model based on pretrained BERT and fine-tuned it with discourse annotated data of varying sizes. To measure the effect of larger training data, we induced synthetic training corpora with DC annotations using word-aligned parallel corpora. We evaluated our models on 3 languages: English, Turkish and Mandarin Chinese in the context of the recent DISRPT 2021 Task 2 shared task. Results show that the Fmeasure achieved by the standard BERT model (92.49%, 93.97%, 87.42% for English, Turkish and Chinese) is hard to improve upon even with larger task specific training corpora
更多查看译文
关键词
Discourse analysis, Multilingual discourse connective identification, Corpus creation
AI 理解论文
溯源树
样例
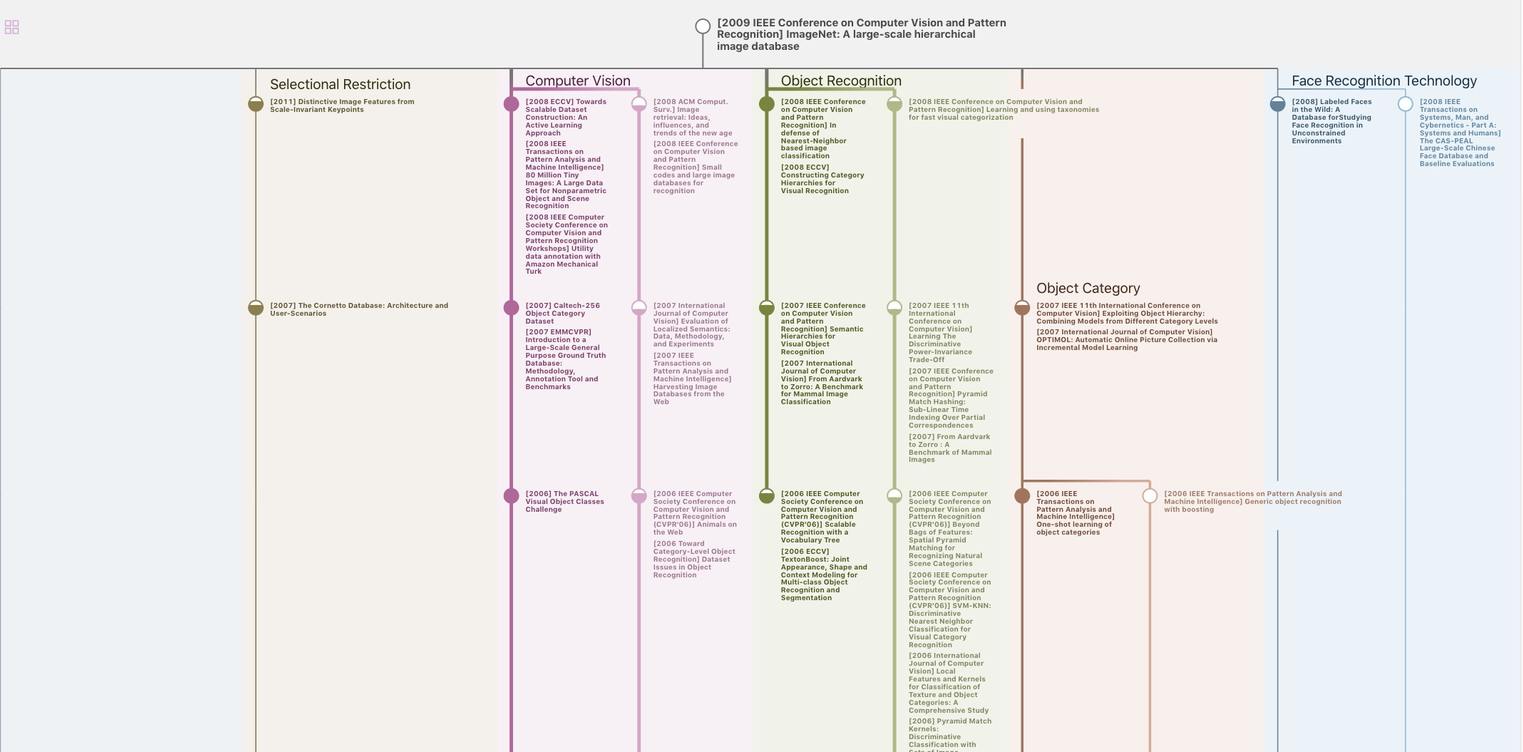
生成溯源树,研究论文发展脉络
Chat Paper
正在生成论文摘要