A Framework for False Negative Detection in NER/NEL
NATURAL LANGUAGE PROCESSING AND INFORMATION SYSTEMS (NLDB 2022)(2022)
摘要
Finding the false negatives of a NER/NEL system is fundamental to improve it, and is usually done by manual annotation of texts. However, in an environment with a huge volume of unannotated texts (e.g. a hospital) and a low frequency of positives (e.g. a mention of a particular disease in the clinical notes) the task becomes very inefficient. This paper presents a framework to tackle this problem: given an existing NER/NEL system, we propose a technique consisting of using text similarity search to rank texts by probability of containing false negatives of a given concept, using as a query those texts where the existing NER/NEL system has found positives of this concept. We formulate text similarity as a function of shared medical entities between texts, and we re-purpose an existing public dataset (CodiEsp) to propose an evaluation strategy.
更多查看译文
关键词
Natural language processing, NLP, Clinical NLP, False negatives, Document representation, Text similarity search, Named Entity Recognition, NER, Named Entity Linking, NEL
AI 理解论文
溯源树
样例
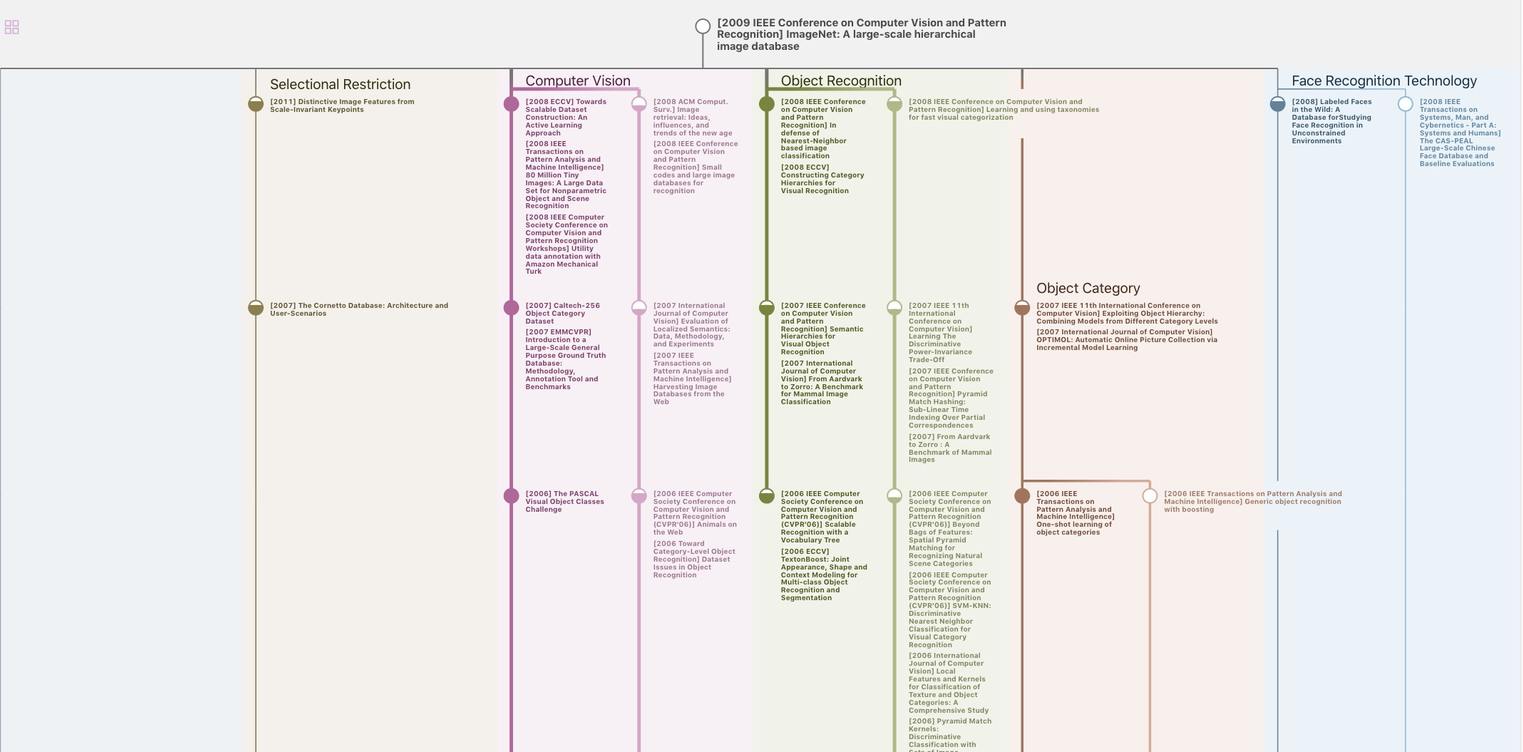
生成溯源树,研究论文发展脉络
Chat Paper
正在生成论文摘要