Predicting Molecular Traits from Tissue Morphology Through Self-interactive Multi-instance Learning
MEDICAL IMAGE COMPUTING AND COMPUTER ASSISTED INTERVENTION, MICCAI 2022, PT II(2022)
摘要
Previous efforts to learn histology features that correlate with specific genetic/molecular traits resort to tile-level multi-instance learning (MIL) which relies on a fixed pretrained model for feature extraction and an instance-bag classifier. We argue that such a two-step approach is not optimal at capturing both fine-grained features at tile level and global features at slide level optimal to the task. We propose a self-interactive MIL that iteratively feedbacks training information between the fine-grained and global context features. We validate the proposed approach on 4 subtyping tasks: EMT status (ovarian), KRAS mutation (colon and lung), EGFR mutation (colon), and HER2 status (breast). Our approach yields an average improvement of 7.05% - 8.34% (in terms of AUC) over the baseline.
更多查看译文
关键词
Histopathological subtyping, Genetic mutation detection, Limited data, Self-interactive learning, Fine-grained features
AI 理解论文
溯源树
样例
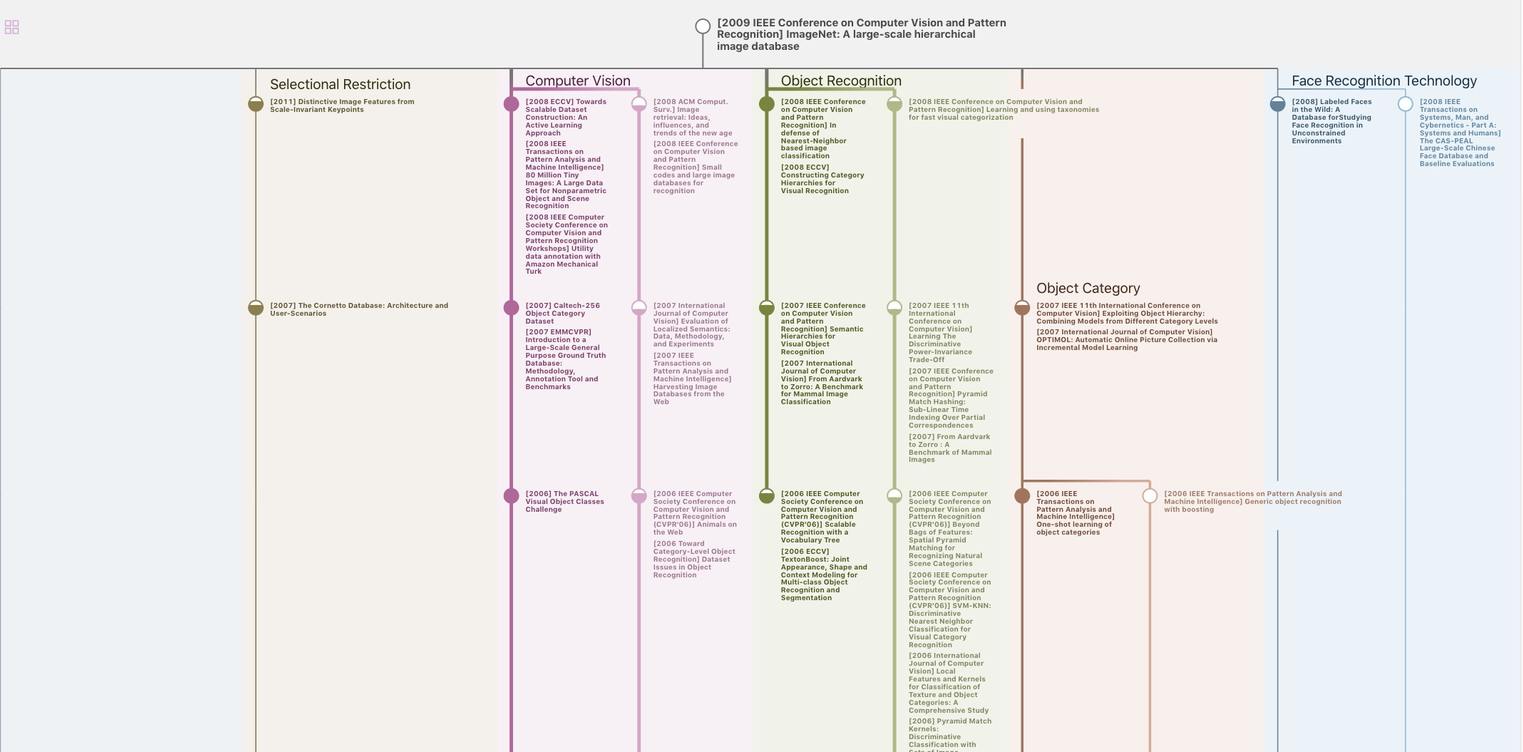
生成溯源树,研究论文发展脉络
Chat Paper
正在生成论文摘要