A Learning Approach to Improve the Selection of Forecasting Algorithms in an Office Building in Different Contexts
PROGRESS IN ARTIFICIAL INTELLIGENCE, EPIA 2022(2022)
摘要
Energy management in buildings can be largely improved by considering adequate forecasting techniques to find load consumption patterns. While these forecasting techniques are relevant, decision making is needed to decide the forecasting technique that suits best each context, thus improving the accuracy of predictions. In this paper, two forecasting methods are used including artificial neural network and k-nearest neighbor. These algorithms are considered to predict the consumption of a building equipped with devices recording consumptions and sensors data. These forecasts are performed from five-to-five minutes and the forecasting technique decision is taken into account as an enhanced factor to improve the accuracy of predictions. This decision making is optimized with the support of the multi-armed bandit, the reinforcement learning algorithm that analyzes the best suitable method in each five minutes. Exploration alternatives are considered in trial and test studies as means to find the best suitable level of unexplored territory that results in higher accumulated rewards. In the case-study, four contexts have been considered to illustrate the application of the proposed methodology.
更多查看译文
关键词
Energy management, Learning, Load forecast
AI 理解论文
溯源树
样例
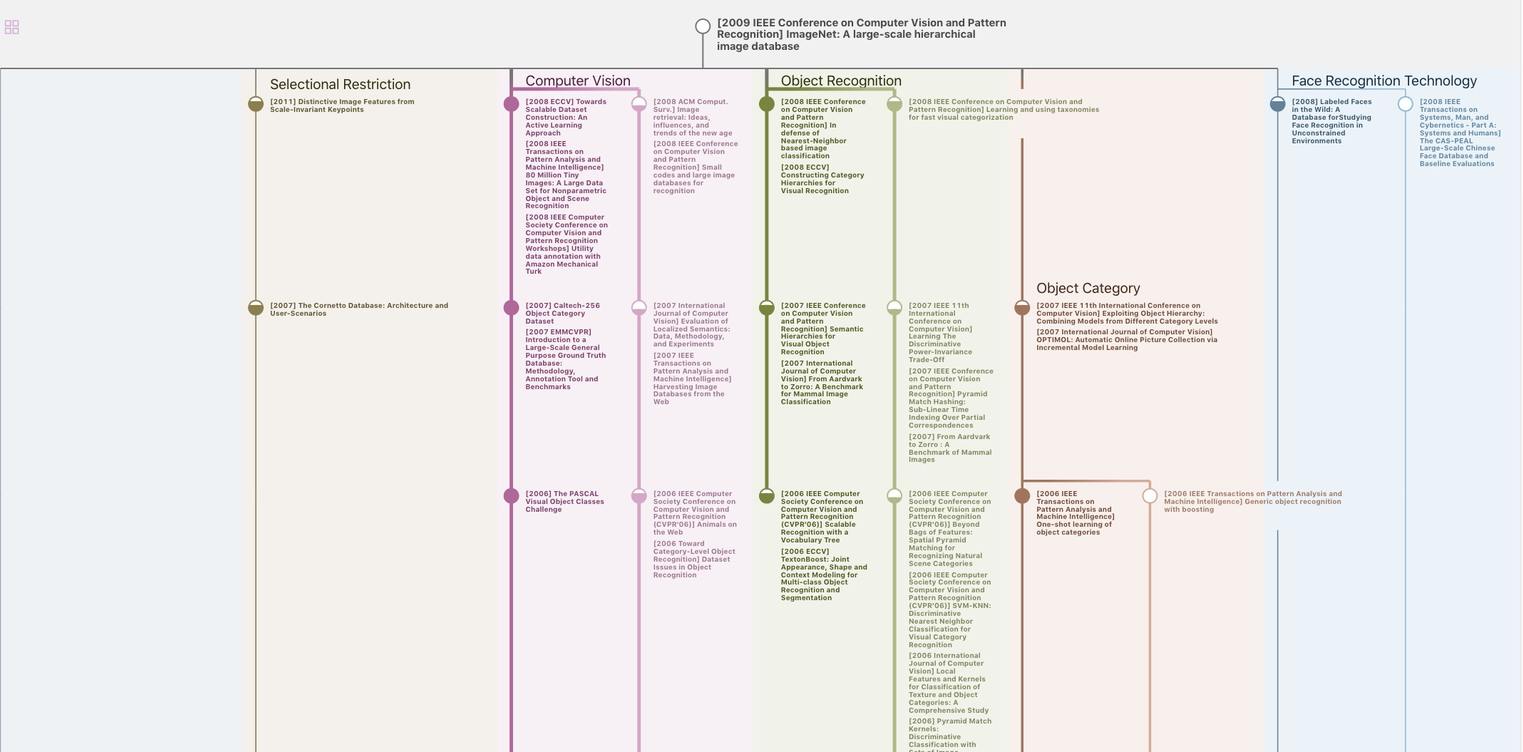
生成溯源树,研究论文发展脉络
Chat Paper
正在生成论文摘要