Federated Sparse Gaussian Processes
INTELLIGENT COMPUTING METHODOLOGIES, PT III(2022)
摘要
In this paper, we propose a federated sparse Gaussian process (FSGP) model, which combines the sparse Gaussian process (SGP) model with the framework of federated learning (FL). Sparsity enables the reduction in the time complexity of training a Gaussian process (GP) from O(N-3) to O(NM2) and the space complexity from O(N-2) to O(NM), where N is the number of training samples and M (M << N) the number of inducing points. Furthermore, FL aims at learning a shared model using data distributed on more than one client under the condition that local data on each client cannot be accessed by other clients. Therefore, our proposed FSGP model can not only deal with large datasets, but also preserve privacy. FSGPs are trained through variational inference and applied to regression problems. In experiments, we compare the performance of FSGPs with that of federated Gaussian processes (FGPs) and SGPs trained using the datasets consisting of all local data. The experimental results show that FSGPs are comparable with SGPs and outperform FGPs.
更多查看译文
关键词
Sparse Gaussian Processes, Variational inference, Federated learning, Preserve privacy
AI 理解论文
溯源树
样例
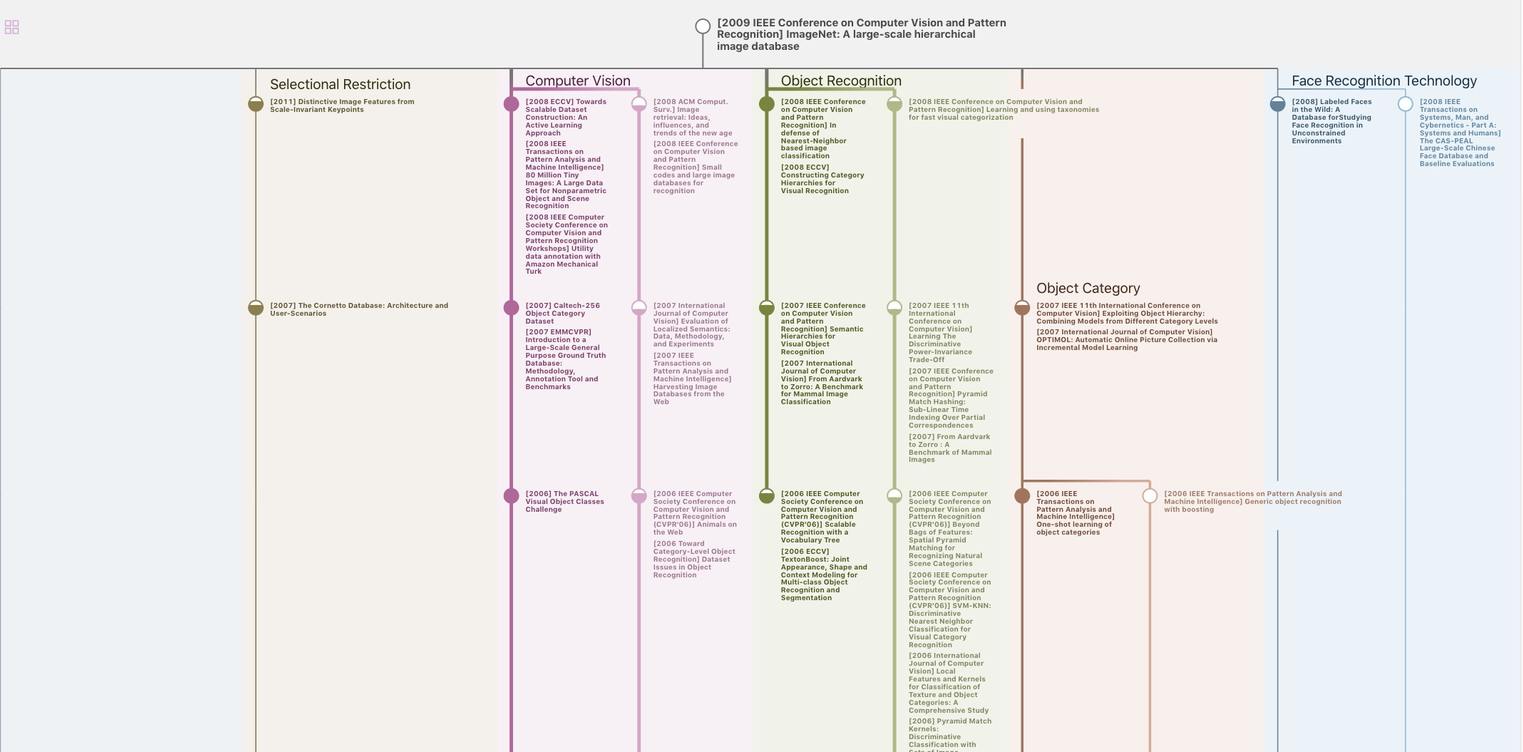
生成溯源树,研究论文发展脉络
Chat Paper
正在生成论文摘要