SpringNet: A Novel Deep Neural Network Architecture for Histopathological Image Analysis
PROCEEDINGS OF THE INTERNATIONAL CONFERENCE ON INNOVATIONS IN COMPUTING RESEARCH (ICR'22)(2022)
摘要
Reliable and capable tools are needed for segmentation and classification of nuclei in histopathology images to improve the workflow of histopathologists and lessen the burden of diagnosis. Analyzing histopathology images can be challenging for deep learning models due to the various sources of noise. We propose a pipeline for segmenting and classifying nuclei, trained on a combination of datasets to ensure robustness. This makes our pipeline capable of generalizing on image tiles with varying Hematoxylin & Eosin concentrations. For nuclei classification, we present a novel convolutional neural network classifier that demonstrate state-of-the-art classification performance on the Lizard dataset.
更多查看译文
关键词
Deep learning, Convolutional neural networks, Computational pathology, Nuclei segmentation, Nuclei classification, Medical imaging
AI 理解论文
溯源树
样例
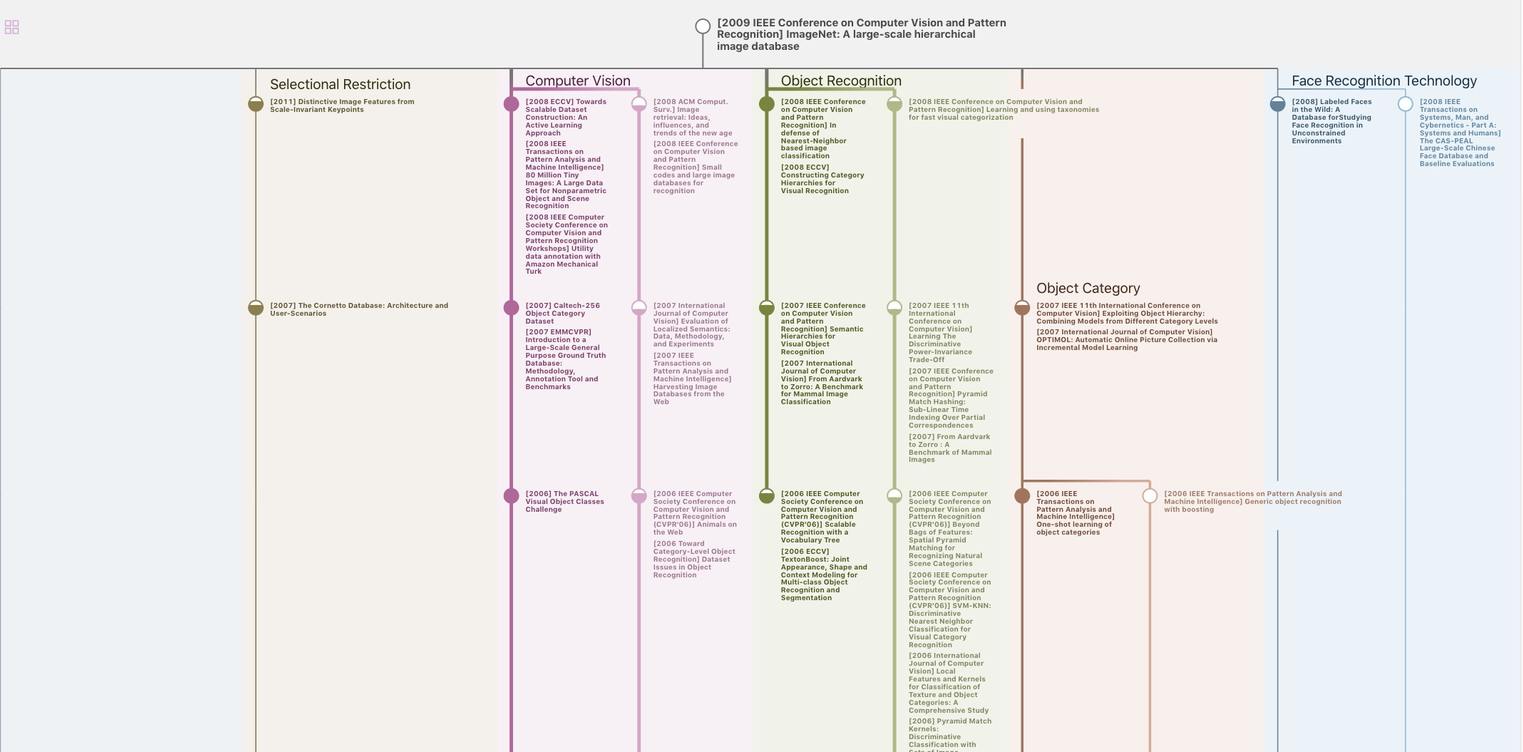
生成溯源树,研究论文发展脉络
Chat Paper
正在生成论文摘要