Marginal Fisher Analysis With Polynomial Matrix Function
IEEE ACCESS(2022)
摘要
Marginal fisher analysis (MFA) is a dimensionality reduction method based on a graph embedding framework. In contrast to traditional linear discriminant analysis (LDA), which requires the data to follow a Gaussian distribution, MFA is suitable for non-Gaussian data, and it has better pattern classification ability. However, MFA has the small-sample-size (SSS) problem. This paper aims to solve the small-sample-size problem while increasing the classification performance of MFA. Based on a matrix function dimensionality reduction framework, the criterion of the MFA method is reconstructed by using the polynomials matrix function transformation, and then a new MFA method is proposed, named PMFA (polynomial marginal fisher analysis). The major contributions of the proposed PMFA method are that it solves the small-sample-size problem of MFA, and it can enlarge the distance between marginal sample points of inter-class, so that it can get better pattern classification performance. Experiments on the public face datasets show that PMFA can get a better classification ability than MFA and its improved methods.
更多查看译文
关键词
Principal component analysis, Symmetric matrices, Face recognition, Manifold learning, Eigenvalues and eigenfunctions, Training data, Pattern classification, Dimensionality reduction, manifold learning, marginal fisher analysis, matrix function, the small-sample-size (SSS) problem
AI 理解论文
溯源树
样例
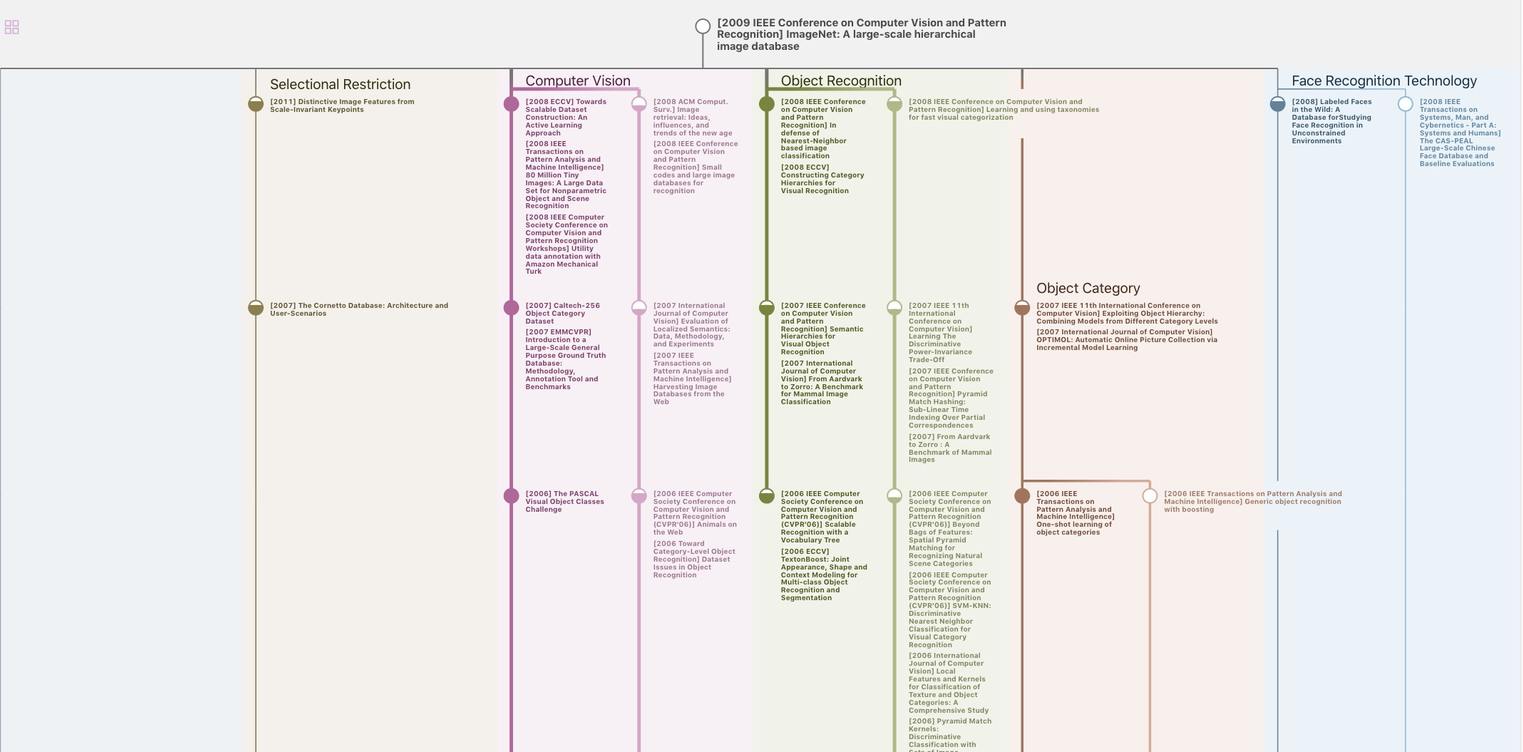
生成溯源树,研究论文发展脉络
Chat Paper
正在生成论文摘要