Wind turbine blade icing detection using a novel bidirectional gated recurrent unit with temporal pattern attention and improved coot optimization algorithm
MEASUREMENT SCIENCE AND TECHNOLOGY(2023)
摘要
Blade icing detection is significant for the safe operation of wind turbines and to reduce power generation losses. Traditional additional sensor methods for monitoring are limited due to the high cost and damage to the original mechanical structure. The deep learning model shows a good performance for icing detection. This paper proposes a temporal pattern attention-based bidirectional gated recurrent unit (BiGRU-TPA). This novel deep learning framework incorporates the TPA module into the BiGRU module to determine the relationship between multiple sensors at different time steps, extracting features from the raw sensor data for discrimination. Meanwhile, its hyperparameters are optimized using an improved coot optimization algorithm (ICOOT) to further enhance its recognition performance. To alleviate the severe imbalance in the dataset, adaptive synthesis and the sliding window upsampling method are imported to oversample and sliding window the icing state samples, which belong to the minority class. ICOOT-BiGRU-TPA illustrates its advantages compared with other state-of-the-art baseline methods, widely used optimization algorithms, and attention mechanisms applied to the real icing dataset. The ablation study and sensitivity analysis also demonstrate the performance of crucial components in the proposed model. Furthermore, its feasibility and practicality are demonstrated by real-time icing detection.
更多查看译文
关键词
temporal pattern attention, bidirectional gated recurrent unit, blade icing detection, wind turbine, multivariate time-series classification, improved coot optimization algorithm
AI 理解论文
溯源树
样例
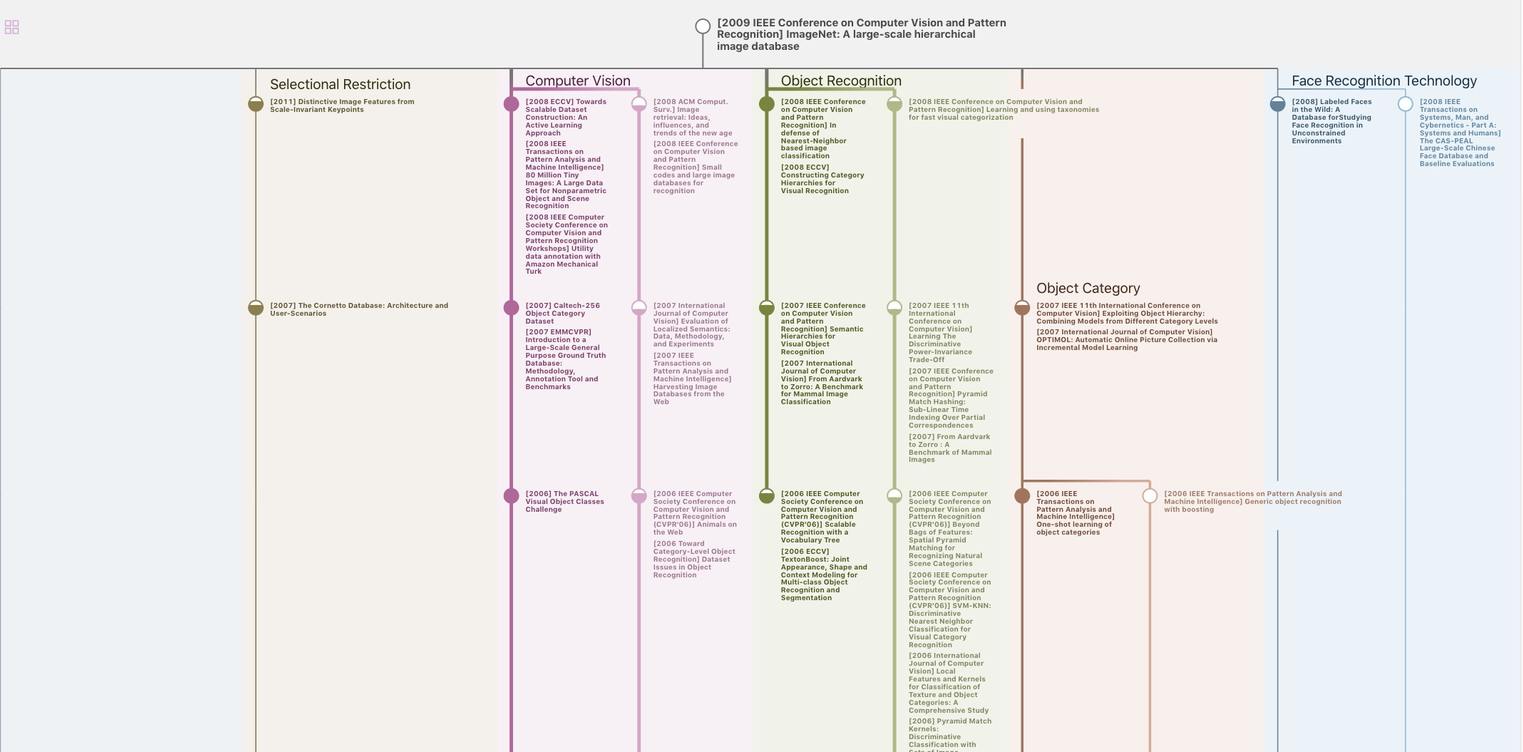
生成溯源树,研究论文发展脉络
Chat Paper
正在生成论文摘要