Graph Attention Network With Spatial-Temporal Clustering for Traffic Flow Forecasting in Intelligent Transportation System
IEEE TRANSACTIONS ON INTELLIGENT TRANSPORTATION SYSTEMS(2023)
摘要
With the development of the Internet of Things (IoT) and 5G technologies, IoT devices deployed on roads are able to collect a large amount of traffic data at any time. Road networks can be easily constructed into a graph structure with spatial-temporal features, and how to use these spatial-temporal features for dynamic traffic flow forecasting has become a heated issue. Although existing studies bring in the consideration of periodicity to deal with spatial-temporal sequence dependence, the similarity of time-varying relationships among cross-spatial nodes has not been well discussed. In this paper, we propose a Graph Attention Network with Spatial-Temporal Clustering (GAT-STC), which considers the so-called recent-aware features and periodic-aware features, to improve the Graph Neural Network (GNN)-based traffic flow forecasting in Intelligent Transportation System (ITS). Specifically, for the recent-aware feature extraction, a distance-based Graph Attention Network (GAT) is improved and constructed to better utilize the hidden features of neighbor nodes within a reliable distance during the recent time interval, thus can effectively capture the dynamic changes in spatial feature representation. For the periodic-aware feature extraction, a spatial-temporal clustering algorithm, in which both features in terms of nodes' current traffic states and similar trends in terms of their dynamic changes are taken into account, is developed and applied to achieve better learning efficiency. Experiments using three public traffic datasets demonstrate the higher accuracy and better efficiency of our proposed model for traffic flow forecasting, compared with five baseline methods in ITS.
更多查看译文
关键词
Forecasting, Feature extraction, Convolution, Predictive models, Task analysis, Internet of Things, Data models, Graph neural network, graph attention network, temporal convolutional network, spatial-temporal clustering, traffic flow forecasting, intelligent transportation system
AI 理解论文
溯源树
样例
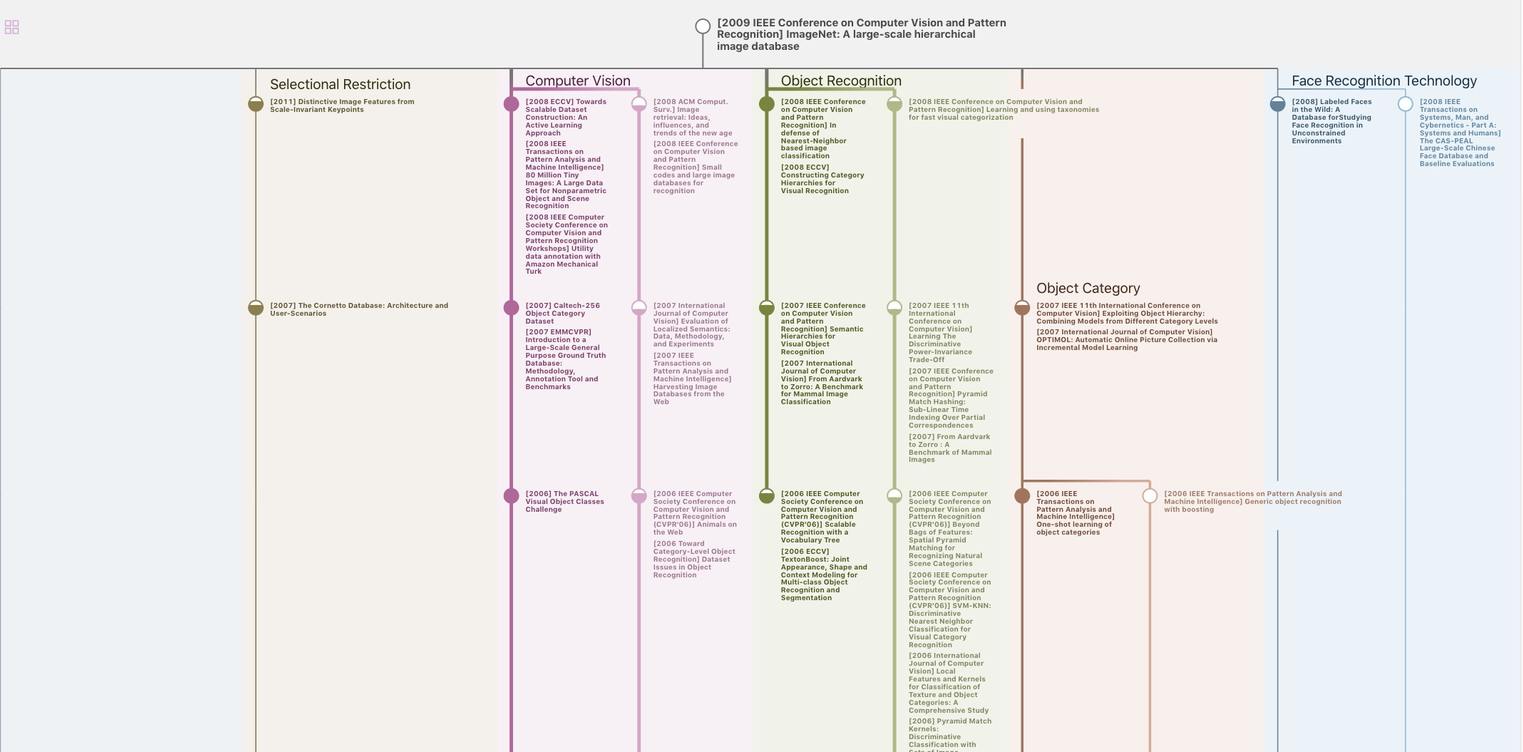
生成溯源树,研究论文发展脉络
Chat Paper
正在生成论文摘要