Multi-tracer PET Imaging Using Deep Learning: Applications in Patients with High-Grade Gliomas
PREDICTIVE INTELLIGENCE IN MEDICINE (PRIME 2022)(2022)
摘要
One of the great strengths of positron emission tomography (PET) is its ability to quantitatively image a wide array of molecular targets for disease evaluation. However, multi-tracer PET imaging is hindered because current technology permits only one PET tracer to be imaged at a time. The aim of this study was to develop a deep learning system that uses a PET image obtained with one tracer to predict a synthetic PET image of a different tracer without having to actually inject the second tracer. Deep neural networks were designed to generate synthetic 3'-deoxy-3'-[F-18]-fluorothymidine (F-18-FLT) PET images from 3,4-dihydroxy-6-[F-18]-fluoro-L-phenylalanine (F-18-FDOPA) PET and magnetic resonance imaging scans of nineteen patients with glioblastoma. Here, we show that the proposed image synthesis method closely predicts the ground truth F-18-FLT PET images (MAE = 0.024 +/- 0.004, SSIM = 0.832 +/- 0.035, PSNR = 27.521 +/- 1.606). Moreover, a blinded image evaluation by three nuclear medicine physicians determined that the synthetic images were rated significantly better on spatial resolution (p < 0.015), image noise (p < 0.001) and overall image quality (p < 0.0001) than the true PET images. This study offers a new strategy for multi-tracer PET imaging using machine learning and reduces radiation dose to the patient by at least 50%.
更多查看译文
关键词
Deep learning, Multi-tracer PET, Synthetic images
AI 理解论文
溯源树
样例
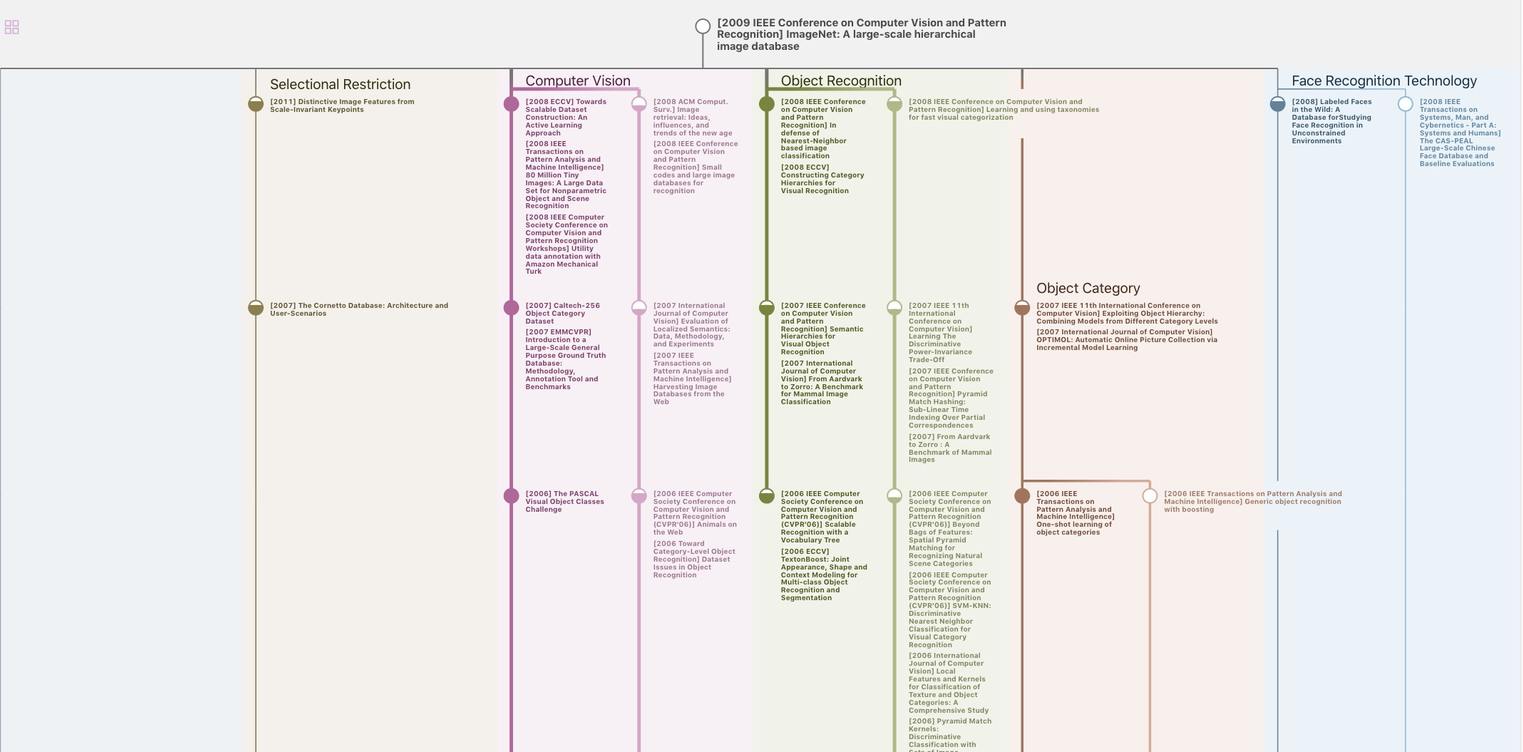
生成溯源树,研究论文发展脉络
Chat Paper
正在生成论文摘要