Fast Acquisition and Accurate Vital Sign Estimation with Deep Learning-Aided Weighted Scheme Using FMCW Radar
2022 IEEE 95TH VEHICULAR TECHNOLOGY CONFERENCE (VTC2022-SPRING)(2022)
摘要
Remote vital sign monitoring systems have attracted attention from researchers as a non-contact solution for the detection of health issues. This assumes significance during the epidemic outbreak, where infections can be transmitted through contact. This paper proposes a deep learning (DL)-aided weighted scheme, where observations are fused, for implementing a vital sign system for fast acquisition and accurate estimation by considering spatial correlations. The specially designed weighted technique properly fuses spatial features to reduce the required size of the observation signal, thereby accomplishing the goal of fast acquisition. Moreover, a convolutional neural network (CNN) is employed to extract hidden information for accurate vital sign detection by performing two-dimensional convolution operations, which effectively utilizes spatial diversity to improve detection performance. Experimental results show that the proposed data-fusion-based scheme achieves satisfactory performance with limited observations for fast acquisition. Furthermore, its performance is comparable to that of conventional contact equipment; the absolute error of 90% breathing measurements is less than 3 respirations per minute (rpm), and the absolute error of 75% heartbeat measurements is less than 3 beats per minute (bpm), thereby confirming the potential of the proposed scheme.
更多查看译文
关键词
Vital signs, remote monitoring, FMCW radar, data fusion, deep learning, convolutional neural network
AI 理解论文
溯源树
样例
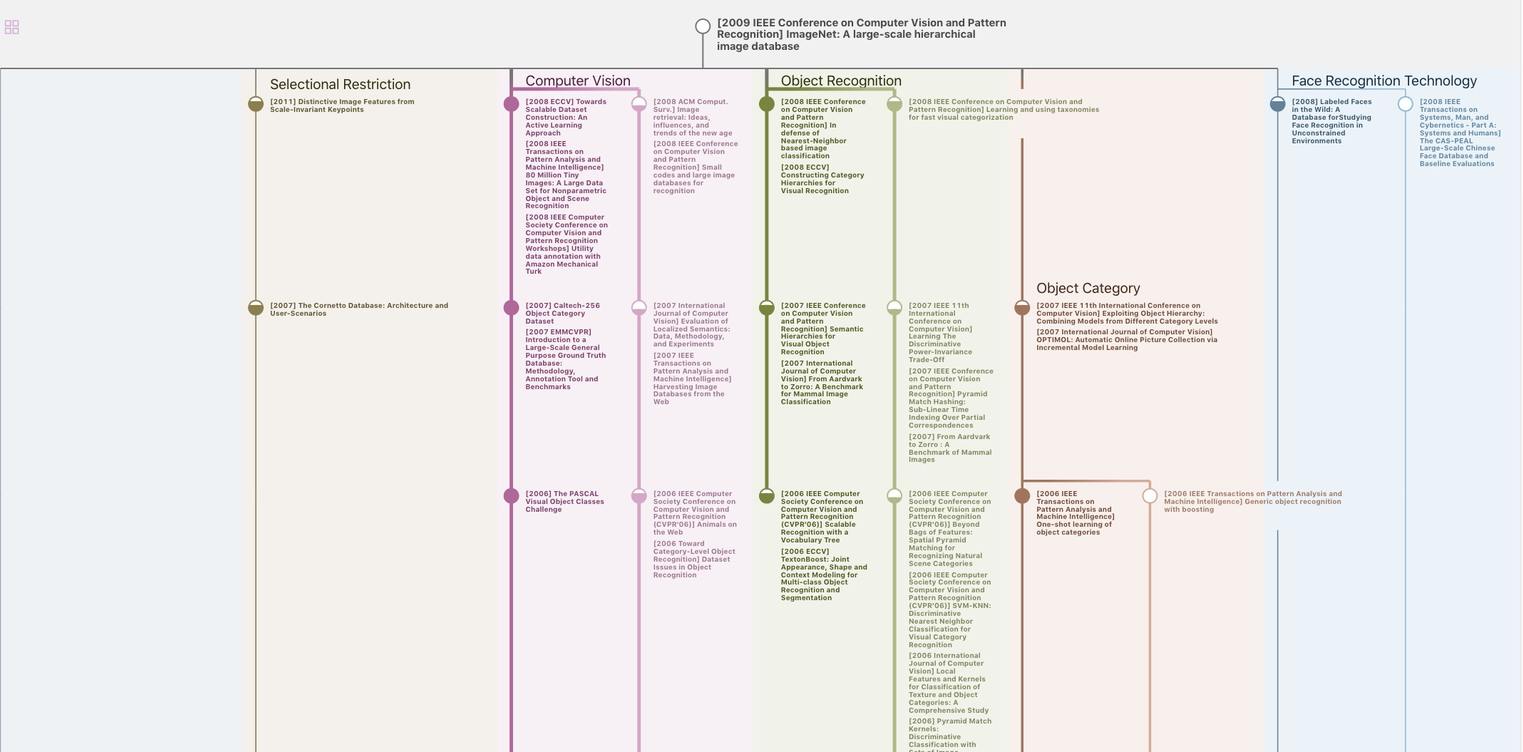
生成溯源树,研究论文发展脉络
Chat Paper
正在生成论文摘要