Investigating the Vulnerability of Federated Learning-Based Diabetic Retinopathy Grade Classification to Gradient Inversion Attacks
OPHTHALMIC MEDICAL IMAGE ANALYSIS, OMIA 2022(2022)
摘要
Diabetic retinopathy (DR) is a serious vision-threatening condition associated with diabetes and is the leading cause of visual impairment for working-age adults worldwide. Smartphone-based fundus imaging (SBFI) has the potential to be combined with machine learning-based DR screening procedures to simultaneously improve global health equity and the prognosis of DR by increasing patient accessibility to low-cost DR screening services. Federated learning is a promising method to train machine learning models for DR grade classification using large amounts of SBFI data, which can protect the privacy of sensitive patient data at the same time. However, gradient inversion attacks have been shown to be able to reconstruct private data using the model parameter gradient information transmitted during federated learning updates. The purpose of this paper is to investigate the privacy threat that gradient inversion attacks pose for reconstructing identifiable retinal fundus images during federated learning-based DR grade classification training. Specifically, a novel metric called "Segmentation Matching Score" (SMS) is proposed to quantify clinically relevant features present in fundus images reconstructed during a gradient inversion attack that could be exploited for patient identification information. Experimental results based on the FGADR dataset demonstrate that reconstructed images could be correctly matched to their corresponding source images using the SMS metric with a top-1 accuracy of 72.0%. These findings indicate that gradient inversion attacks pose a significant threat for federated learning-based DR grade classification models and warrant further investigation into viable defense strategies.
更多查看译文
关键词
Diabetic retinopathy, Gradient inversion attack, Federated learning
AI 理解论文
溯源树
样例
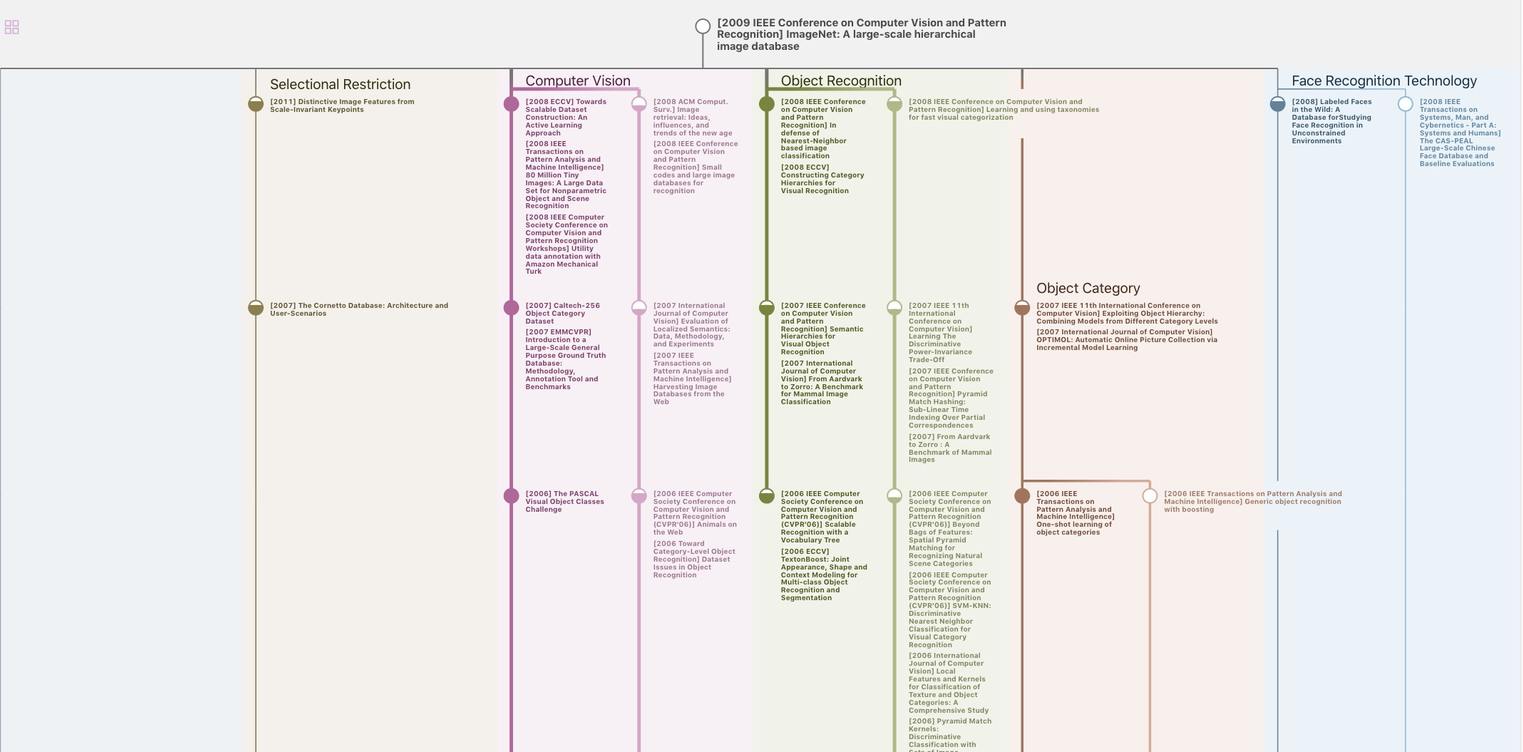
生成溯源树,研究论文发展脉络
Chat Paper
正在生成论文摘要