SelfMix: A Self-adaptive Data Augmentation Method for Lesion Segmentation
MEDICAL IMAGE COMPUTING AND COMPUTER ASSISTED INTERVENTION, MICCAI 2022, PT IV(2022)
摘要
Deep learning-based methods have obtained promising results in various organ segmentation tasks, due to their effectiveness in learning feature representation. However, accurate segmentation of lesions can still be challenging due to 1) the lesions provide less information than normal organs; 2) the available number of labeled lesions is more limited than normal organs; 3) the morphology, shape, and size of lesions are more diverse than normal organs. To increase the number of lesion samples and further boost the performance of various lesion segmentation, in this paper, we propose a simple but effective lesion-aware data augmentation method called Self-adaptive Data Augmentation (SelfMix). Compared with existing data augmentation methods, such as Mixup, CutMix, and CarveMix, our proposed SelfMix have three-fold advances: 1) Solving the challenges that the generated tumor images are facing the problem of distortion by absorbing both tumor and non-tumor information; 2) SelfMix is tumor-aware, which can adaptively adjust the fusing weights of each lesion voxels based on the geometry and size information from the tumor itself; 3) SelfMix is the first one that notices non-tumor information. To evaluate the proposed data augmentation method, experiments were performed on two public lesion segmentation datasets. The results show that our method improves the lesion segmentation accuracy compared with other data augmentation approaches.
更多查看译文
关键词
Lesions segmentation, Data augmentation, Tumor-aware
AI 理解论文
溯源树
样例
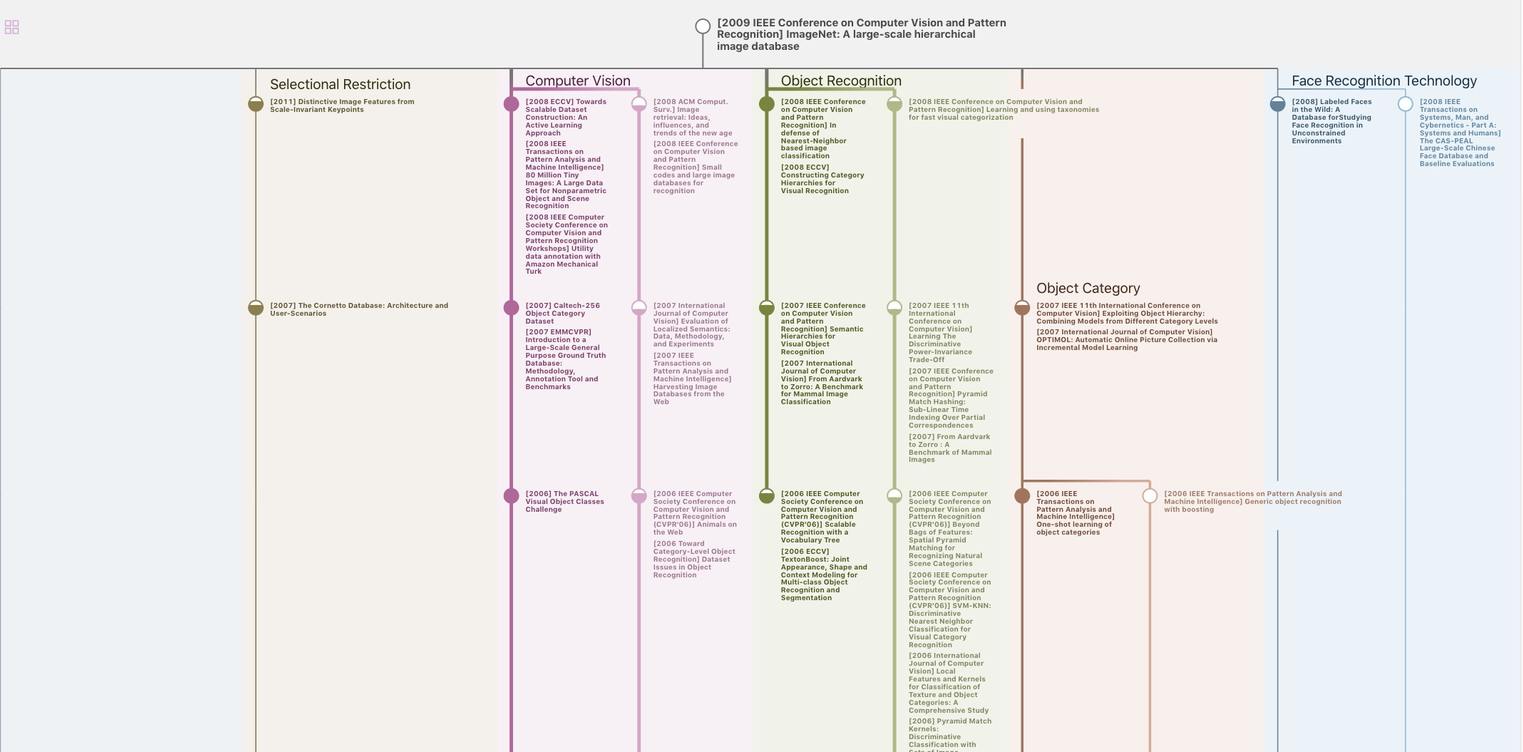
生成溯源树,研究论文发展脉络
Chat Paper
正在生成论文摘要