BoxPolyp: Boost Generalized Polyp Segmentation Using Extra Coarse Bounding Box Annotations
MEDICAL IMAGE COMPUTING AND COMPUTER ASSISTED INTERVENTION, MICCAI 2022, PT III(2022)
摘要
Accurate polyp segmentation is of great importance for colorectal cancer diagnosis and treatment. However, due to the high cost of producing accurate mask annotations, existing polyp segmentation methods suffer from severe data shortage and impaired model generalization. Reversely, coarse polyp bounding box annotations are more accessible. Thus, in this paper, we propose a boosted BoxPolyp model to make full use of both accurate mask and extra coarse box annotations. In practice, box annotations are applied to alleviate the over-fitting issue of previous polyp segmentation models, which generate fine-grained polyp area through the iterative boosted segmentation model. To achieve this goal, a fusion filter sampling (FFS) module is firstly proposed to generate pixel-wise pseudo labels from box annotations with less noise, leading to significant performance improvements. Besides, considering the appearance consistency of the same polyp, an image consistency (IC) loss is designed. Such IC loss explicitly narrows the distance between features extracted by two different networks, which improves the robustness of the model. Note that our BoxPolyp is a plug-and-play model, which can be merged into any appealing backbone. Quantitative and qualitative experimental results on five challenging benchmarks confirm that our proposed model outperforms previous state-of-the-art methods by a large margin.
更多查看译文
关键词
Polyp segmentation, Colonoscopy, Colorectal cancer
AI 理解论文
溯源树
样例
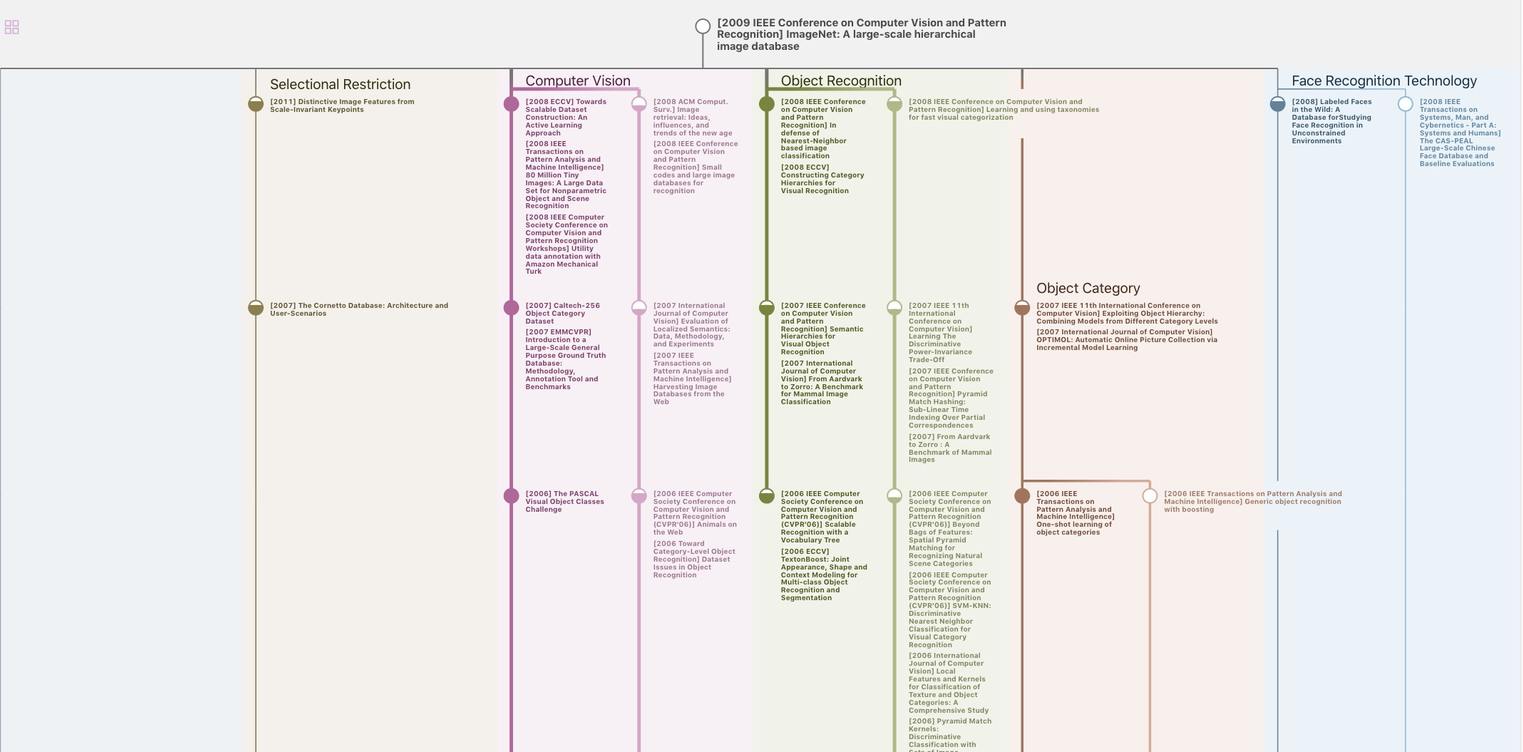
生成溯源树,研究论文发展脉络
Chat Paper
正在生成论文摘要