Few-shot Medical Image Segmentation Regularized with Self-reference and Contrastive Learning
MEDICAL IMAGE COMPUTING AND COMPUTER ASSISTED INTERVENTION, MICCAI 2022, PT IV(2022)
摘要
Despite the great progress made by deep convolutional neural networks (CNN) in medical image segmentation, they typically require a large amount of expert-level accurate, densely-annotated images for training and are difficult to generalize to unseen object categories. Few-shot learning has thus been proposed to address the challenges by learning to transfer knowledge from a few annotated support examples. In this paper, we propose a new prototype-based few-shot segmentation method. Unlike previous works, where query features are compared with the learned support prototypes to generate segmentation over the query images, we propose a self-reference regularization where we further compare support features with the learned support prototypes to generate segmentation over the support images. By this, we argue for that the learned support prototypes should be representative for each semantic class and meanwhile discriminative for different classes, not only for query images but also for support images. We additionally introduce contrastive learning to impose intra-class cohesion and inter-class separation between support and query features. Results from experiments conducted on two publicly available datasets demonstrated the superior performance of the proposed method over the state-of-the-art (SOTA).
更多查看译文
关键词
Few-shot, Medical image segmentation, Self-reference, Contrastive learning
AI 理解论文
溯源树
样例
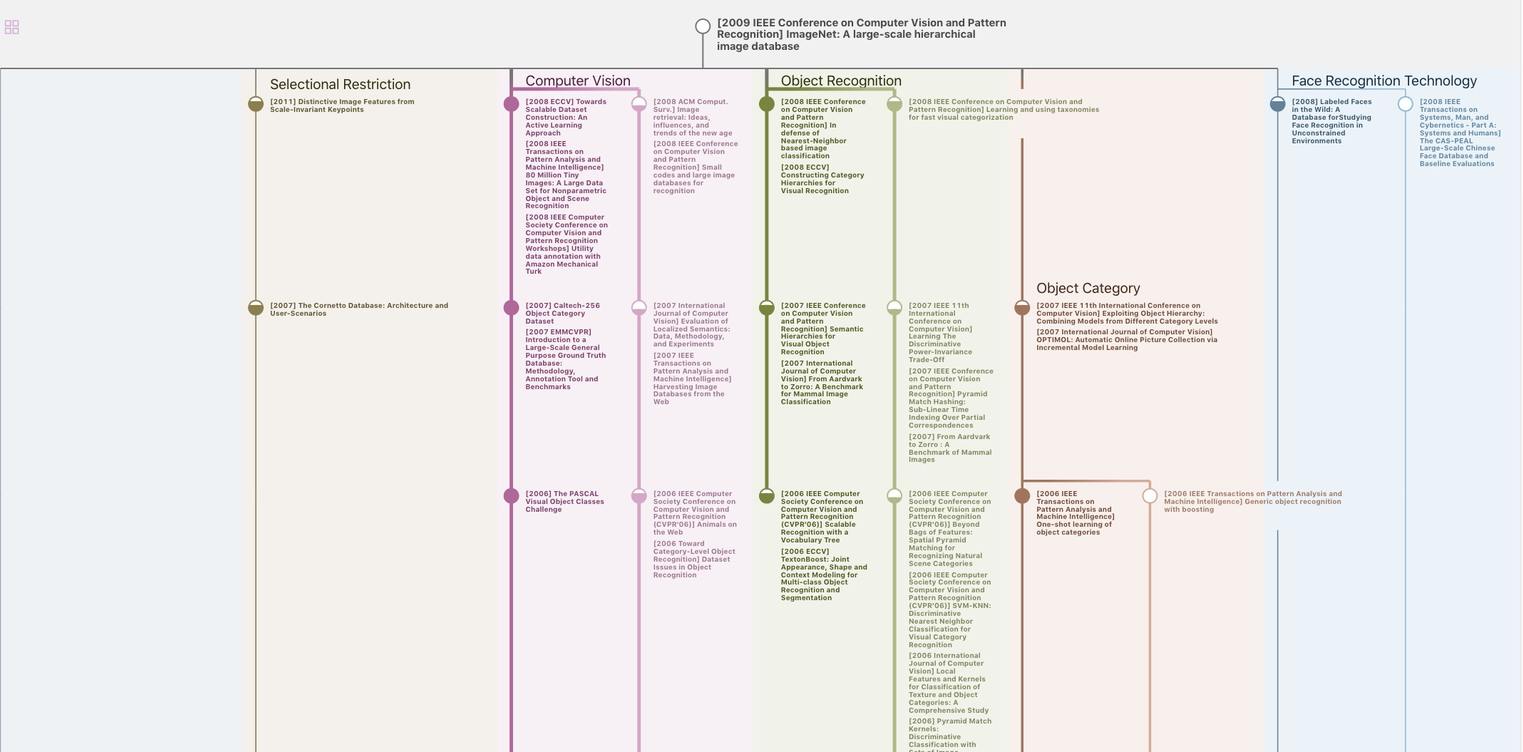
生成溯源树,研究论文发展脉络
Chat Paper
正在生成论文摘要