MLAN: Multi-Level Attention Network
IEEE ACCESS(2022)
摘要
In this paper, we proposed a "Multi-Level Attention Network" (MLAN), which defines a multi-level structure, including layer, block, and group levels to get hierarchical attention and combines corresponding residual information for better feature extraction. We also constructed a shared mask attention module (SMA) which can significantly reduce the number of parameters compared with conventional attention methods. Based on the MLAN and SMA, we further investigated a variety of information fusion modules for better feature fusion at different levels. We conducted classification task experiments based on the ResNet backbone with different depths, and the experimental results show that our method has a significant performance improvement over the backbone on CIFAR10 and CIFAR100 datasets. Meanwhile, compared with the mainstream attention methods, our MLAN performs better with higher accuracy as well as less parameters and computation complexity. We also visualized some intermediate feature maps and explained why our MLAN performs well.
更多查看译文
关键词
Feature extraction, Visualization, Time-frequency analysis, Neural networks, Convolutional neural networks, Computational complexity, Multi-level structure, shared mask attention, hierarchical attention aggregation, information fusion
AI 理解论文
溯源树
样例
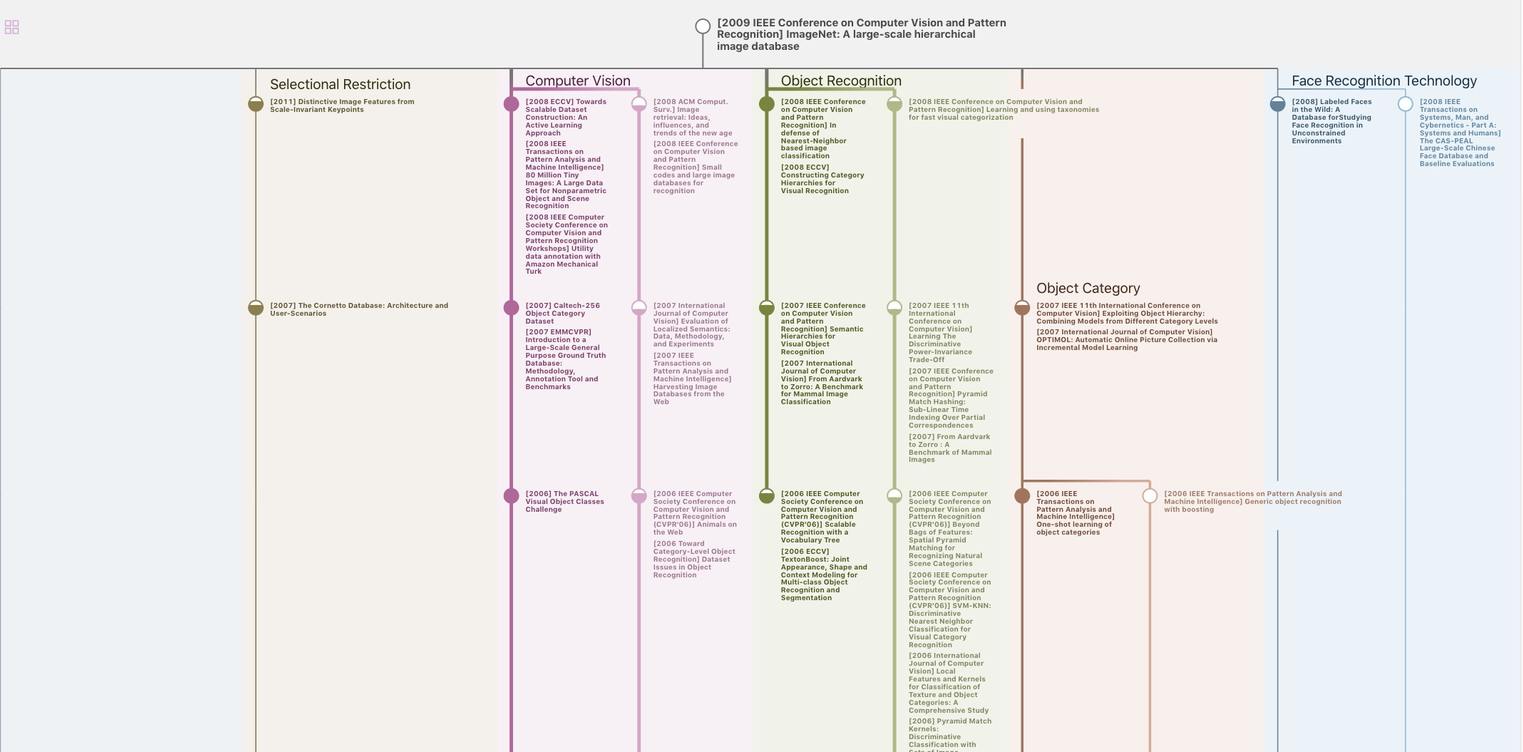
生成溯源树,研究论文发展脉络
Chat Paper
正在生成论文摘要