Incentive and Knowledge Distillation Based Federated Learning for Cross-Silo Applications
IEEE INFOCOM 2022 - IEEE CONFERENCE ON COMPUTER COMMUNICATIONS WORKSHOPS (INFOCOM WKSHPS)(2022)
摘要
Big data are usually characterized by heterogeneity in real-world cross-silo applications, such as healthcare, finance, and smart cities, leaving federated learning a big challenge. Further, many existing federated learning schemes fail to fully consider the diverse willingness and contributions of data providers in participation. In this paper, to address these challenges, we are motivated to propose an incentive and knowledge distillation based federated learning scheme for cross-silo applications. Specifically, we first develop a new federated learning framework, to support cooperative learning among diverse heterogeneous client models. Second, we devise an incentive mechanism, which not only stimulates workers to provide more high-quality data, but also improves clients' enthusiasm for participating in federated learning. Third, a novel knowledge distillation algorithm is designed to deal with data heterogeneity. Extensive experiments on MNIST/FEMNIST and CIFAR10/100 datasets with both IID and Non-IID settings, demonstrate the high effectiveness of the proposed scheme, compared with state-of-the-art studies.
更多查看译文
关键词
Artificial intelligence, federated learning, knowledge distillation, incentive mechanism, data privacy
AI 理解论文
溯源树
样例
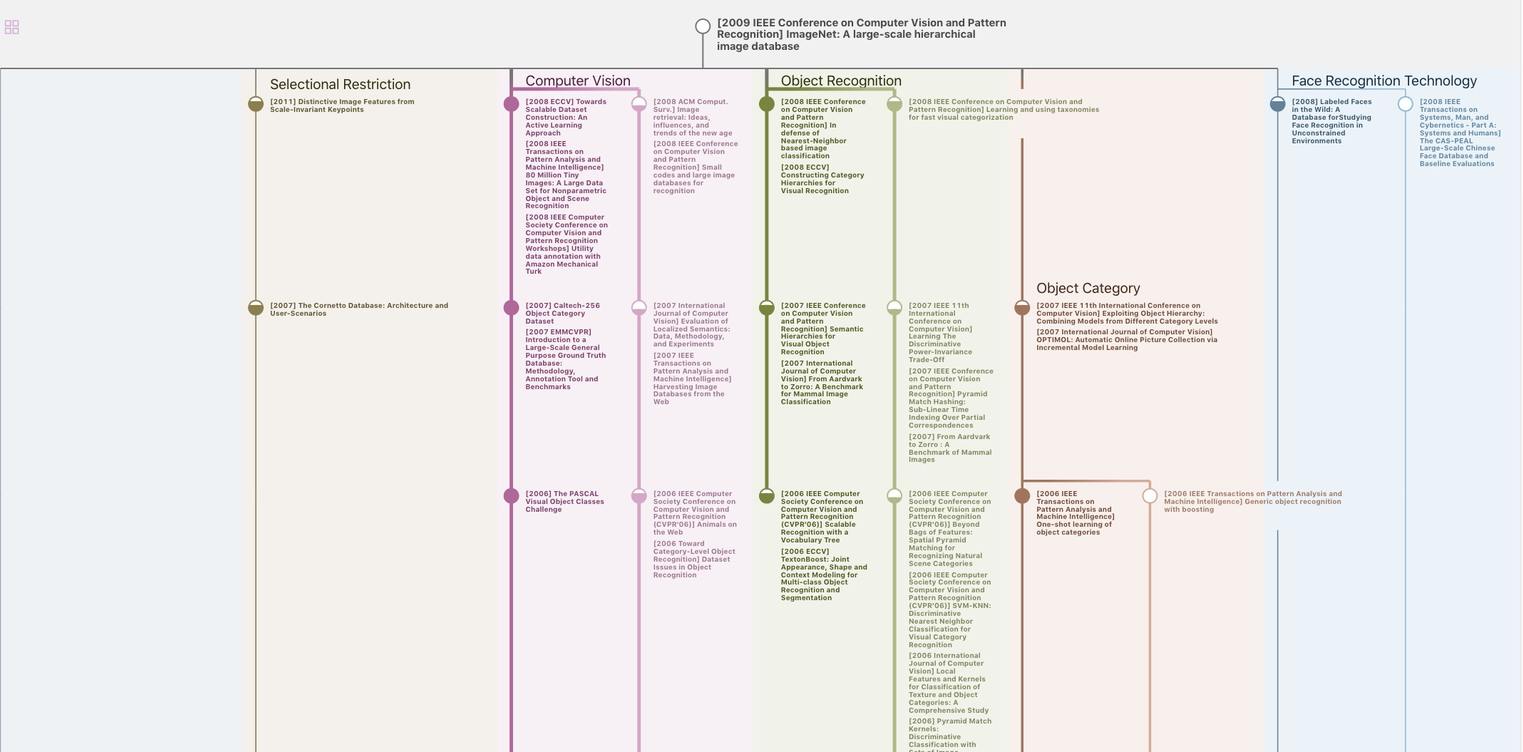
生成溯源树,研究论文发展脉络
Chat Paper
正在生成论文摘要