Edge Intelligence-based Privacy Protection Framework for IoT-based Smart Healthcare Systems
IEEE INFOCOM 2022 - IEEE Conference on Computer Communications Workshops (INFOCOM WKSHPS)(2022)
摘要
Federated Learning (FL) mechanisms determine the implications of sensitive data for constructing on-device Machine Learning (ML) to achieve personalisation in a smart application network, for example, sharing critical information of the smart healthcare industry over the Internet of Things (IoT) systems. The main function of centralised FL can be combined with Edge Intelligence (EI) for processing before final aggregation to reduce data manipulation and privacy hazard. However, executing EI in an Edge Computing (EC) layer also poses privacy risks to clients. Differential Privacy (DP) offers a viable solution by adding artificial noise to a parameter before aggregation. This paper introduces a Federated Edge Aggregator (FEA) framework with DP for safeguarding the high-tech healthcare industry using IoT systems. An iteration-based converged Convolutional Neural Network (CNN) model at Edge Layer (EL) is developed to perform EI to balance $\mathrm{FL}^{\prime}$ s privacy preservation and model performance over an IoT network. The results demonstrated a 90% accuracy performance after specific iterations, better than those of other baseline approaches with accuracy levels of approximately 80% with the same epsilon value of 4. Also, this framework is faster and more successfully meets the privacy preservation paradigm.
更多查看译文
关键词
Internet of Things,Federated Learning,Edge Intelligence,Privacy-preserving,Smart Healthcare System
AI 理解论文
溯源树
样例
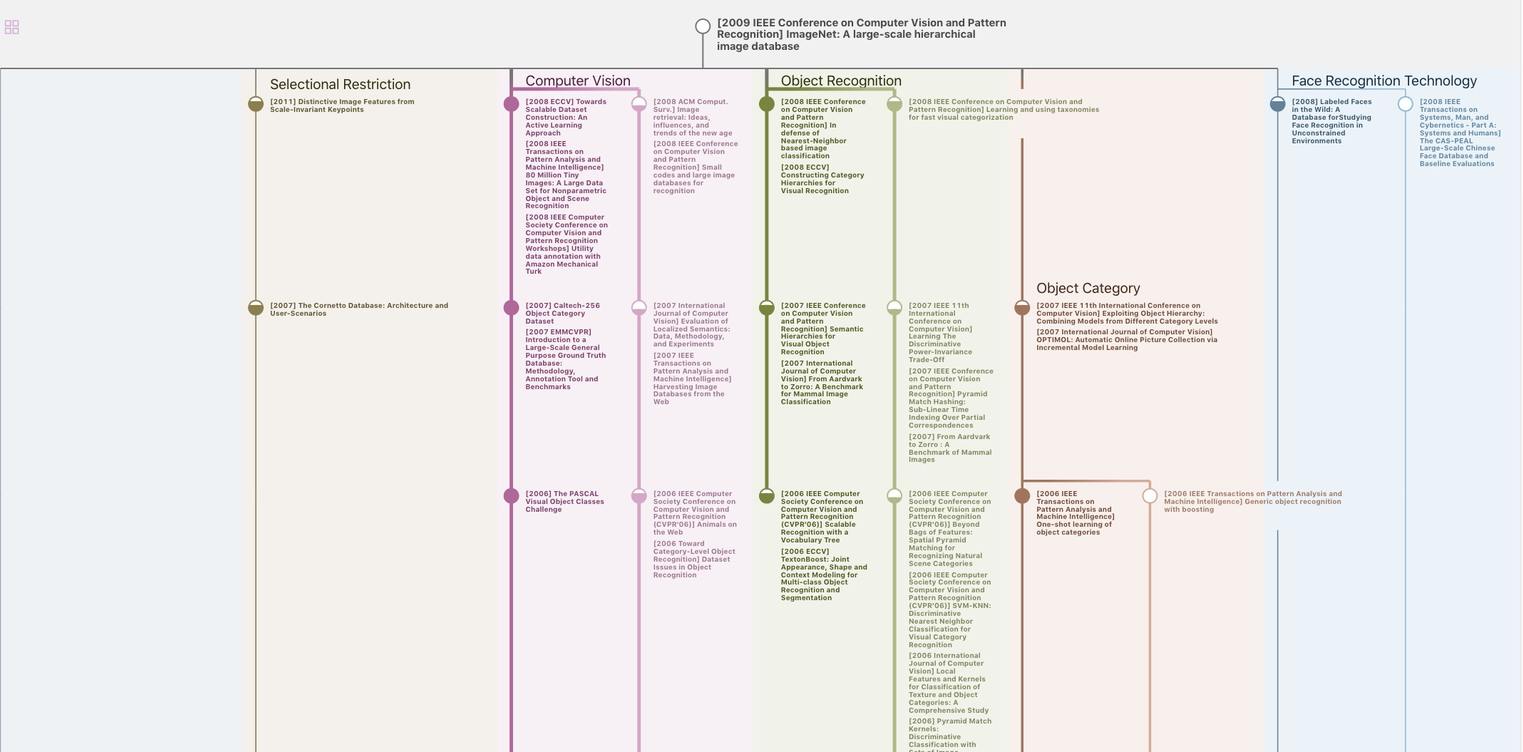
生成溯源树,研究论文发展脉络
Chat Paper
正在生成论文摘要