Image Inpainting Based Multi-scale Gated Convolution and Attention
ARTIFICIAL NEURAL NETWORKS AND MACHINE LEARNING - ICANN 2022, PT II(2022)
摘要
For image inpainting tasks, details and semantics are very important. Most methods based deep learning will make the result lack structure and texture features or even lose rationality. Additionally, many algorithms are step-by-step inpainting algorithms, which complicates the whole process. To avoid these, following the previous work, we propose an end-to-end inpainting model based on a multi-scale residual convolutional network, using MSGC to replace the conventional convolutional layer in encoder. This method combines multi-scale information and expands the receptive field, which can capture long-term information. In addition, we exploit SAB, a new scale attention block to capture important features from previous scale. At the same time, for getting richer detailed information, various loss functions are used to constrain the model during the training process. The test results of CelebA-HQ and Paris street view show that our proposed method is superior to other classic image inpainting algorithms.
更多查看译文
关键词
Image inpainting, Gated convolution, Multi-scale connection, Generative adversarial network
AI 理解论文
溯源树
样例
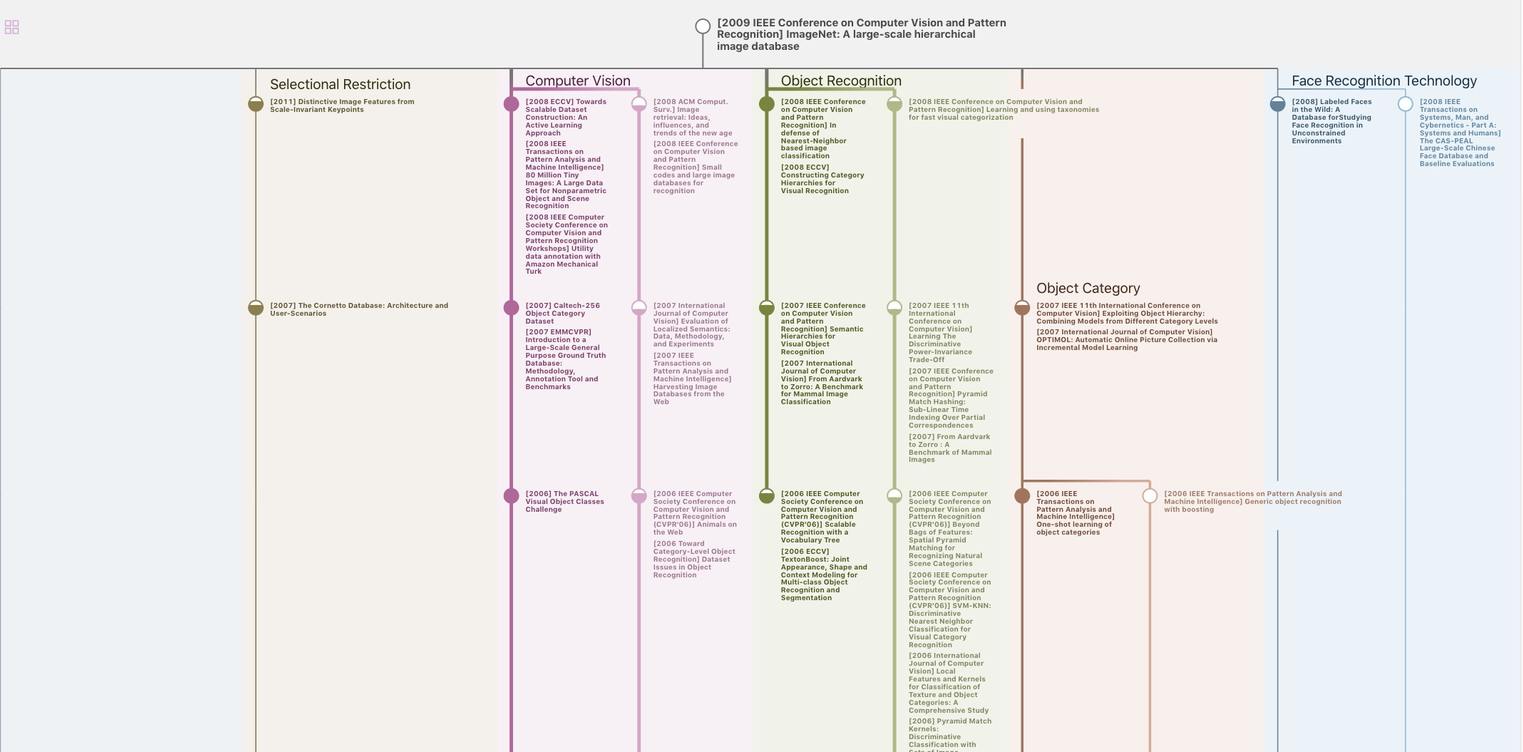
生成溯源树,研究论文发展脉络
Chat Paper
正在生成论文摘要