Phenotype Anomaly Detection for Biological Dynamics Data Using a Deep Generative Model
ARTIFICIAL NEURAL NETWORKS AND MACHINE LEARNING - ICANN 2022, PT II(2022)
摘要
Unsupervised anomaly detection is applied to biological dynamics data using a deep generative model. Phenotype anomaly detection for gene function identification has been successfully used in the reverse genetics, where the anomaly is conventionally characterized by a large number of pre-defined handcrafted features. The latest database of three-dimensional cell division data for the early development process of a model animal Caenorhabditis elegans (C. elegans) enables the present data-driven approach. A variational auto-encoder (VAE) was trained by 59 wild type (WT) data to acquire the normal individual features, and used to detect phenotypic anomalies in individuals resulting from selective RNA interference (RNAi) gene knockdown. Morphological anomalies were detected using the reconstruction error, whereas temporal anomalies were characterized by the time development trajectory in the VAE latent space. RNAi data corresponding to 97 essential genes on chromosome III in the two-cell period were studied by computational experiments, and several genes were suggested to be responsible for morphological or temporal anomalies, including genes with well-known functions in asymmetric cell division or cytoskeleton organization.
更多查看译文
关键词
Unsupervised anomaly detection, Variational auto-encoder, Biological time series, C. elegans, Gene function analysis
AI 理解论文
溯源树
样例
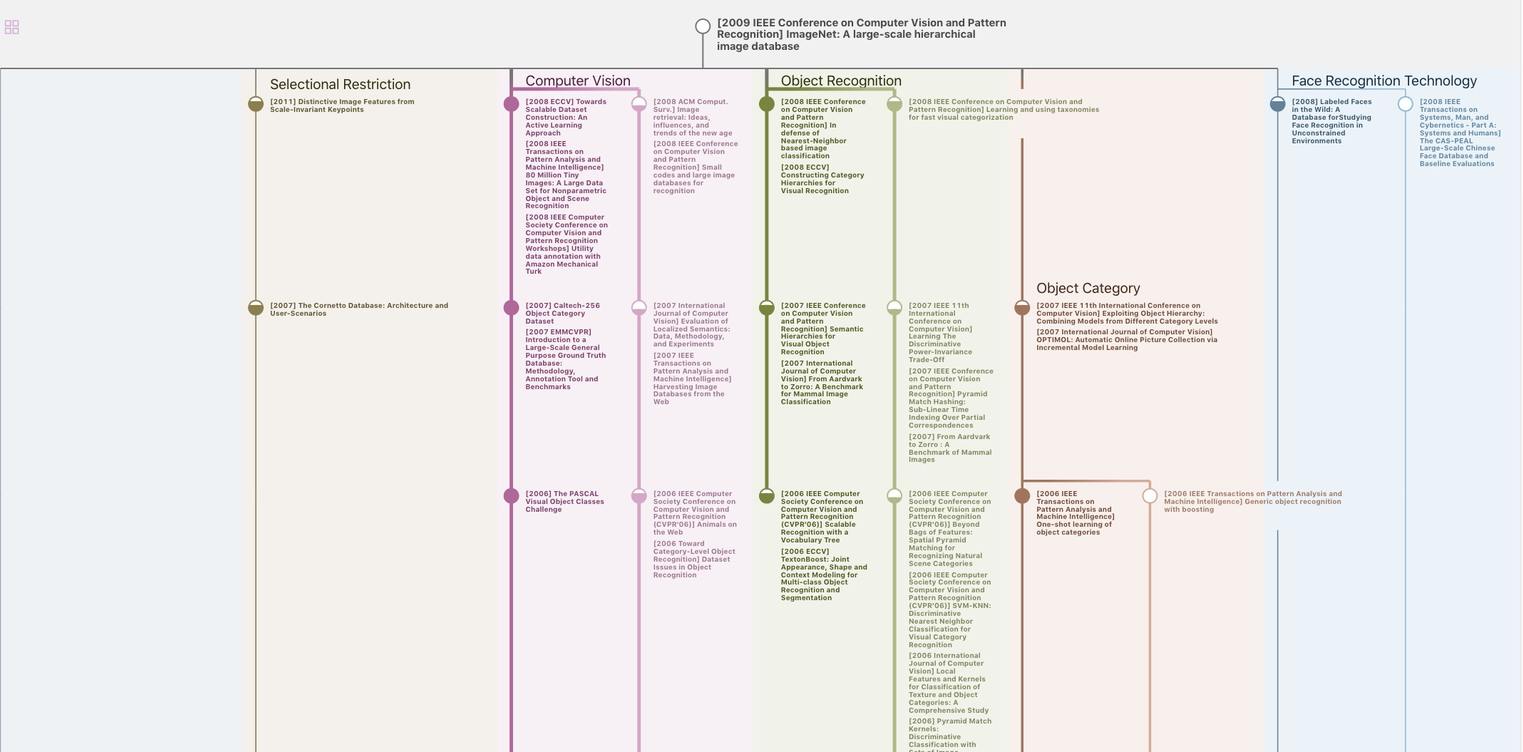
生成溯源树,研究论文发展脉络
Chat Paper
正在生成论文摘要