BUILDING SECTION INSTANCE SEGMENTATION WITH COMBINED CLASSICAL AND DEEP LEARNING METHODS
XXIV ISPRS CONGRESS IMAGING TODAY, FORESEEING TOMORROW, COMMISSION II(2022)
摘要
In big cities, the complexity of urban infrastructure is very high. In city centers, one construction can consist of several building sections of different heights or roof geometries. Most of the existing approaches detect those buildings as a single construction in the form of binary building segmentation maps or as one instance of object-oriented segmentation. However, reconstructing complex buildings consisting of several parts requires a higher level of detail. In this work, we present a methodology for individual building section instance segmentation on satellite imagery. We show that fully convolutional networks (FCNs) can tackle the issue much better than the state-of-the-art Mask-RCNN. A ground truth raster image with pixel value 1 for building sections and 2 for their touching borders was generated to train models on predicting both classes as a semantic output. The semantic outputs were then post-processed with the help of morphology and watershed labeling to generate segmentation on the instance level. The combination of a deep learning-based approach and a classical image processing algorithm allowed us to fulfill the segmentation task on the instance level and reach high-quality results with an mAP of up to 42 %.
更多查看译文
关键词
Building instance segmentation, high-resolution satellite imagery, WorldView-4, deep learning, watershed labeling, semantic segmentation
AI 理解论文
溯源树
样例
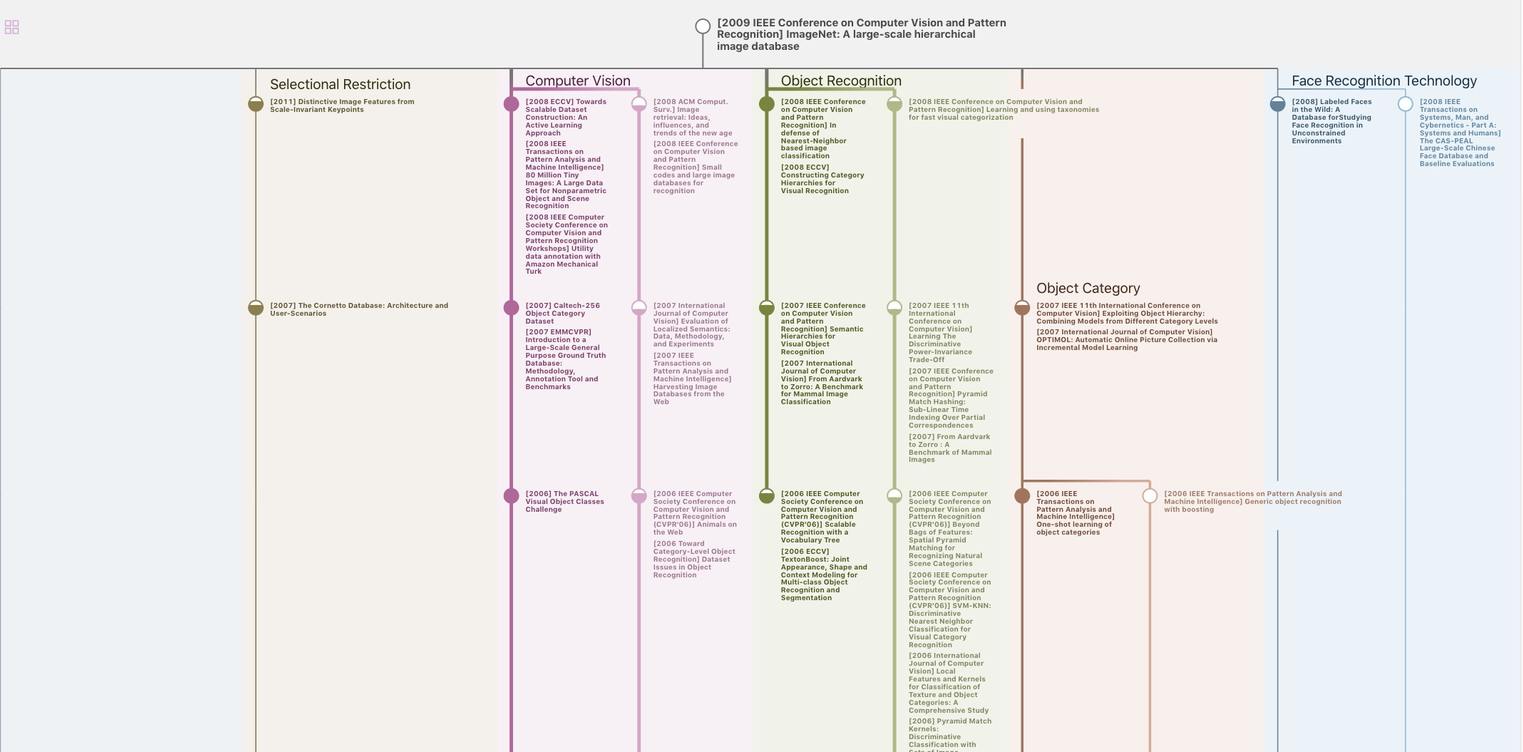
生成溯源树,研究论文发展脉络
Chat Paper
正在生成论文摘要