Discrepancy-Based Active Learning for Weakly Supervised Bleeding Segmentation in Wireless Capsule Endoscopy Images
MEDICAL IMAGE COMPUTING AND COMPUTER ASSISTED INTERVENTION, MICCAI 2022, PT VIII(2022)
摘要
Weakly supervised methods, such as class activation maps (CAM) based, have been applied to achieve bleeding segmentation with low annotation efforts in Wireless Capsule Endoscopy (WCE) images. However, the CAM labels tend to be extremely noisy, and there is an irreparable gap between CAM labels and ground truths for medical images. This paper proposes a new Discrepancy-basEd Active Learning (DEAL) approach to bridge the gap between CAMs and ground truths with a few annotations. Specifically, to liberate labor, we design a novel discrepancy decoder model and a CAMPUS (CAM, Pseudo-label and groUnd-truth Selection) criterion to replace the noisy CAMs with accurate model predictions and a few human labels. The discrepancy decoder model is trained with a unique scheme to generate standard, coarse and fine predictions. And the CAMPUS criterion is proposed to predict the gaps between CAMs and ground truths based on model divergence and CAM divergence. We evaluate our method on the WCE dataset and results show that our method outperforms the state-of-the-art active learning methods and reaches comparable performance to those trained with full annotated datasets with only 10% of the training data labeled. The source code is available at https://github.com/baifanxxx/DEAL.
更多查看译文
关键词
Active learning, Segmentation, WCE images
AI 理解论文
溯源树
样例
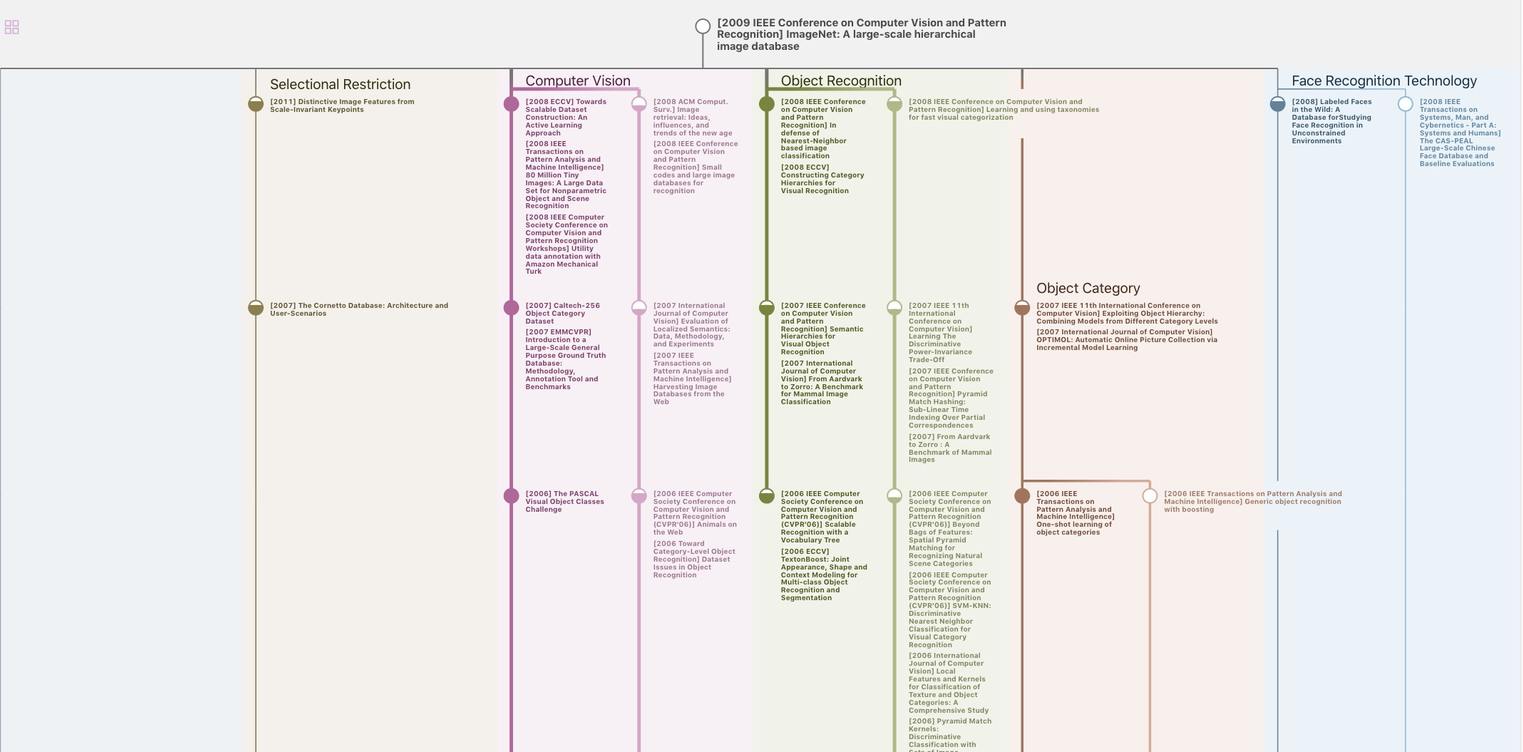
生成溯源树,研究论文发展脉络
Chat Paper
正在生成论文摘要