Large-scale Multi-objective Optimization for Water Quality in Chesapeake Bay Watershed
2022 IEEE CONGRESS ON EVOLUTIONARY COMPUTATION (CEC)(2022)
摘要
The careful selection of Best Management Practices (BMPs) to reduce loading, such as nitrogen, phosphorus, and sediments, can substantially improve the water quality of watersheds. This paper introduces the first implementation of a hybrid and customized evolutionary multi-objective (EMO) algorithm to improve the Chesapeake Bay Watershed's (CBW) water quality. To make the algorithm scalable, we inject a few solutions obtained using an integer programming algorithm (IPOPT) in the initial population of EMO. Also, a repair operator is applied to satisfy every equality constraint. Combining these approaches can find a set of non-dominated trade-off solutions from 1,012 variables (Tucker county in West Virginia) to a staggering 153,818 variable problem (the whole state of West Virginia). Furthermore, a preliminary analysis of obtained trade-off solutions finds interesting properties of BMP allocations, providing an optimistic picture of applying the proposed customized optimization algorithm in addressing other bigger states leading to the whole Chesapeake Bay watershed.
更多查看译文
关键词
Watershed Optimization, Large-scale Optimization, Multi-objective Optimization, Hybrid approach
AI 理解论文
溯源树
样例
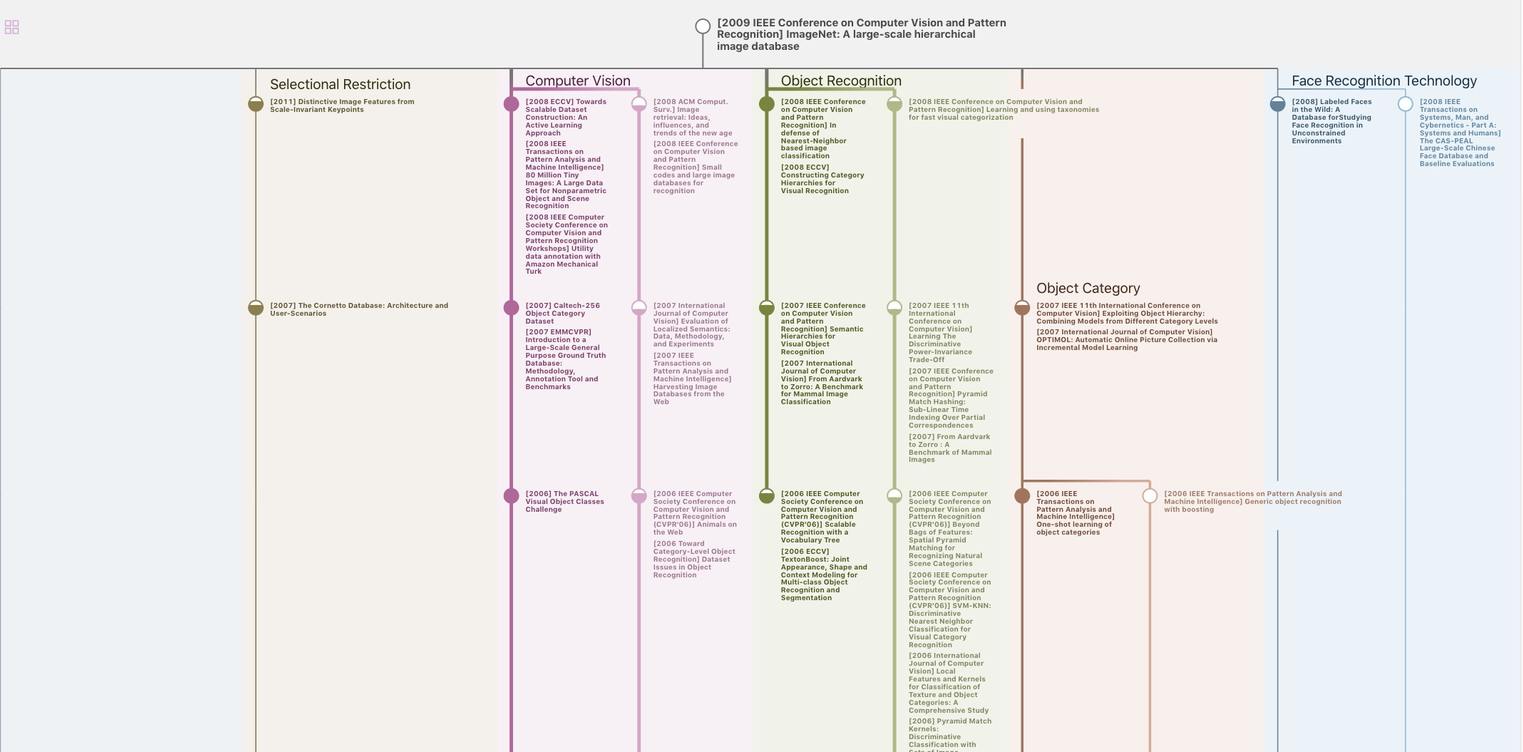
生成溯源树,研究论文发展脉络
Chat Paper
正在生成论文摘要