Trading Strategies Optimization by Genetic Algorithm under the Directional Changes Paradigm
2022 IEEE CONGRESS ON EVOLUTIONARY COMPUTATION (CEC)(2022)
摘要
The subject of financial forecasting has been re-searched for decades, and the driver behind its measured data has been fuelled by the selection of physical time series, which summarize data using fixed time intervals. For instance, time-series for daily stock data would be profiled at 252 points in one year. However, this episodic style neglects the important events, or price changes that occur between two intervals. Thus, we use Directional Changes (DC) as an event-based series, which is an alternative way to record price movements. In DC, unlike time-series methods, time intervals are constituted by price changes. The unique feature that decides the price change to be considered as a significant is called a threshold θ. The objective of our paper is to create DC-based trading strategies, and then optimize them using a Genetic Algorithm (GA). To construct such strategies, we use DC-based indicators and scaling laws that have been empirically identified under DC summaries. We first propose four novel DC-based trading strategies and then combine them with existing DC-based strategies and finally optimize them via the GA. We conduct trading experiments over 44 stocks. Results show that the GA-optimized strategies are able to generate new and profitable trading strategies, significantly outperforming the individual DC-based strategies, as well as a buy and sell benchmark.
更多查看译文
关键词
directional changes, trading strategies, genetic algorithm, financial forecasting, machine learning
AI 理解论文
溯源树
样例
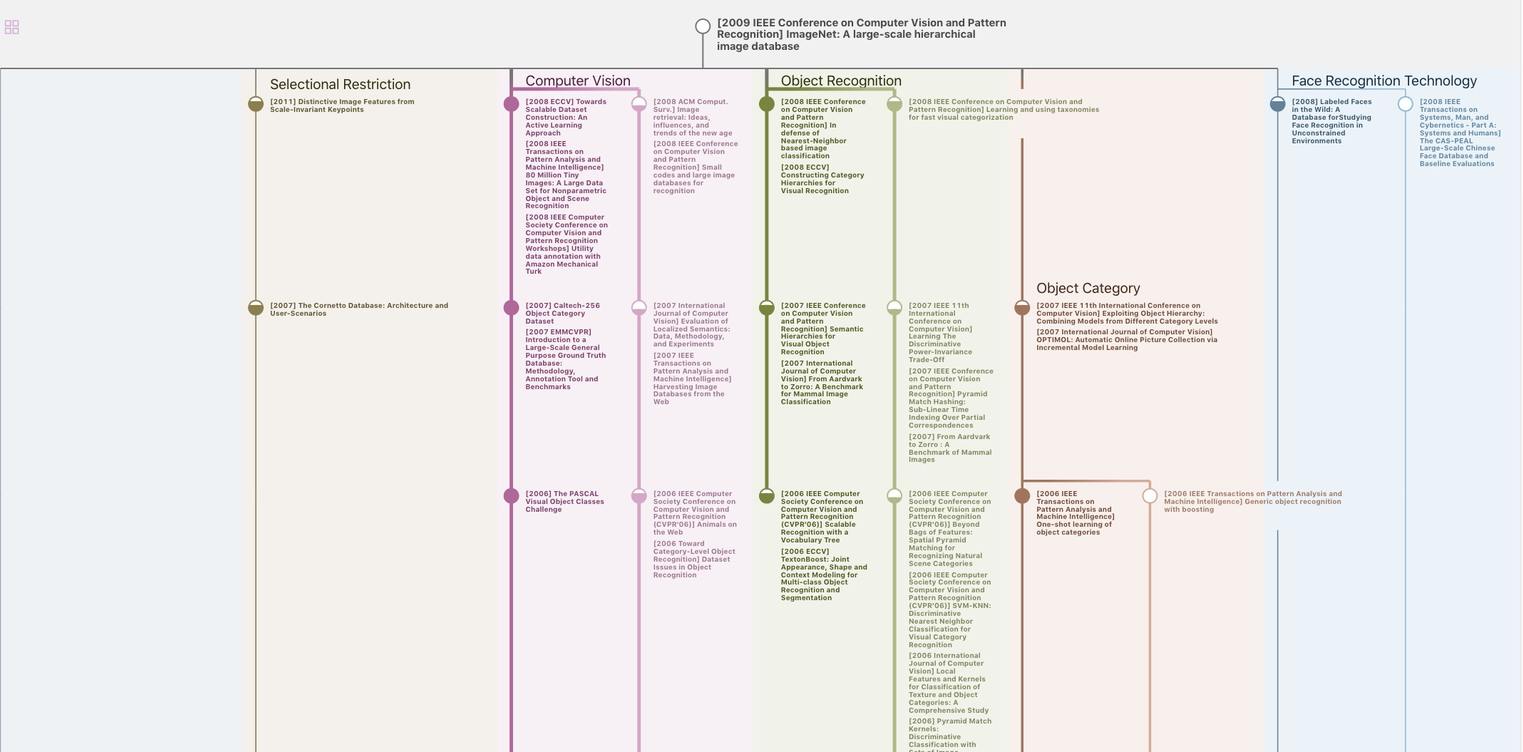
生成溯源树,研究论文发展脉络
Chat Paper
正在生成论文摘要