A multitasking surrogate-assisted differential evolution method for solving bi-level optimization problems
2022 IEEE CONGRESS ON EVOLUTIONARY COMPUTATION (CEC)(2022)
摘要
Bi-level programming (BLP) is a hierarchical decision-making problem in which part of the constraints is determined by solving other optimization problems. Classic optimization techniques cannot be applied directly, while standard metaheuristics often demand high computational costs. The transfer optimization paradigm uses the experience acquired when solving one optimization problem to speed up a distinct but related task. In particular, the multitasking technique addresses two or more optimization tasks simultaneously to explore similarities and improve convergence. BLPs can benefit from multitasking as many (potentially similar) lower-level problems must be solved. Recently, several studies used surrogate methods to save expensive upper-level function evaluations in BLPs. This work proposes an algorithm based on Differential Evolution supported by transfer optimization and surrogate models to solve BLPs more efficiently. Experiments show a reduction of up to 86% regarding the number of function evaluations of the upper-level problem while achieving similar or superior accuracy when compared to state-of-the-art solvers.
更多查看译文
关键词
differential evolution method,optimization,surrogate-assisted,bi-level
AI 理解论文
溯源树
样例
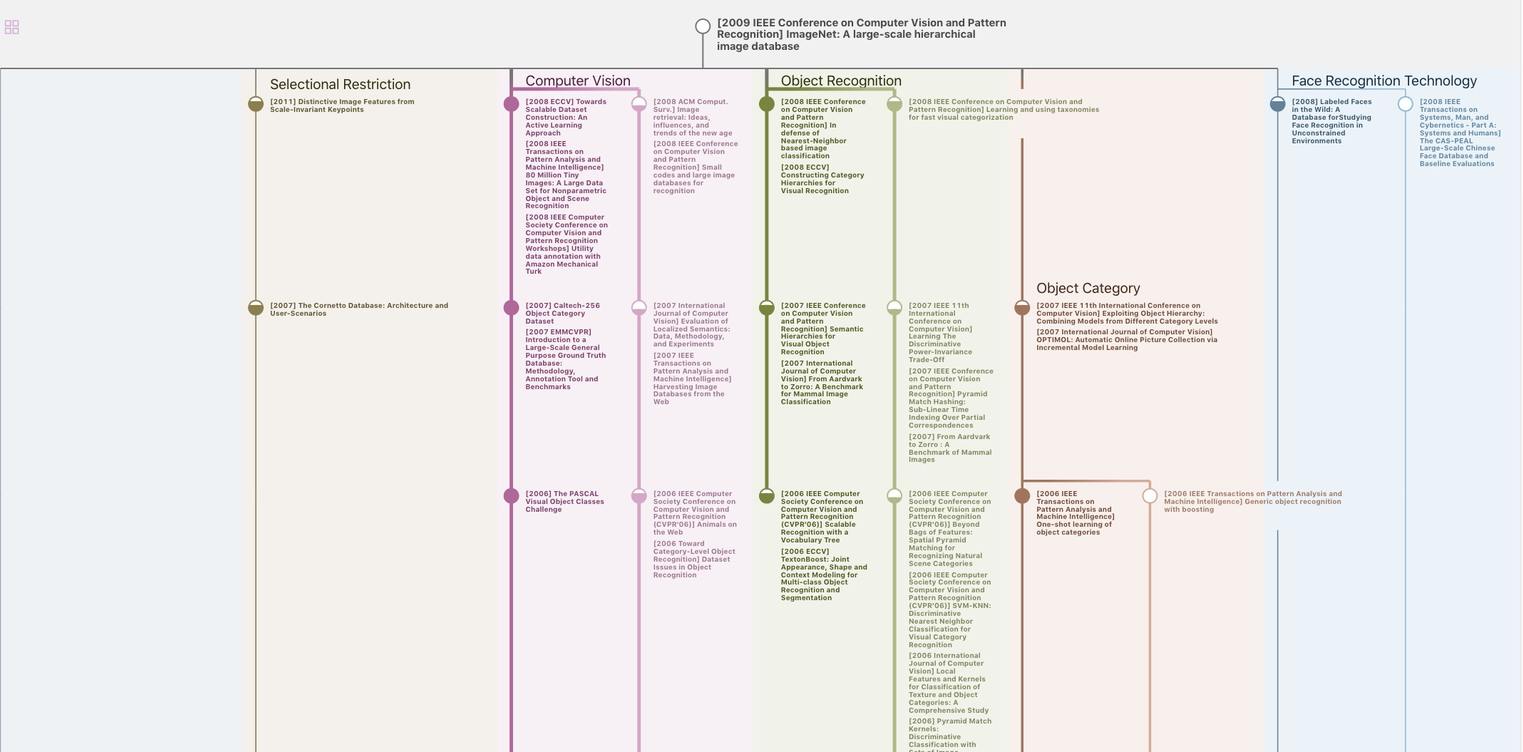
生成溯源树,研究论文发展脉络
Chat Paper
正在生成论文摘要