A 200M-Query-Vector/s Computing-in-RRAM ADC-less k-Nearest-Neighbor Accelerator with Time-Domain Winner-Takes-All Circuits
2022 IEEE INTERNATIONAL CONFERENCE ON ARTIFICIAL INTELLIGENCE CIRCUITS AND SYSTEMS (AICAS 2022): INTELLIGENT TECHNOLOGY IN THE POST-PANDEMIC ERA(2022)
摘要
The
$k$
-nearest neighbor(
$k$
NN) is widely used for pattern matching, data mining, and object recognition [1]. However, the previous computing-in-memory accelerator for the
$k$
NN algorithm heavily relies on analog-digital converter(ADC) circuits leading to huge area and power consumption. This paper proposed a computing-in-RRAM ADC-less k-nearest-neighbor accelerator with time-domain winner-takes-all circuits. The proposed accelerator features a time-domain winner-takes-all circuit with high PVT-variation intolerance and a scalable binary tree structure. Moreover, we improve the performance of voltage control lines circuits with fewer delay stages through the codesign of the computing-in-RRAM module and winner-takes-all circuit. The designed and simulated
$k$
NN accelerator performs up to 200 million query vectors per second while consuming 0.75 mW, demonstrating> 24.5 × energy performance improvement over prior works.
更多查看译文
关键词
computing-in-RRAM ADC-less,k-nearest-neighbor accelerator,pattern matching,data mining,computing-in-memory accelerator,time-domain winner-takes-all circuit,high PVT-variation intolerance,voltage control lines circuits,computing-in-RRAM module,query vectors,simulated k-NN accelerator
AI 理解论文
溯源树
样例
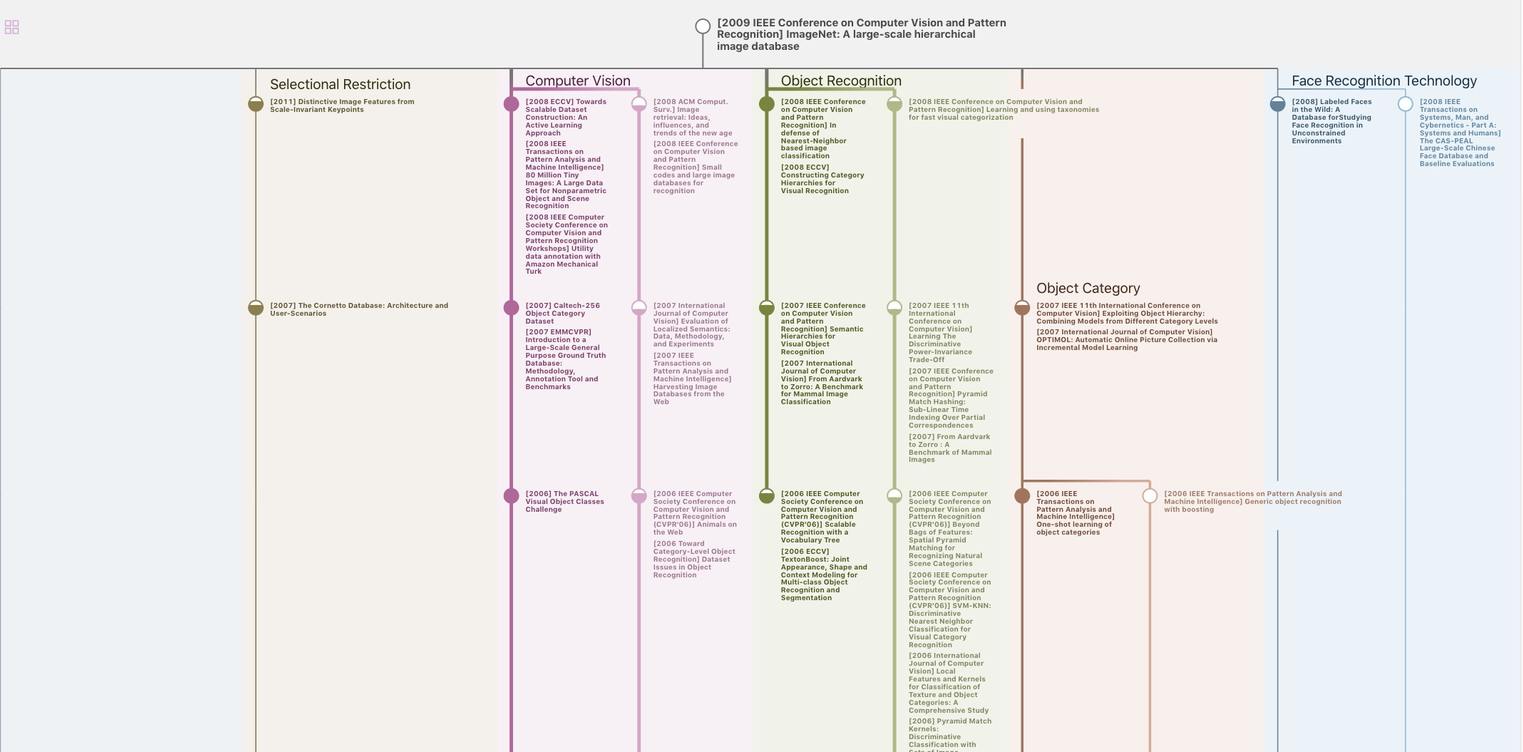
生成溯源树,研究论文发展脉络
Chat Paper
正在生成论文摘要