A Behavior-Level Simulation Framework for RRAM-Based Deep Learning Accelerators with Flexible Architecture Configurations
2022 IEEE 4th International Conference on Artificial Intelligence Circuits and Systems (AICAS)(2022)
摘要
It is recognized that RRAM-based deep learning accelerators provide a promising solution to boost the energy efficiency of neural network algorithms. However, to precisely evaluate the model performance at the early design stage, there is a demand to integrate RRAM's physical properties, such as the lognormal distribution of resistance state, leakage current, and sneak path, to the neural network design framework (such as PyTorch). In this paper, we propose a behavior-level simulator, which considers RRAM's physical properties, for the development of RRAM-based deep learning accelerators. Especially, our simulator has the flexibility to allow users to configure their own hardware architectures. As a result, an accurate behavior-level hardware architecture exploration can be made at the early design stage. Benchmark data consistently show that the proposed approach works well in practice.
更多查看译文
关键词
Computing-in-memories,DNN accelerators,Non-linear effects,RRAM arrays,Simulator
AI 理解论文
溯源树
样例
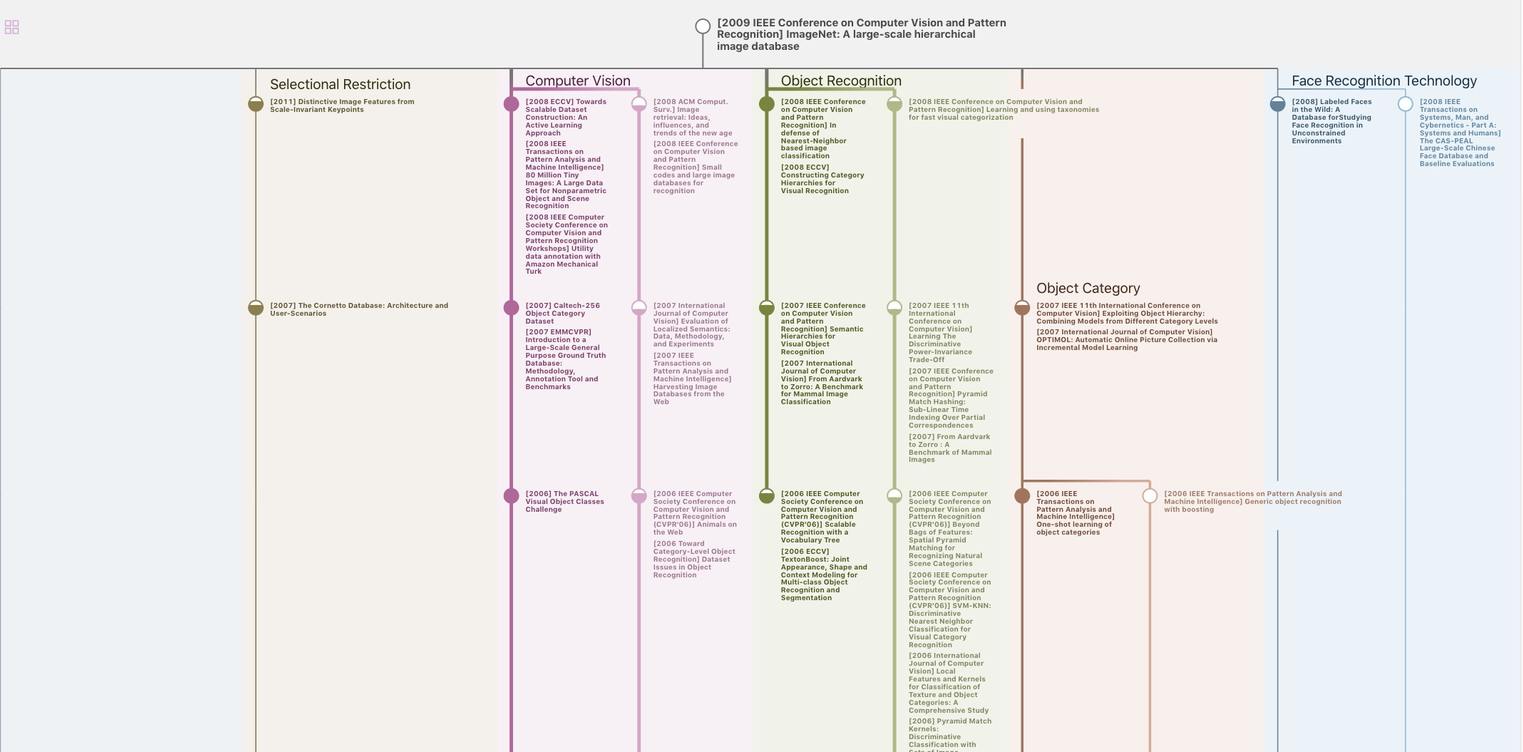
生成溯源树,研究论文发展脉络
Chat Paper
正在生成论文摘要