Weighted Decoupling: An Effective Image Resizing Method for Binarized Neural Network
2022 IEEE 4th International Conference on Artificial Intelligence Circuits and Systems (AICAS)(2022)
摘要
Many neuromorphic hardware uses a micro controller unit that has low computing power and small memory size. To process deep neural network applications on hardware-restricted conditions and to alleviate computational latency, binarized neural networks have been developed. Moreover, we can reduce required multiply-accumulate operation by shrinking resolution of input images. In this paper, we compare various image resizing method and propose weighted decoupling, a new resizing method for the best accuracy of ResNetB18 model, an additionally binarized version of ResNetE18. A ResNetB18 model with proposed resizing method shows the better accuracy performance than that of a same model trained with 12.25× large images.
更多查看译文
关键词
Neuromorphic Hardware,ResNet,ResNetE18,ResNetB18,Binarized Neural Network,Image resizing
AI 理解论文
溯源树
样例
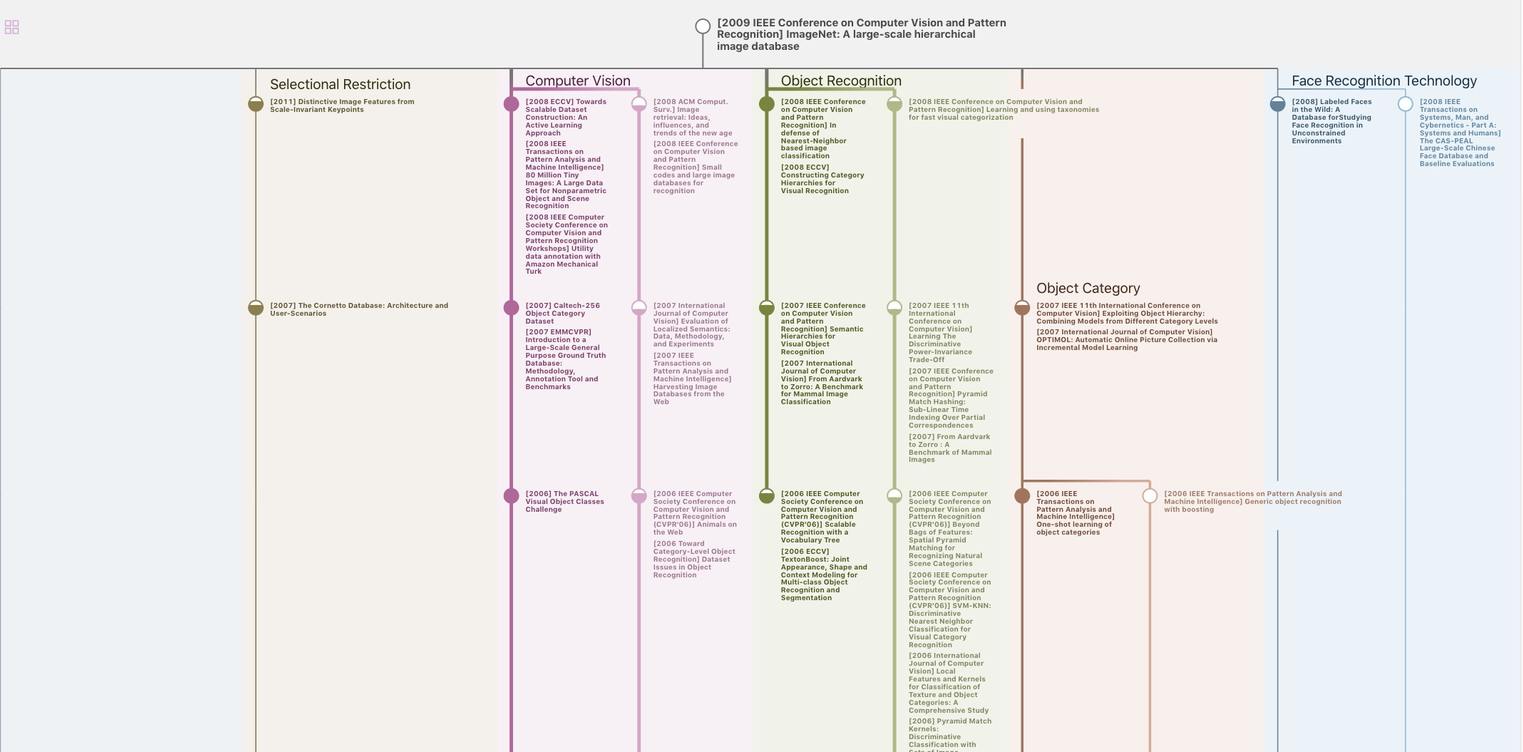
生成溯源树,研究论文发展脉络
Chat Paper
正在生成论文摘要