Transformer Based Multi-task Deep Learning with Intravoxel Incoherent Motion Model Fitting for Microvascular Invasion Prediction of Hepatocellular Carcinoma
MEDICAL IMAGE COMPUTING AND COMPUTER ASSISTED INTERVENTION, MICCAI 2022, PT VII(2022)
摘要
Prediction of microvascular invasion (MVI) in hepatocellular carcinoma (HCC) has important clinical value for treatment decisions and prognosis. Diffusion-weighted imaging (DWI) intravoxel incoherent motion (IVIM) models have been used to predict MVI in HCC. However, the parameter fitting of the IVIM model based on the typical nonlinear least squares method has a large amount of computation, and its accuracy is disturbed by noise. In addition, the performance of characterizing tumor characteristics based on the feature of IVIM parameter values is limited. In order to overcome the above difficulties, we proposed a novel multi-task deep learning network based on transformer to simultaneously conduct IVIM parameter model fitting and MVI prediction. Specifically, we utilize the transformer's powerful long-distance feature modeling ability to encode deep features of different tasks, and then generalize self-attention to cross-attention to match features that are beneficial to each task. In addition, inspired by the work of Compact Convolutional Transformer (CCT), we design the multi-task learning network based on CCT to enable the transformer to work in the small dataset of medical images. Experimental results of clinical HCC with IVIM data show that the proposed transformer based multi-task learning method is better than the current multi-task learning methods based on attention. Moreover, the performance of MVI prediction and IVIM model fitting based on multitask learning is better than those of single-task learning methods. Finally, IVIM model fitting facilitates the performance of IVIM to characterize MVI, providing an effective tool for clinical tumor characterization.
更多查看译文
关键词
Transformer, Multi-task learning, Attention mechanism
AI 理解论文
溯源树
样例
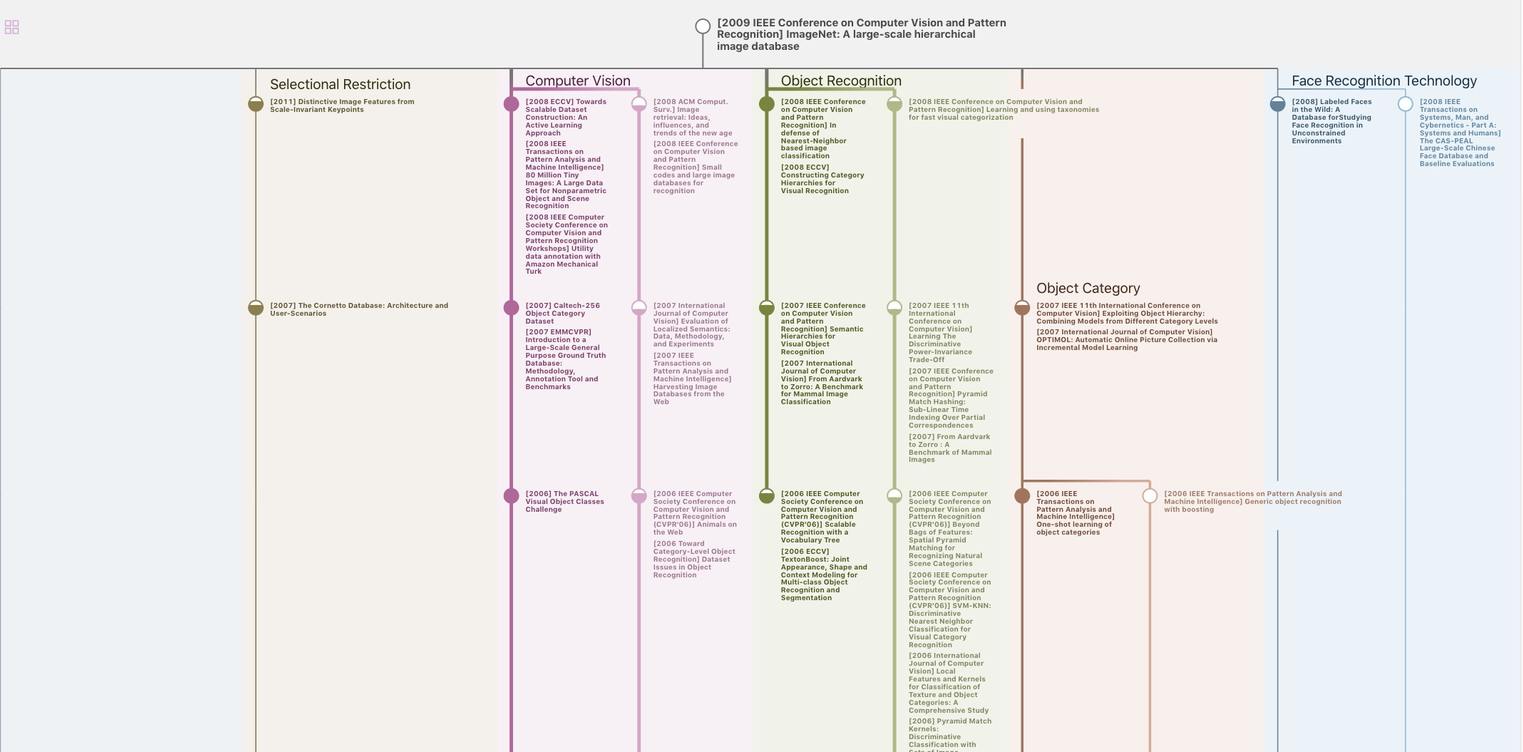
生成溯源树,研究论文发展脉络
Chat Paper
正在生成论文摘要