A more informed clustering algorithm through the aggregation of linkage methods
2022 IEEE INTERNATIONAL CONFERENCE ON FUZZY SYSTEMS (FUZZ-IEEE)(2022)
摘要
Agglomerative hierarchical clustering algorithms for finding groups in unlabelled data achieve different clusters of objects depending on how the similarity between two clusters is measured. In this context, the linkage method refers to the process to decide if two clusters are merged. However, it is not possible to establish in advance the linkage method that provides the best grouping of the data. In the classic hierarchical clustering algorithm, the two most similar clusters according to one linkage method are merged together into a single cluster. In this work, we propose a hierarchical clustering algorithm that aggregates in each step the criteria of the single, complete and average linkage methods in order to determine the two most similar clusters to merge. In each step, each linkage method gives a ranking of pairs of clusters, where the pairs are ordered from most to least similar. The rankings given by each linkage method are aggregated using ranking rules to achieve a consensus of the different criteria about which one is the pair to be merged into a single cluster. Results obtained from the validation of the new algorithm with the Rand Index metric in data sets with different characteristics show how the proposed algorithm is useful for reducing the impact of the linkage method chosen in the final clusters obtained.
更多查看译文
关键词
Hierarchical clustering, Information fusion, Ranking aggregation, Scoring ranking rules
AI 理解论文
溯源树
样例
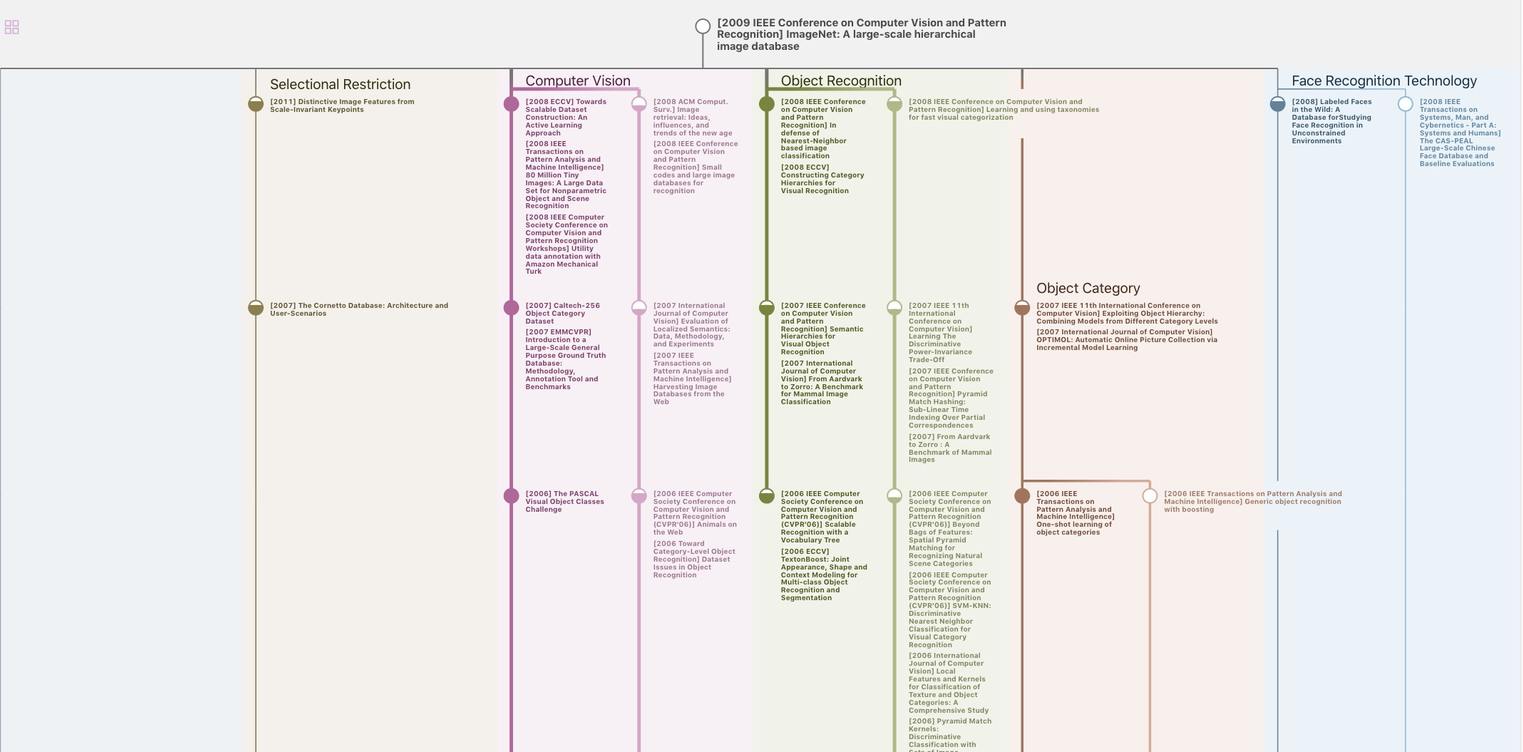
生成溯源树,研究论文发展脉络
Chat Paper
正在生成论文摘要